A Novel 130.1 pJ/Decision Binary Tree Ensemble Classifier for an Energy Efficient Atrial Fibrillation Detecting ECG Processing System in 22 nm FDSOI.
2023 IEEE Biomedical Circuits and Systems Conference (BioCAS)(2023)
摘要
This paper presents a new classifier architecture for decision tree ensembles (DTEs) based on lookup-tables. The classifier is integrated in an electrocardiogram processing system that detects atrial fibrillation (AF). It targets energy efficiency and achieves best in class performance for AF-detecting systems by a margin of one to two orders of magnitude at 275.9 pJ/heartbeat. The classifier itself only requires 130.1 pJ/decision or 0.93 pJ/node per decision at up to 5 Mdecisions/s inference rate, similar to dedicated analog in-memory classifiers. In addition, a strategy for mapping/synthesizing models to this DTE architecture based on satisfiability modulo theories is proposed.
更多查看译文
关键词
atrial fibrillation,ECG,decision tree ensemble,DTE,machine learning,satisfiability,SAT,SMT,synthesis,LUT
AI 理解论文
溯源树
样例
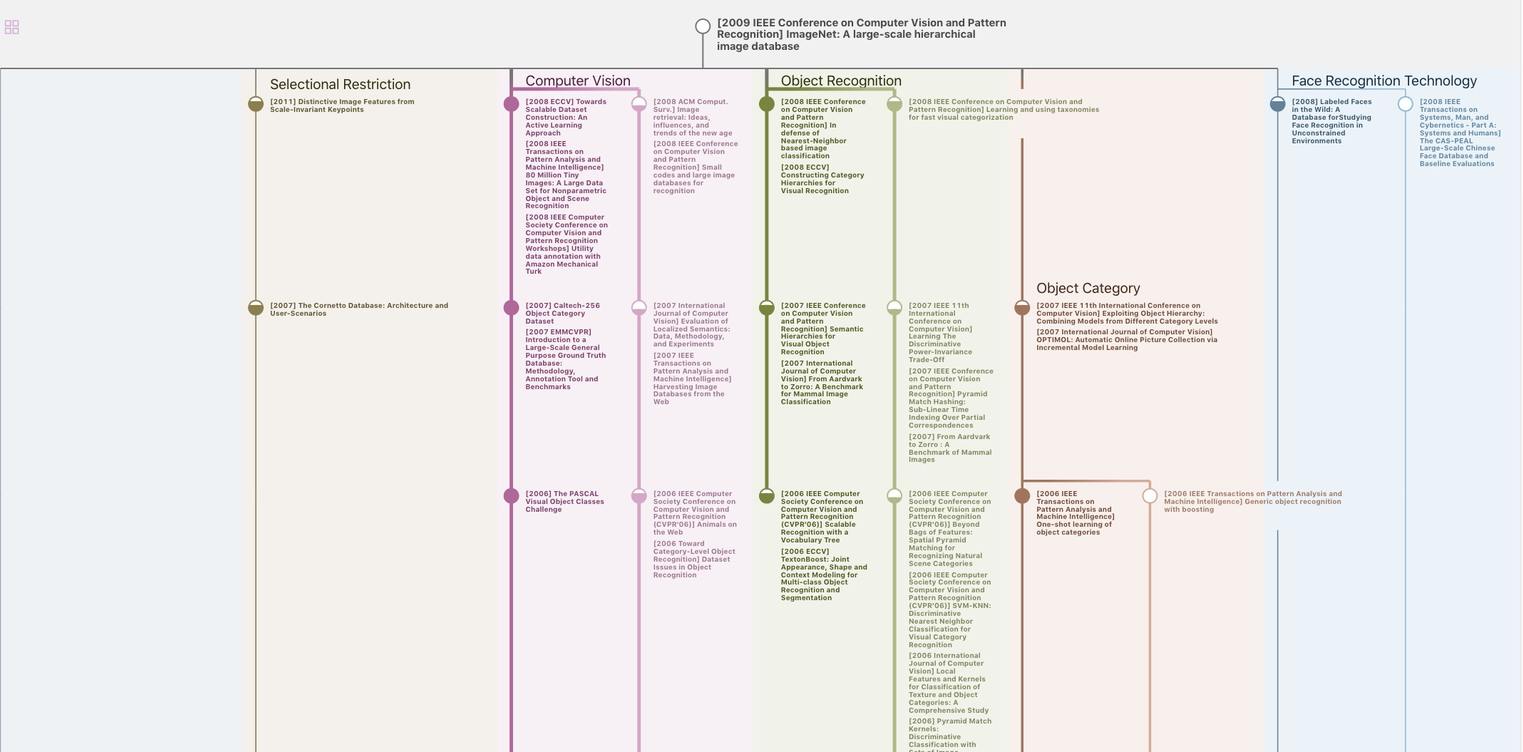
生成溯源树,研究论文发展脉络
Chat Paper
正在生成论文摘要