Graph-Based Alzheimer's Disease Diagnosis with Contrastive Learning and Graph Transformer.
2023 IEEE International Conference on Bioinformatics and Biomedicine (BIBM)(2023)
摘要
Structural magnetic resonance imaging (sMRI) plays an important role in Alzheimer’s disease (AD) diagnosis. AD-related brain atrophy exhibits subtle local structural changes. Therefore, the key to AD diagnosis lies in pathological region identification and discriminative feature learning. However, existing methods often overlook the spatial and feature relationships between brain regions. To address this, we propose a graph learning framework based on contrastive learning and graph transformer for AD diagnosis using sMRI. First, we use a supervised contrastive learning strategy to learn discriminative brain representations from sMRI data. The features of the regions of interest (ROIs) are computed using a brain template to represent the graph nodes. Then, we build a multi-level connected brain network that incorporates spatial and feature information. Finally, a graph transformer network is introduced to gradually aggregate graph information for AD diagnosis. Our method achieves good performance in AD diagnosis using sMRI data from the ADNI dataset. Additionally, important brain regions such as the hippocampus, amygdala, and insula are identified as highly associated with Alzheimer’s disease.
更多查看译文
关键词
Alzheimer’s disease,contrastive learning,graph transformer,medical image analysis
AI 理解论文
溯源树
样例
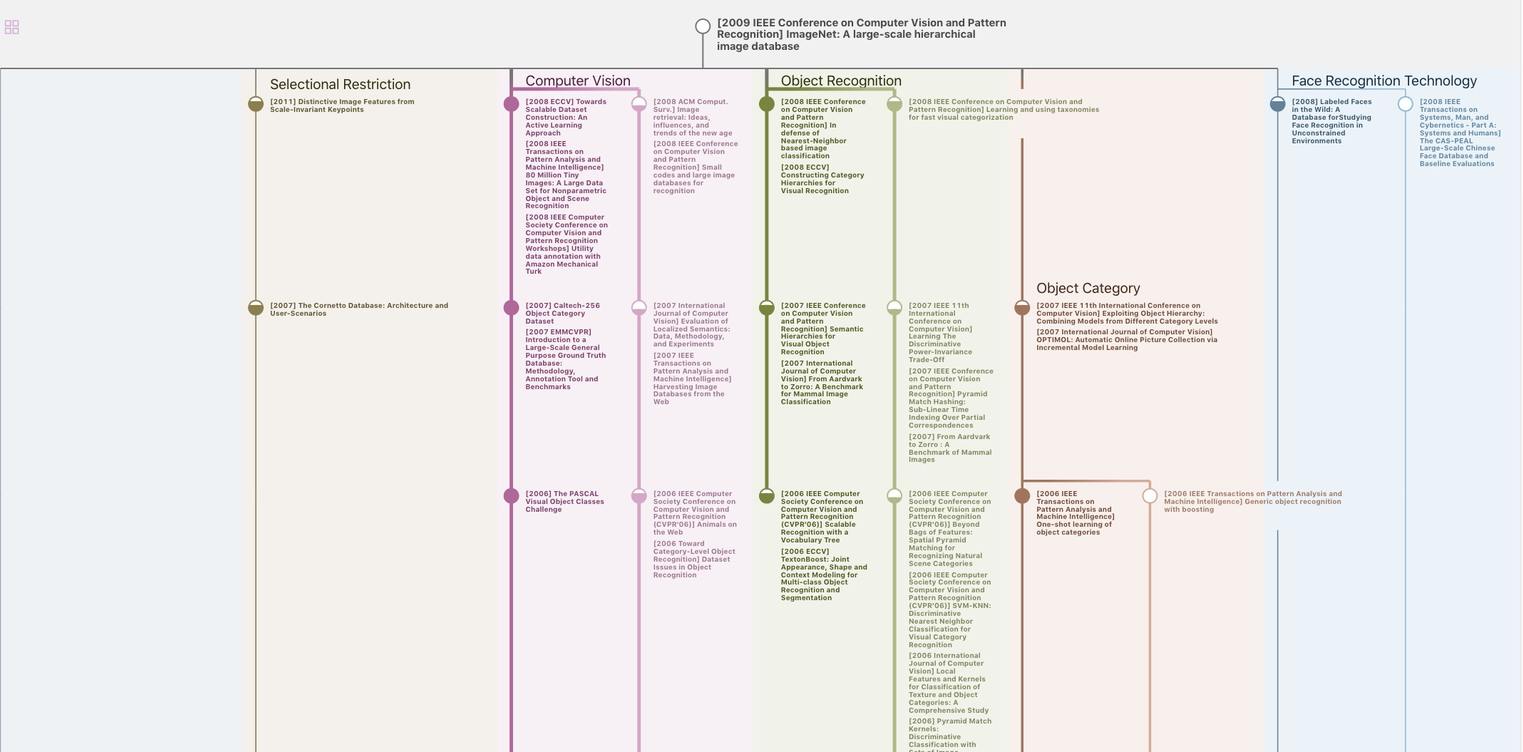
生成溯源树,研究论文发展脉络
Chat Paper
正在生成论文摘要