Effects of Urban Vegetation on Microclimate and Building Energy Demand in Winter: an Evaluation Using Coupled Simulations
SUSTAINABLE CITIES AND SOCIETY(2024)
Abstract
As an important landscape, the evergreen vegetation is prevailing, even in cold region cities. However, the impacts of promoting vegetation on microclimate and building heating load (BHL) are still inexplicit. This study validated the feasibility of a new-developed coupled model that linked ENVI-met and EnergyPlus, for predicting the microclimate and BHL in winter. The impacts of vegetation configuration changes on the performances of three typical urban blocks in Xi'an, a high-density city in the cold region of China, were then evaluated. When the leaf area index of green lands increased from 0.79 to 4.77 m2/m2, a decrease of 3.27 degrees C on mean radiant temperature, a decrease of 0.48 m/s on wind speed, and an increase of 0.47 degrees C on air temperature at the block scale could be observed. To the three blocks, the BHL of thermal zones in the height range of tree canopy increased by 7.16 %, 1.52 % and 3.57 % during a sunny day, and decreased by 2.80 %, 0.55 %, and 0.06 % during a cloudy day maximally. Overall, the evergreen vegetation produced negative impacts on the building energy efficiency of cold region cities. For urban planners, it's advisable to concentrate evergreen plants in highrise blocks to mitigate such disadvantages.
MoreTranslated text
Key words
Urban vegetation,Microclimate,Building energy,Coupled simulation
求助PDF
上传PDF
View via Publisher
AI Read Science
AI Summary
AI Summary is the key point extracted automatically understanding the full text of the paper, including the background, methods, results, conclusions, icons and other key content, so that you can get the outline of the paper at a glance.
Example
Background
Key content
Introduction
Methods
Results
Related work
Fund
Key content
- Pretraining has recently greatly promoted the development of natural language processing (NLP)
- We show that M6 outperforms the baselines in multimodal downstream tasks, and the large M6 with 10 parameters can reach a better performance
- We propose a method called M6 that is able to process information of multiple modalities and perform both single-modal and cross-modal understanding and generation
- The model is scaled to large model with 10 billion parameters with sophisticated deployment, and the 10 -parameter M6-large is the largest pretrained model in Chinese
- Experimental results show that our proposed M6 outperforms the baseline in a number of downstream tasks concerning both single modality and multiple modalities We will continue the pretraining of extremely large models by increasing data to explore the limit of its performance
Upload PDF to Generate Summary
Must-Reading Tree
Example
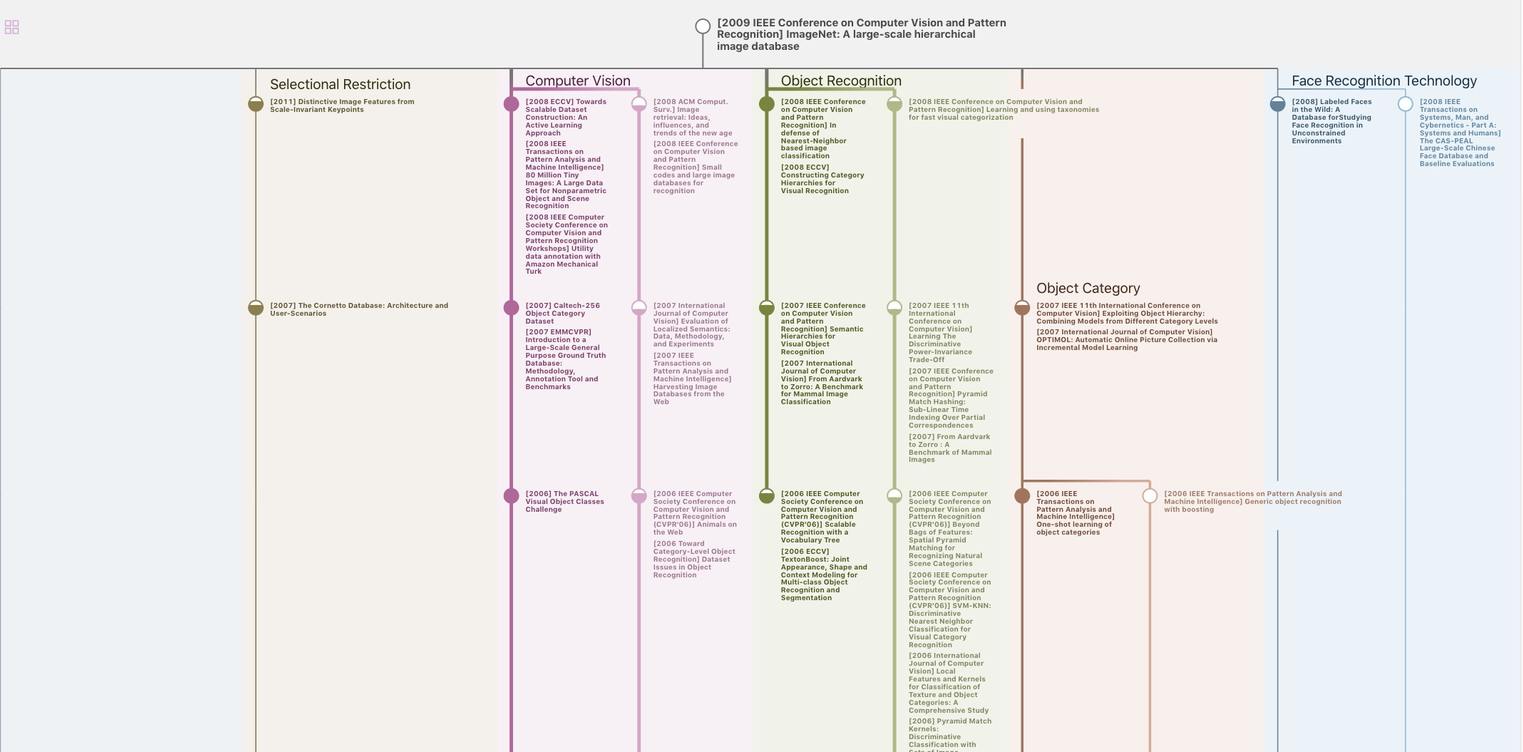
Generate MRT to find the research sequence of this paper
Related Papers
ENERGIES 2024
被引用1
GEOMATICS NATURAL HAZARDS & RISK 2024
被引用1
Sustainability 2024
被引用0
Rangeland Ecology & Management 2024
被引用0
Journal of Environmental Management 2024
被引用0
HYDROLOGICAL PROCESSES 2024
被引用1
PHYSICS AND CHEMISTRY OF THE EARTH 2024
被引用0
An Advanced Hybrid Deep Learning Model for Accurate Energy Load Prediction in Smart Building
ENERGY EXPLORATION & EXPLOITATION 2024
被引用0
Data Disclaimer
The page data are from open Internet sources, cooperative publishers and automatic analysis results through AI technology. We do not make any commitments and guarantees for the validity, accuracy, correctness, reliability, completeness and timeliness of the page data. If you have any questions, please contact us by email: report@aminer.cn
Chat Paper