Distributionally Robust Behavioral Cloning for Robust Imitation Learning
2023 62ND IEEE CONFERENCE ON DECISION AND CONTROL, CDC(2023)
摘要
Robust reinforcement learning (RL) aims to learn a policy that can withstand uncertainties in model parameters, which often arise in practical RL applications due to modeling errors in simulators, variations in real-world system dynamics, and adversarial disturbances. This paper introduces the robust imitation learning (IL) problem in a Markov decision process (MDP) framework where an agent learns to mimic an expert demonstrator that can withstand uncertainties in model parameters without additional online environment interactions. The agent is only provided with a dataset of state-action pairs from the expert on a single (nominal) dynamics, without any information about the true rewards from the environment. Behavioral cloning (BC), a supervised learning method, is a powerful algorithm to address the vanilla IL problem. We propose an algorithm for the robust IL problem that utilizes distributionally robust optimization (DRO) with BC. We call the algorithm DR-BC and show its robust performance against parameter uncertainties both in theory and in practice. We also demonstrate the empirical performance of our approach to addressing model perturbations on several MuJoCo continuous control tasks.
更多查看译文
关键词
Imitation Learning,Reinforcement Learning,Robust Reinforcement Learning
AI 理解论文
溯源树
样例
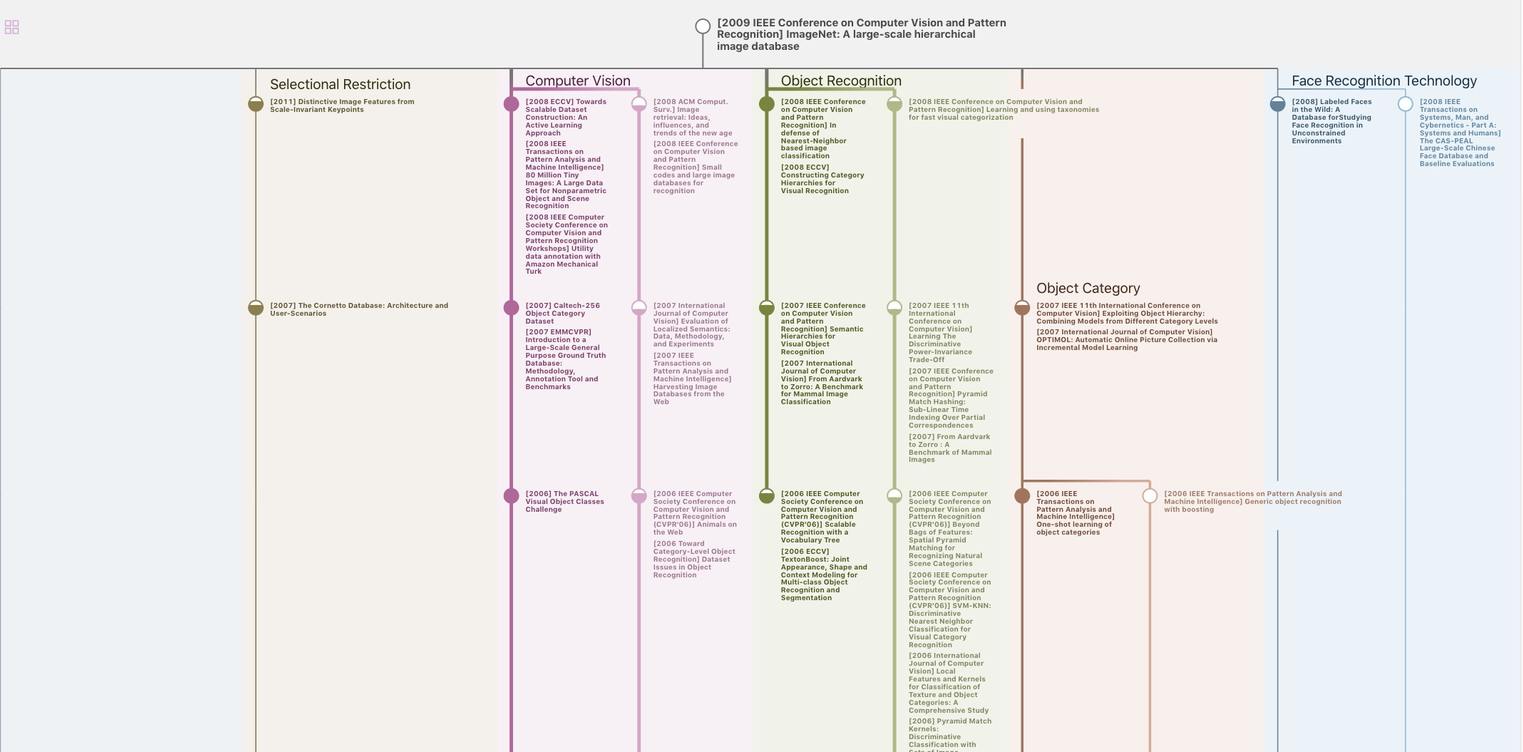
生成溯源树,研究论文发展脉络
Chat Paper
正在生成论文摘要