Learning Quadrupedal High-Speed Running on Uneven Terrain
BIOMIMETICS(2024)
摘要
Reinforcement learning (RL)-based controllers have been applied to the high-speed movement of quadruped robots on uneven terrains. The external disturbances increase as the robot moves faster on such terrains, affecting the stability of the robot. Many existing RL-based methods adopt higher control frequencies to respond quickly to the disturbance, which requires a significant computational cost. We propose a control framework that consists of an RL-based control policy updating at a low frequency and a model-based joint controller updating at a high frequency. Unlike previous methods, our policy outputs the control law for each joint, executed by the corresponding high-frequency joint controller to reduce the impact of external disturbances on the robot. We evaluated our method on various simulated terrains with height differences of up to 6 cm. We achieved a running motion of 1.8 m/s in the simulation using the Unitree A1 quadruped. The RL-based control policy updates at 50 Hz with a latency of 20 ms, while the model-based joint controller runs at 1000 Hz. The experimental results show that the proposed framework can overcome the latency caused by low-frequency updates, making it applicable for real-robot deployment.
更多查看译文
关键词
reinforcement learning,quadrupedal robot,high-speed locomotion
AI 理解论文
溯源树
样例
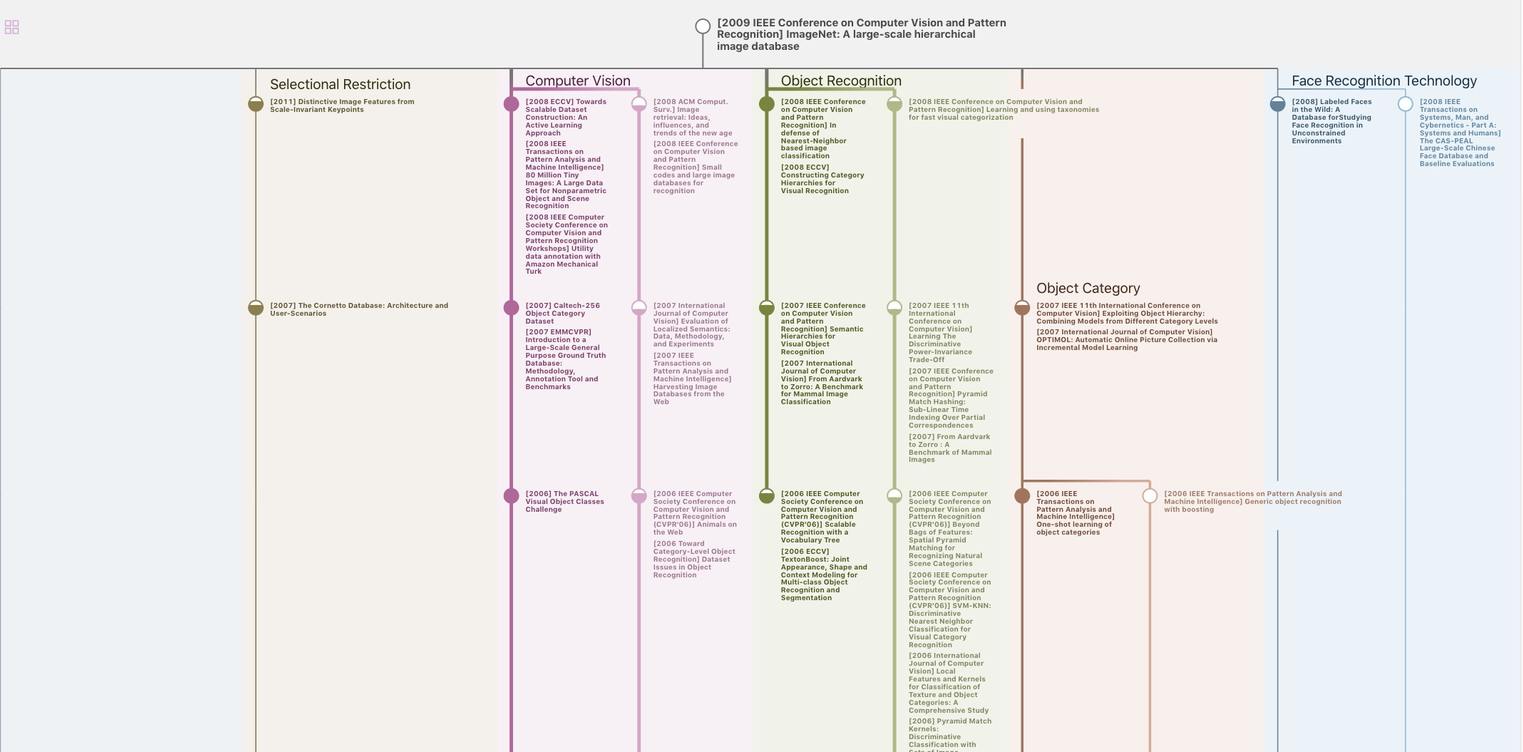
生成溯源树,研究论文发展脉络
Chat Paper
正在生成论文摘要