Few shot learning for avocado maturity determination from microwave images
JOURNAL OF AGRICULTURE AND FOOD RESEARCH(2024)
摘要
Artificial intelligence (AI) has played a critical role in the enhancement and automation of global food production and delivery. The assessment of ripeness through microwave images and AI presents a unique opportunity to enhance the current process and minimize food wastage. Particularly in avocado maturity prediction, microwave imaging provides a non-invasive solution to see through the shell and predict the maturity intelligently. However, conventional deep learning models are generally data-hungry, requiring huge amounts of training data to maintain high performance. In this paper, we propose a few-shot learning (FSL) model to address the need for a large training dataset in maturity estimation. We classify avocado microwave images for maturity assessment through a prototypical FSL model. The FSL model achieves an accuracy range of 80%-96 % across the different experimental groups and all outperforms the conventional deep learning in scenarios where limited data is available for training. This experiment demonstrates the feasibility and accuracy of utilizing microwave scanning and FSL to determine avocado maturity.
更多查看译文
关键词
Fruit maturity assessment,Avocado,Artificial intelligence,Computer vision,Few-shot learning,Microwave imaging
AI 理解论文
溯源树
样例
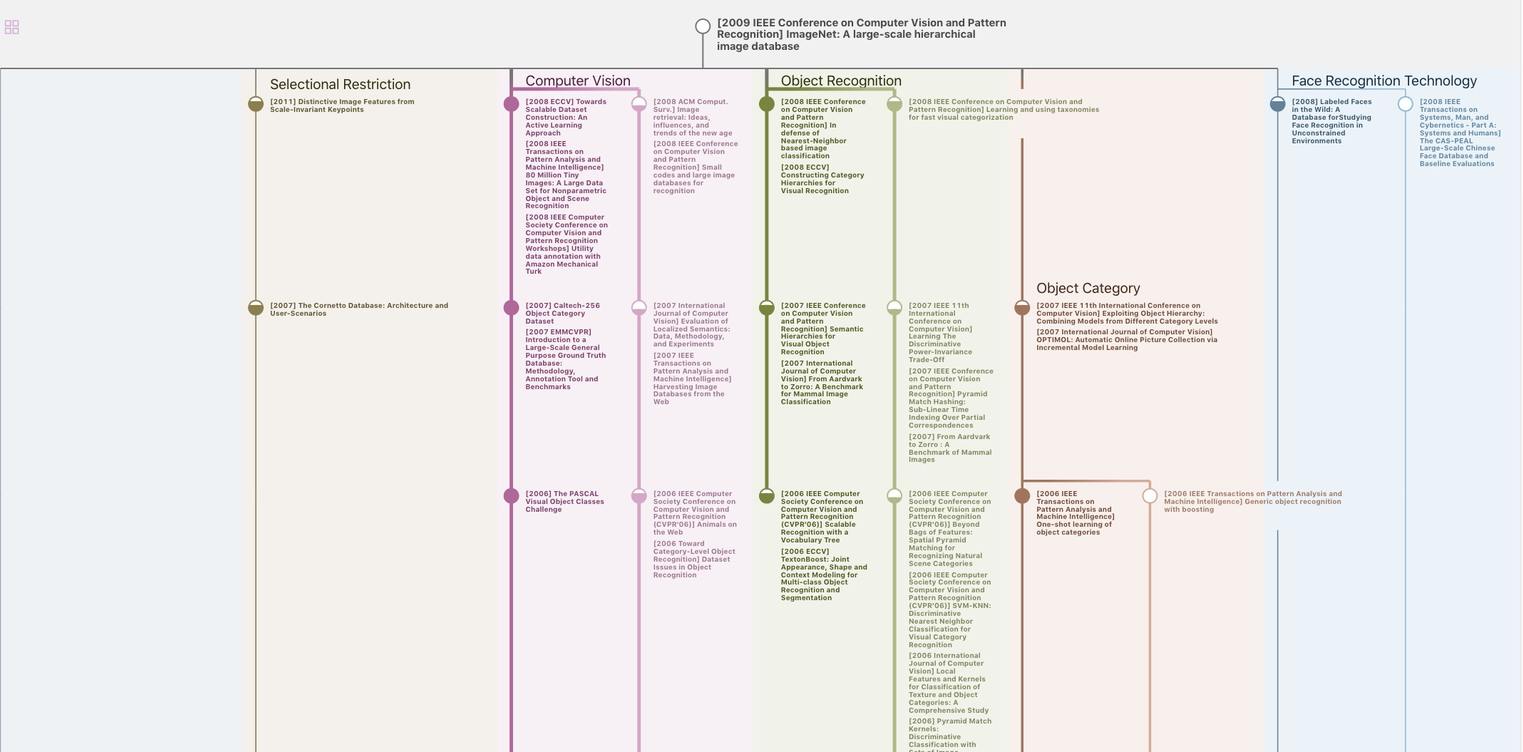
生成溯源树,研究论文发展脉络
Chat Paper
正在生成论文摘要