Multi-Agent Reinforcement Learning for Resource Allocation in Large-Scale Robotic Warehouse Sortation Centers
2023 62ND IEEE CONFERENCE ON DECISION AND CONTROL, CDC(2023)
摘要
Robotic sortation centers use mobile robots to sort packages by their destinations. The destination-to-sort-location (chute) mapping can significantly impact the volume of packages that can be sorted by the sortation floor. In this work, we propose a multi-agent reinforcement learning method to solve large-scale chute mapping problems with hundreds of agents (the destinations). To address the exponential growth of the state-action space, we decompose the joint action-value function as the sum of local action-value functions associated with the individual agents. To incorporate robot congestion effects on the rates at which packages are sorted, we couple the local action-value functions through the states of destinations mapped to nearby chutes on the sortation floor. We show that our proposed framework can solve large chute mapping problems and outperforms static or reactive policies that are commonly used in practice in robotic sortation facilities.
更多查看译文
AI 理解论文
溯源树
样例
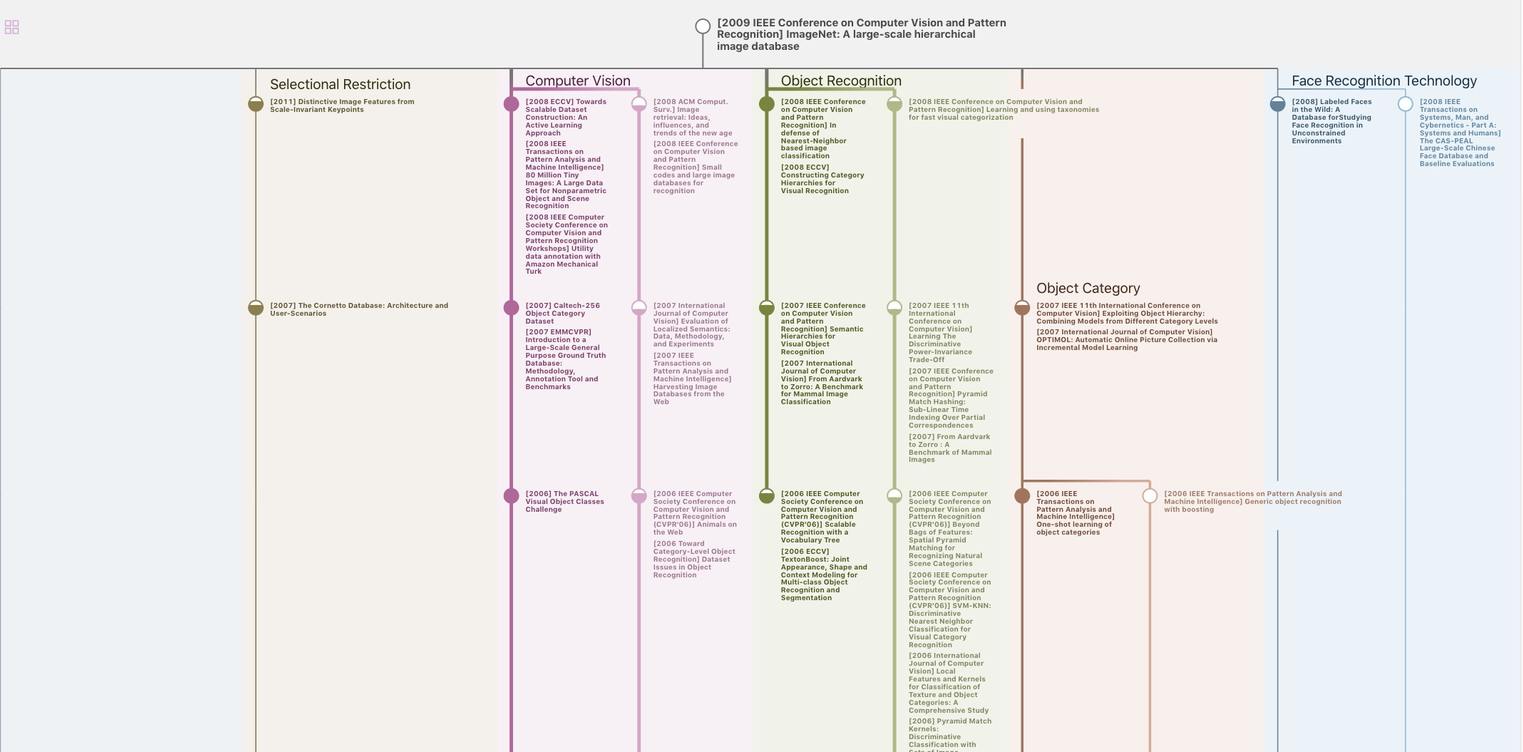
生成溯源树,研究论文发展脉络
Chat Paper
正在生成论文摘要