Physics-Model-Regulated Deep Reinforcement Learning Towards Safety & Stability Guarantees
2023 62ND IEEE CONFERENCE ON DECISION AND CONTROL, CDC(2023)
摘要
Deep reinforcement learning (DRL) has demonstrated impressive success in solving complex control tasks by synthesizing control policies from data. However, the safety and stability of applying DRL to safety-critical systems remain a primary concern and challenging problem. To address the problem, we propose the Phy-DRL: a novel physics-modelregulated deep reinforcement learning framework. The Phy-DRL is novel in two architectural designs: a physics-model-regulated reward and residual control, which integrates physics-modelbased control and data-driven control. The concurrent designs enable the Phy-DRL the mathematically provable safety and stability guarantees. Finally, the effectiveness of the Phy-DRL is validated by an inverted pendulum system. Additionally, the experimental results demonstrate that the Phy-DRL features remarkably accelerated training and enlarged reward.
更多查看译文
AI 理解论文
溯源树
样例
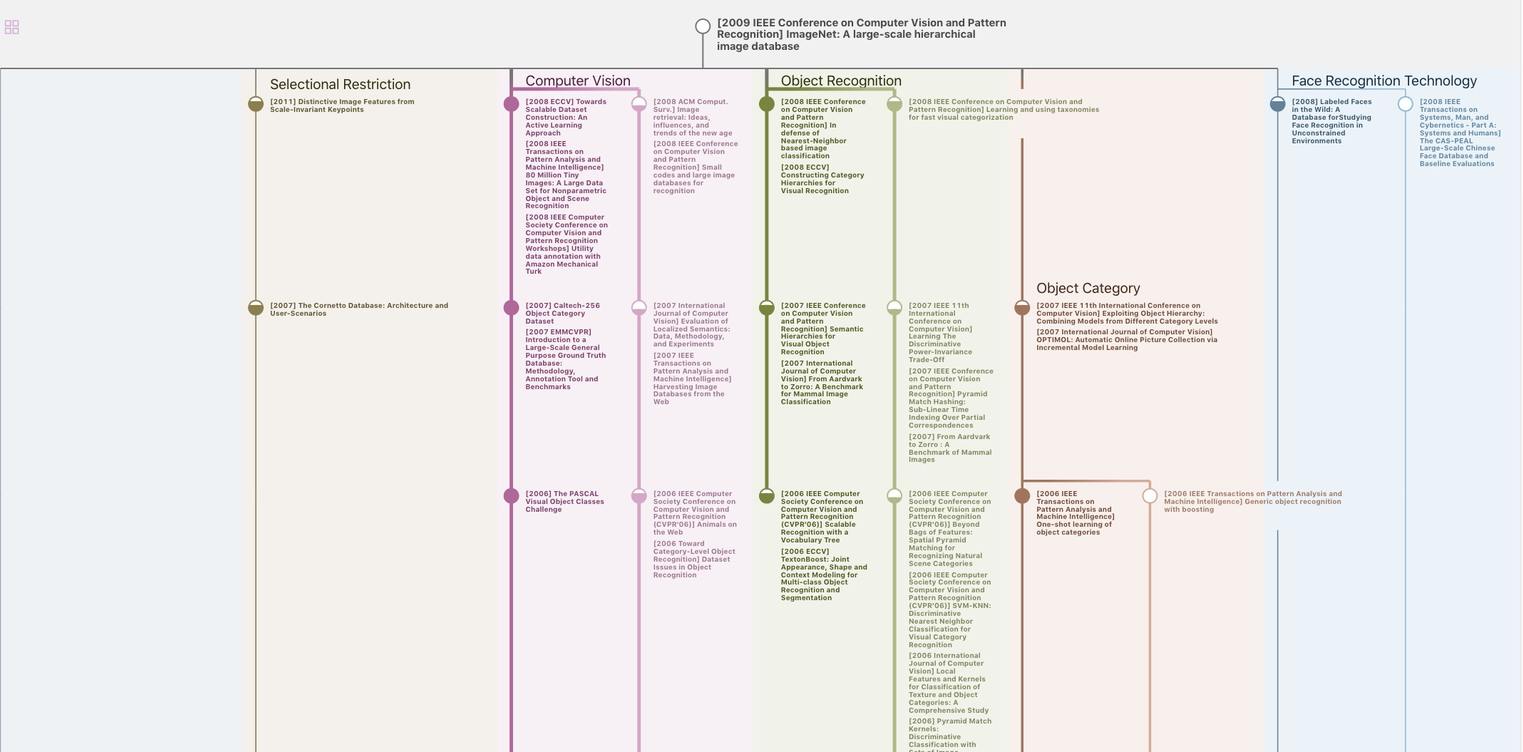
生成溯源树,研究论文发展脉络
Chat Paper
正在生成论文摘要