SLPFA: Protein Structure-Label Embedding Attention Network for Protein Function Annotation.
IEEE International Conference on Bioinformatics and Biomedicine(2023)
摘要
Gene Ontology (GO) is a framework that utilizes a series of GO terms in a Directed Acyclic Graph (DAG) to describe protein functions. Proteins are typically annotated with several or dozens of GO terms. However, existing methods often struggle to simultaneously annotate multiple relevant GO terms with hierarchical dependencies to proteins, as they solely rely on protein sequences or structures. To better utilize the hierarchical information of GO terms and improve protein function annotation performance, we propose the Protein Structure-Label Embedding Attention Network for Protein Function Annotation (SLPFA). SLPFA embeds proteins and GO terms into a joint latent space using attention mechanisms to bridge the semantic gap between them. Specifically, we employ a soft-mask GNN to learn the topological structure of proteins, allowing simultaneous focus on key nodes while remaining invariant to irrelevant parts. Additionally, we encode the ancestral information for each GO term in its embedding and utilize a learnable matrix to capture the hierarchical dependencies. Finally, SLPFA employs protein structure-label embedding attention to project the protein structure and label embedding together into a joint latent space. This enables the model to learn the high-level semantics of proteins and hierarchical GO terms, resulting in a reduced semantic gap between proteins and their functions. Experimental results demonstrate that SLPFA outperforms state-of-the-art deep learning-based methods on the PDB-cdhit dataset, which yields Fmax of 0.604, 0.478, 0.524 and the AUPRC of 0.630, 0.357, 0.452 for the MF, BP, CC ontology domains, respectively. Furthermore, when the training and testing proteins have less than 15% sequence identity, SLPFA also achieves competitive results in the MF, BP, and CC ontology domains.
更多查看译文
关键词
protein function annotation,soft-mask GNN,learnable matrix,gene ontology,deep learning
AI 理解论文
溯源树
样例
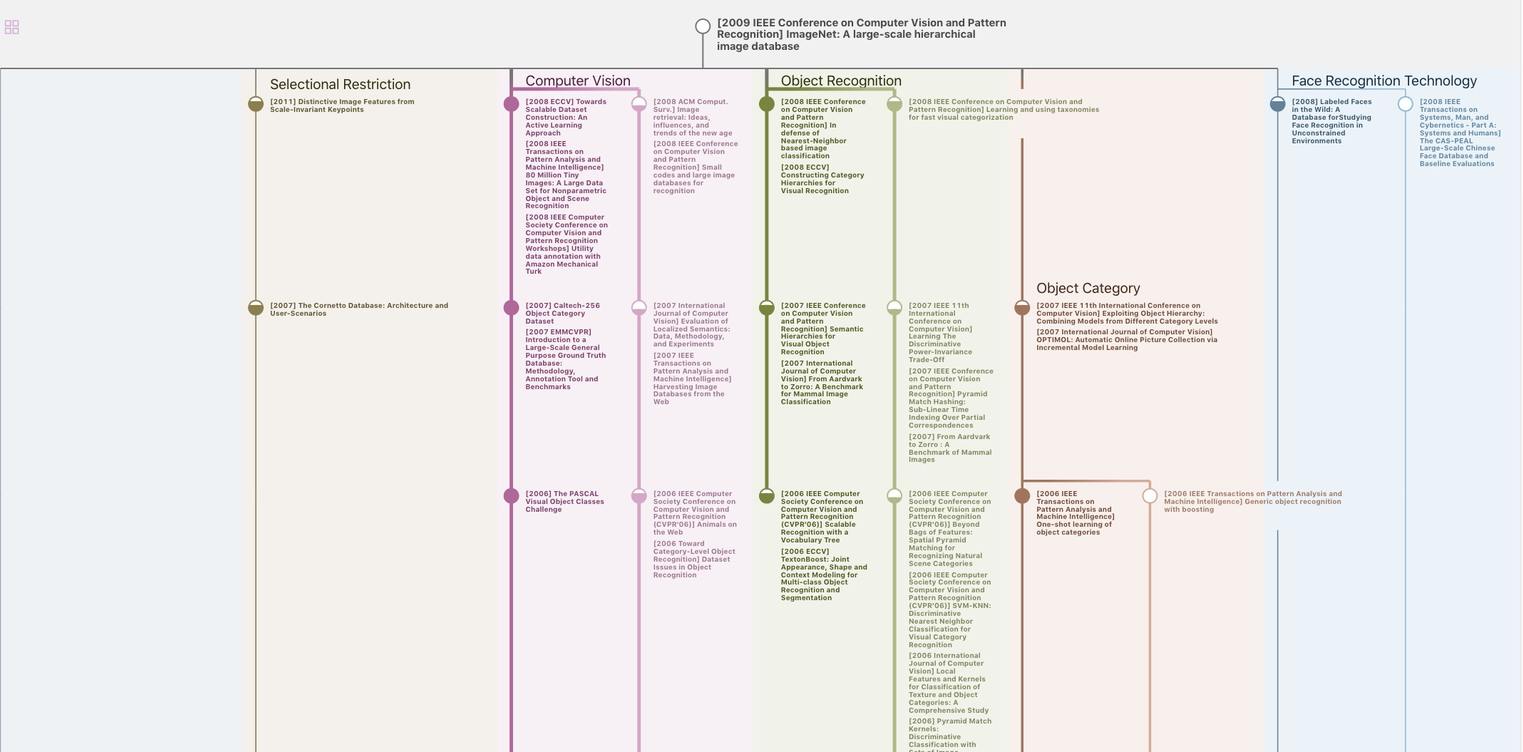
生成溯源树,研究论文发展脉络
Chat Paper
正在生成论文摘要