Deep Residual Fourier and Self-Attention for Arbitrary Scale MRI Super-Resolution.
2023 IEEE International Conference on Bioinformatics and Biomedicine (BIBM)(2023)
摘要
The excellent inherent contrast between biological tissues afforded by MR imaging is one of the foremost characteristics of this technique, but it depends on the scan time and hardware devices. Recent studies have shown significant progress in deep learning-based single-image super-resolution (SISR) algorithms based on MR images. Many researchers have employed implicit functions for super-resolution tasks, achieving arbitrary resolution upsampling. Nevertheless, challenges like loss of generated image texture and weak high-frequency features persist. We propose an arbitrary multiple super-resolution model based on deep residual Fourier transform and a selfattention mechanism to tackle these issues. We first use the deep Fourier residual block to construct the high and low-frequency difference of the image, compensating for the spectral bias of the multiple perceptron (MLP) network. Building upon the substantial similarity in medical image tissue structures, we add a vertical and horizontal self-attention mechanism to capture the internal correlation of features in the vertical and horizontal directions. Finally, we learn a continuous functional representation to execute super-resolution tasks at any scale. Experiment results show the effectiveness of our method on the T2 sequence of public dataset SIMON and T1, T2, and Proton Density (PD) weighted scan sequences of public dataset IXI. We conduct qualitative and quantitative comparisons of the three contrasts, illustrating the superiority of our approach.
更多查看译文
关键词
single-image super-resolution,the deep Fourier residual block,self-attention
AI 理解论文
溯源树
样例
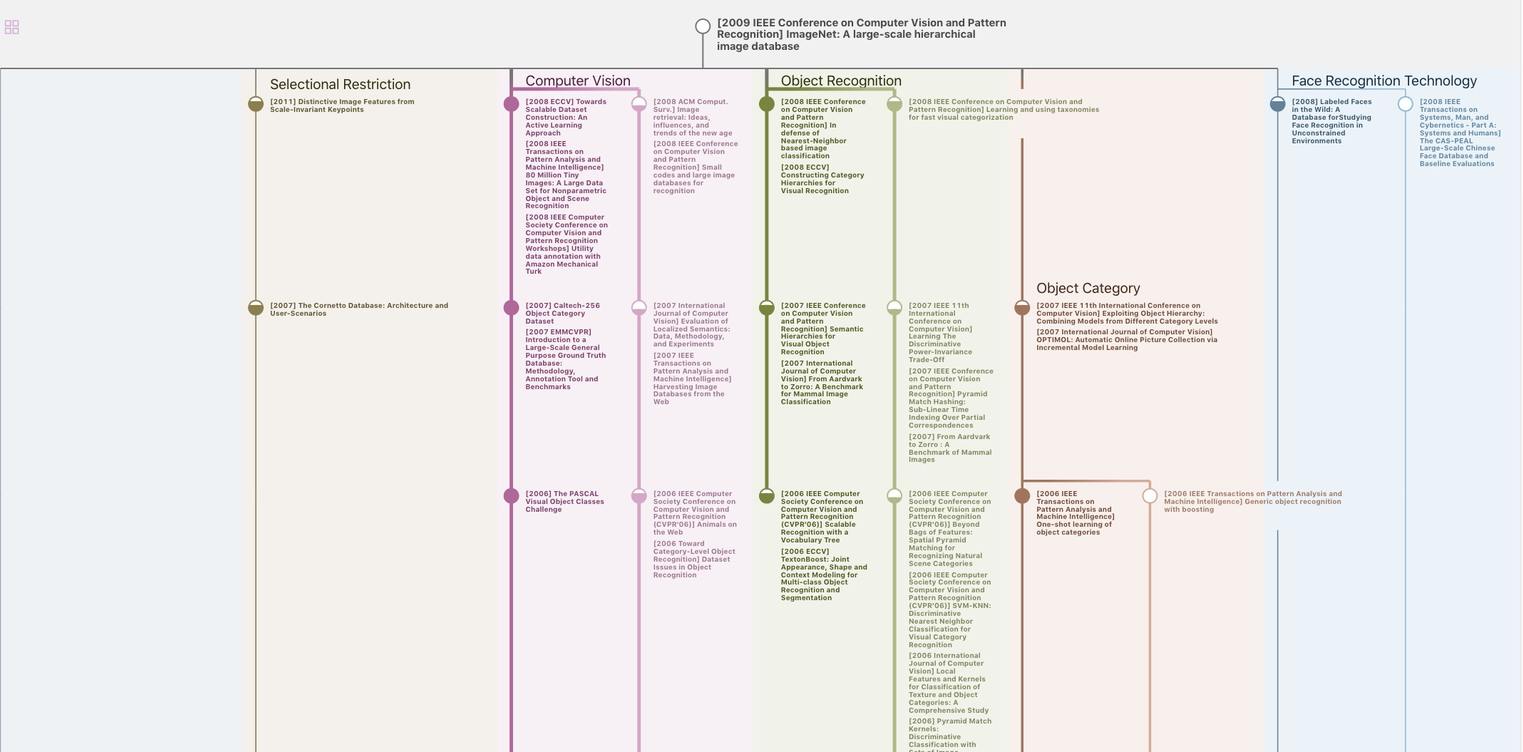
生成溯源树,研究论文发展脉络
Chat Paper
正在生成论文摘要