A Comprehensive Feature Aggregation Network for Sleep Apnea Detection using Respiratory Signals.
2023 IEEE International Conference on Bioinformatics and Biomedicine (BIBM)(2023)
摘要
Sleep apnea is a prevalent sleep disorder that poses a significant public health concern. Polysomnography, the gold standard for detecting sleep apnea, is costly and time-consuming, making widespread adoption challenging. To address this issue, in this paper, we propose a novel Comprehensive Feature Aggregation Network(CFAN) model comprising an embedding layer, an attention module, and a temporal module. The proposed CFAN leverages one of the respiratory signals as its input. Grouped dilated convolutional neural networks with various dilation rates, multi-head self-attention mechanism, and bidirectional Gated Recurrent Unit effectively integrate global and local spatial-temporal information from the input signal, enabling the characterization of intricate internal details and facilitate the detection of sleep apnea syndrome. Experimental results unequivocally demonstrate the superior performance of our proposed model, surpassing both benchmark models and the current state-of-the-art in terms of its comprehensive ability and robustness. Furthermore, ablation experiments confirm the indispensability of each component within the model. Our proposed method offers a convenient and innovative approach to automatically detect sleep apnea syndrome using respiratory signals, presenting a promising avenue for further application.
更多查看译文
关键词
Deep learning,Dilated convolution,Multi-head self-attention,Respiratory signal,Sleep apnea
AI 理解论文
溯源树
样例
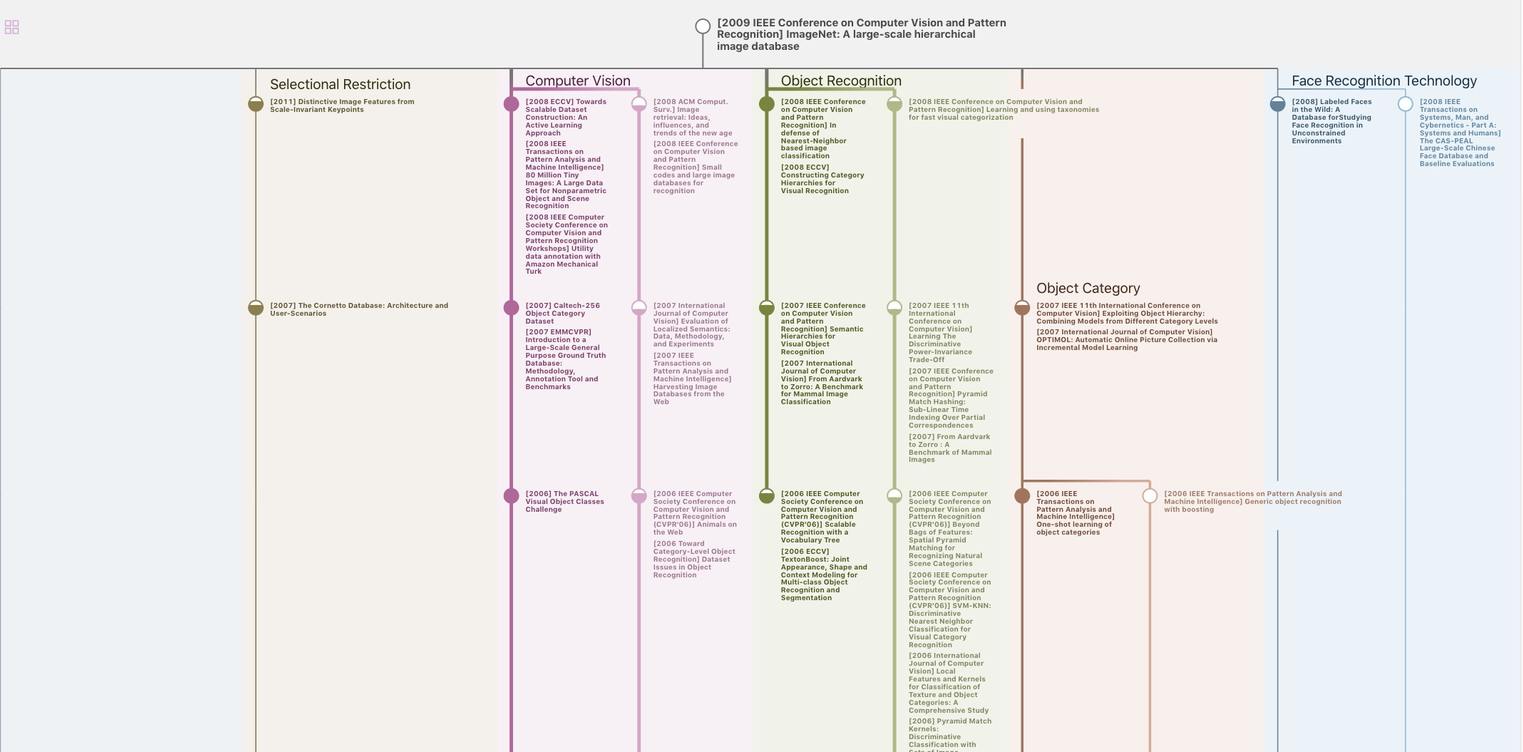
生成溯源树,研究论文发展脉络
Chat Paper
正在生成论文摘要