An improved generative adversarial network to oversample imbalanced datasets
ENGINEERING APPLICATIONS OF ARTIFICIAL INTELLIGENCE(2024)
摘要
Many oversampling methods applied to imbalanced data generate samples according to local density distribution of minority samples. However, samples generated by these methods can only present a non -deterministic relationship between the local and global distributions. A generative adversarial network (GAN) is a suitable tool to learn an unknown global probability distribution. In this paper, we propose an improved GAN (I-GAN) to oversample according to the global underlying structure of minority samples. The originality of I-GAN stems from the fact it provides additional density distribution information of minority samples for GAN and generated samples. By building on this idea, three detailed strategies are presented: input random vectors of the generator are sampled from a rough estimate of the distribution of minority samples to orientate fake samples more believable; a residual about minority samples is added into the discriminator to strengthen the constraint of loss function; generated samples are redistributed with a reshaper. These three strategies provide innovative methodologies at various stages of GANs for the oversampling task. Compared with 22 classical and popular imbalanced sampling methods under metrics of Gm, F1, and AUC on 24 benchmark imbalanced datasets, it is shown that I-GAN is effective and robust. The I-GAN implementation line procedure has been uploaded to Github (https://github.com/flowerbloom000/I-GAN).
更多查看译文
关键词
Imbalanced learning,Generative adversarial network (GAN),Oversampling,Probability distribution
AI 理解论文
溯源树
样例
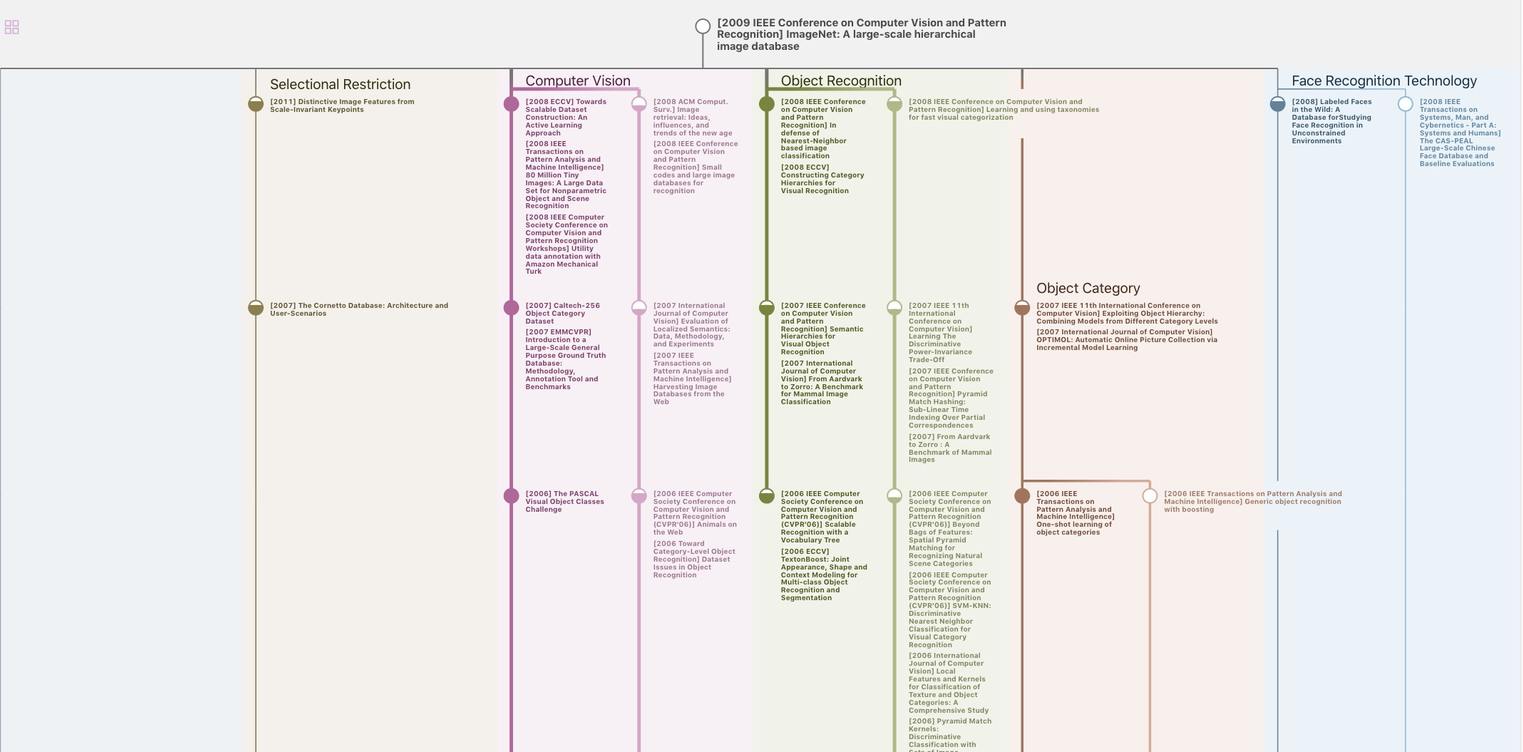
生成溯源树,研究论文发展脉络
Chat Paper
正在生成论文摘要