DUALWISE-MAR: Dual-domain Multi-view Weakly supervised Segmentation Network for CBCT Metal Artifact Reduction.
2023 IEEE International Conference on Bioinformatics and Biomedicine (BIBM)(2023)
摘要
Intraoperative Cone-Beam Computed Tomography (CBCT) provides fast multiplanar cross-sectional imaging and three-dimensional reconstructions in clinical scene. However, high-attenuation metallic implants result in the obstruction of low-energy X-rays and further lead to metal artifacts in the reconstructed CT images. Existing metal artifact reduction approaches do not consider automated fine-grained metallic implants segmentation. Imprecise metallic implants segmentation severely limits the clinical practical applicability of MAR approaches. To address the above challenge, in this study, we present a novel DUAL-domain multi-view Weak supervIsed SEgmentation network for Metal Artifact Reduction (DUALWISE-MAR) to coarse-to-fine segment metallic implants without segmentation ground-truth. First, an Iterative Segmentation Refinement Mechanism is constructed to progressively enhance initial coarse label and guide segmentation network close to the ideal metal mask. Second, a Multi-view Consistent Segmentation Network is constructed for data-consistent segmentation to capture the metal shape relationship between adjacent views in projection-domain. Third, a Segmentation Recalibration Module is proposed to calibrate the inaccurate prediction regions in the imagedomain. Extensive experiments have been conducted on the real clinical CBCT dataset and demonstrate that the proposed DUALWISE-MAR framework achieves excellent performance in metal segmentation compared to the state-of-the-art methods.
更多查看译文
关键词
CBCT metal artifact reduction,weakly supervised learning,dual-domain,metal segmentation
AI 理解论文
溯源树
样例
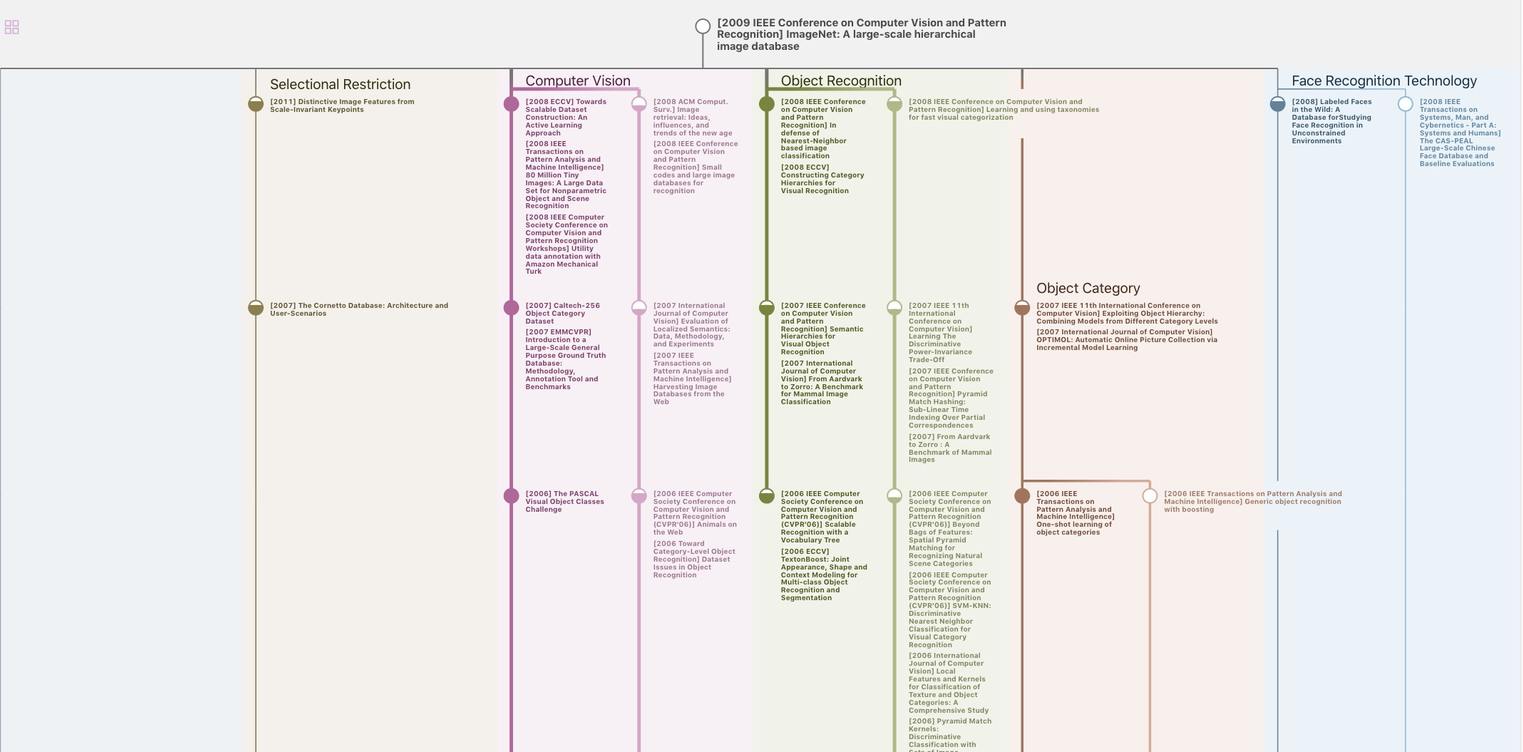
生成溯源树,研究论文发展脉络
Chat Paper
正在生成论文摘要