Generative Positive-Unlabeled Classification for Hunting Small Open Reading Frames.
IEEE International Conference on Bioinformatics and Biomedicine(2023)
摘要
The annotation of Open Reading Frames (ORFs) is a crucial step in gene annotation, as it precisely delineates the specific regions of expressed genes. However, small Open Reading Frames (smORFs), in comparison to ORFs, are shorter in length, exhibit lower expression abundance, and are more challenging to predict. Particularly in the presence of noise in prokaryotic data and limited availability of positive sample data, the difficulty of prediction is amplified. Therefore, it is necessary to study smORF prediction methods. However, current machine learning models use limited data for modeling and overlook the existence of undiscovered positive samples within the negative samples. Additionally, they do not incorporate prior knowledge that can be calibrated to enhance the 3-nt periodicity. This work utilizes a multimodal VAE for data dimensionality reduction and employs a GAN to generate latent vectors for data augmentation. It incorporates PU learning to leverage unknown samples and combines Riboseq data from experiments with and without antibiotic treatment. Additionally, an adversarial training mechanism is employed to enhance the model’s robustness.
更多查看译文
关键词
Gene Annotation,Class Imbalance,Data Generation,Positive-Unlabeled Learning
AI 理解论文
溯源树
样例
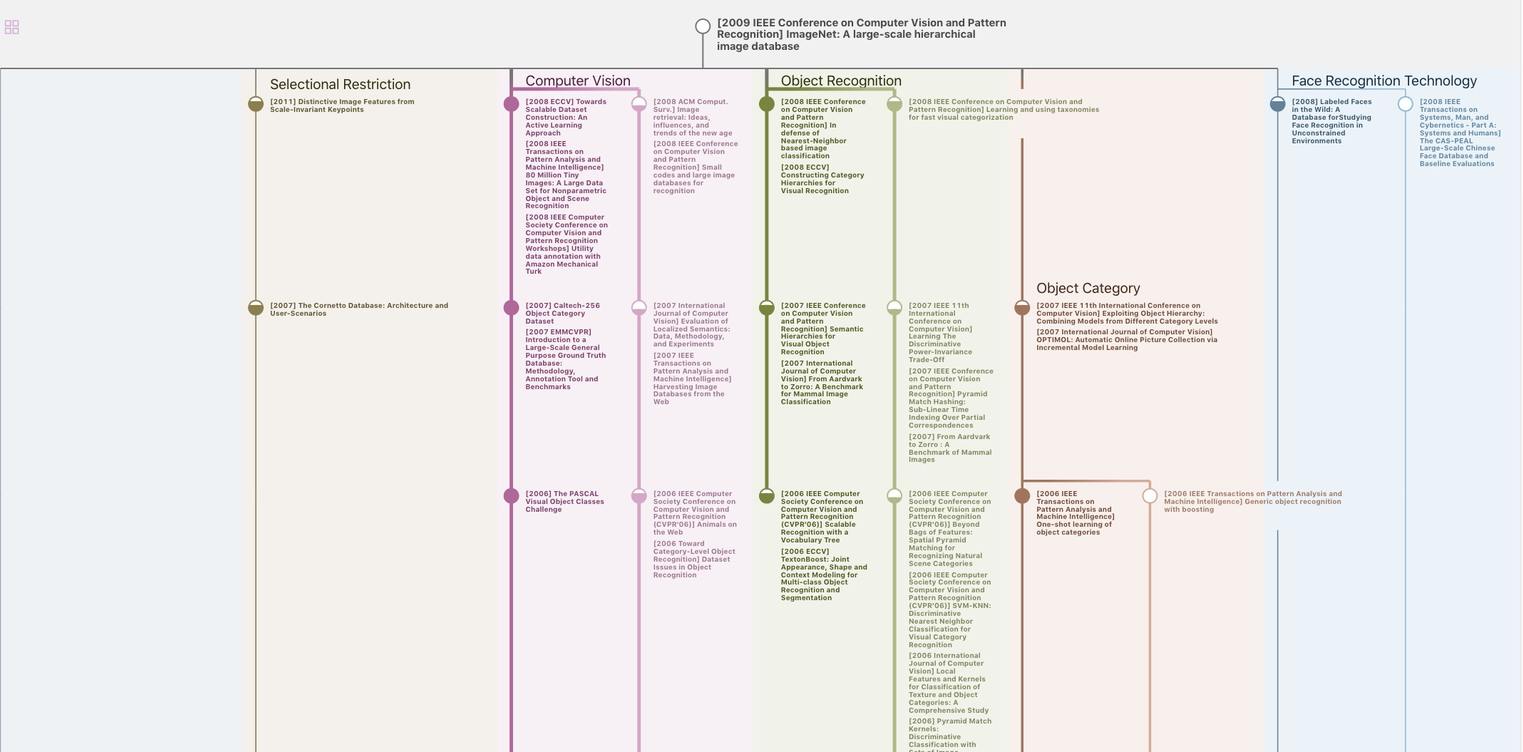
生成溯源树,研究论文发展脉络
Chat Paper
正在生成论文摘要