A Gibbs Posterior Framework for Fair Clustering
ENTROPY(2024)
摘要
The rise of machine learning-driven decision-making has sparked a growing emphasis on algorithmic fairness. Within the realm of clustering, the notion of balance is utilized as a criterion for attaining fairness, which characterizes a clustering mechanism as fair when the resulting clusters maintain a consistent proportion of observations representing individuals from distinct groups delineated by protected attributes. Building on this idea, the literature has rapidly incorporated a myriad of extensions, devising fair versions of the existing frequentist clustering algorithms, e.g., k-means, k-medioids, etc., that aim at minimizing specific loss functions. These approaches lack uncertainty quantification associated with the optimal clustering configuration and only provide clustering boundaries without quantifying the probabilities associated with each observation belonging to the different clusters. In this article, we intend to offer a novel probabilistic formulation of the fair clustering problem that facilitates valid uncertainty quantification even under mild model misspecifications, without incurring substantial computational overhead. Mixture model-based fair clustering frameworks facilitate automatic uncertainty quantification, but tend to showcase brittleness under model misspecification and involve significant computational challenges. To circumnavigate such issues, we propose a generalized Bayesian fair clustering framework that inherently enjoys decision-theoretic interpretation. Moreover, we devise efficient computational algorithms that crucially leverage techniques from the existing literature on optimal transport and clustering based on loss functions. The gain from the proposed technology is showcased via numerical experiments and real data examples.
更多查看译文
关键词
algorithmic fairness,balance,generalized Bayes,minimum cost flow,optimal transport
AI 理解论文
溯源树
样例
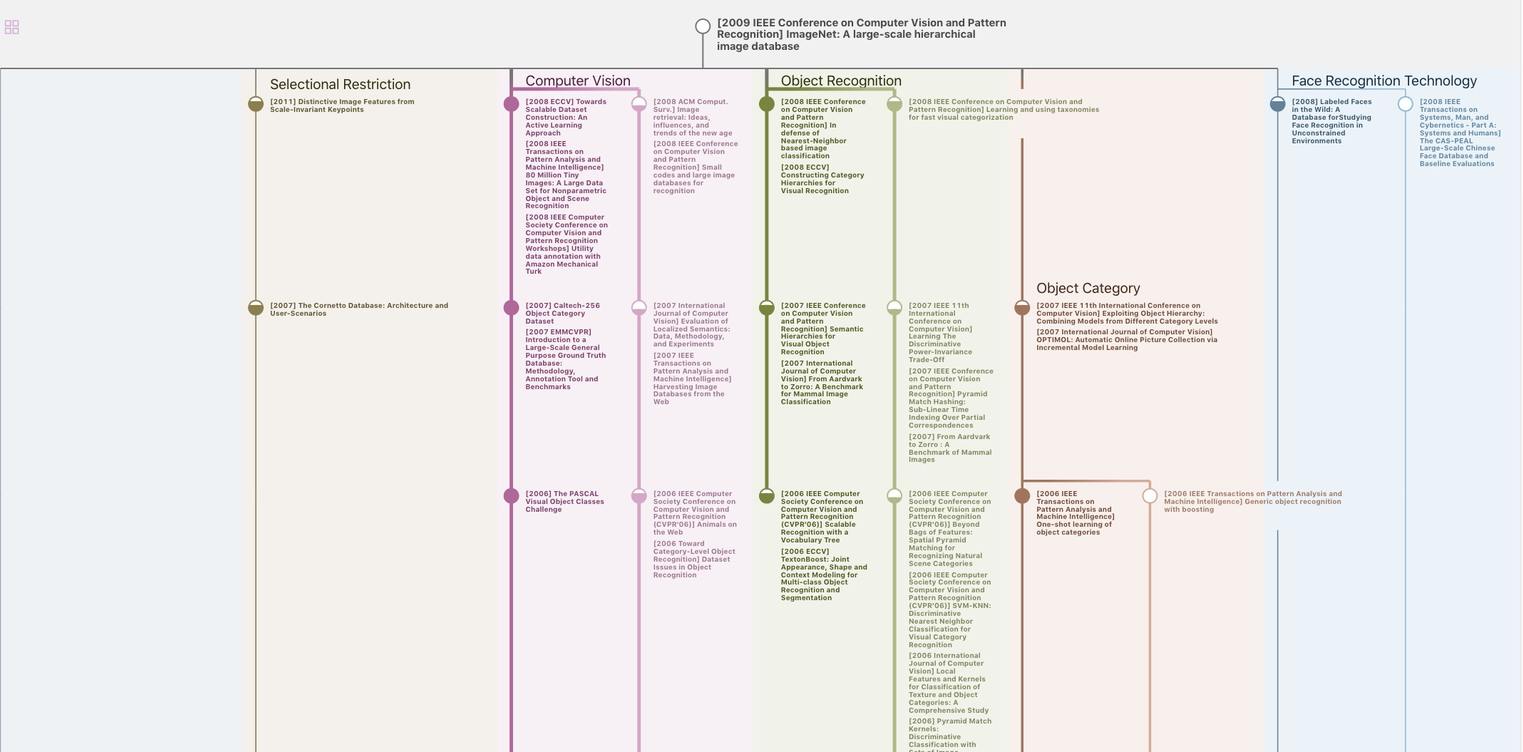
生成溯源树,研究论文发展脉络
Chat Paper
正在生成论文摘要