Towards Robust Learning with Noisy and Pseudo Labels for Text Classification
INFORMATION SCIENCES(2024)
摘要
Unlike Positive Training (PT), Negative Training (NT) is an indirect learning technique that trains the model on a combination of clean and noisy data using complementary labels, which are randomly generated from the label space except for the actual label. Although clean samples have identical distributions to the test samples, they are treated with the same level of uncertainty as noisy samples because of the complementary labeling of NT. Consequently, their contribution to the overall performance is relatively lower. We propose a Learning with Noisy and Pseudo Label (LNPL) framework, which jointly trains the model using PT and NT on clean and noisy data, respectively. We aim to enable direct learning on clean samples while leveraging the robustness of NT against noise in a unified framework. To mitigate the abundance of noisy instances, we leverage a gradient reversal layer at the top of LNPL as a regularization term to mislead the recognition of the source of the instance (e.g., clean or noisy). Moreover, we introduce a selftraining LNPL that performs a semi -supervised text classification task as a learning with noisy pseudo -label problem. Extensive experiments on various textual benchmark datasets demonstrate that LNPL is robust and consistently outperforms the alternatives. The code is available on GitHub.1
更多查看译文
关键词
Natural language processing,Negative learning,Learning with noisy labels,Semi-supervised text classification
AI 理解论文
溯源树
样例
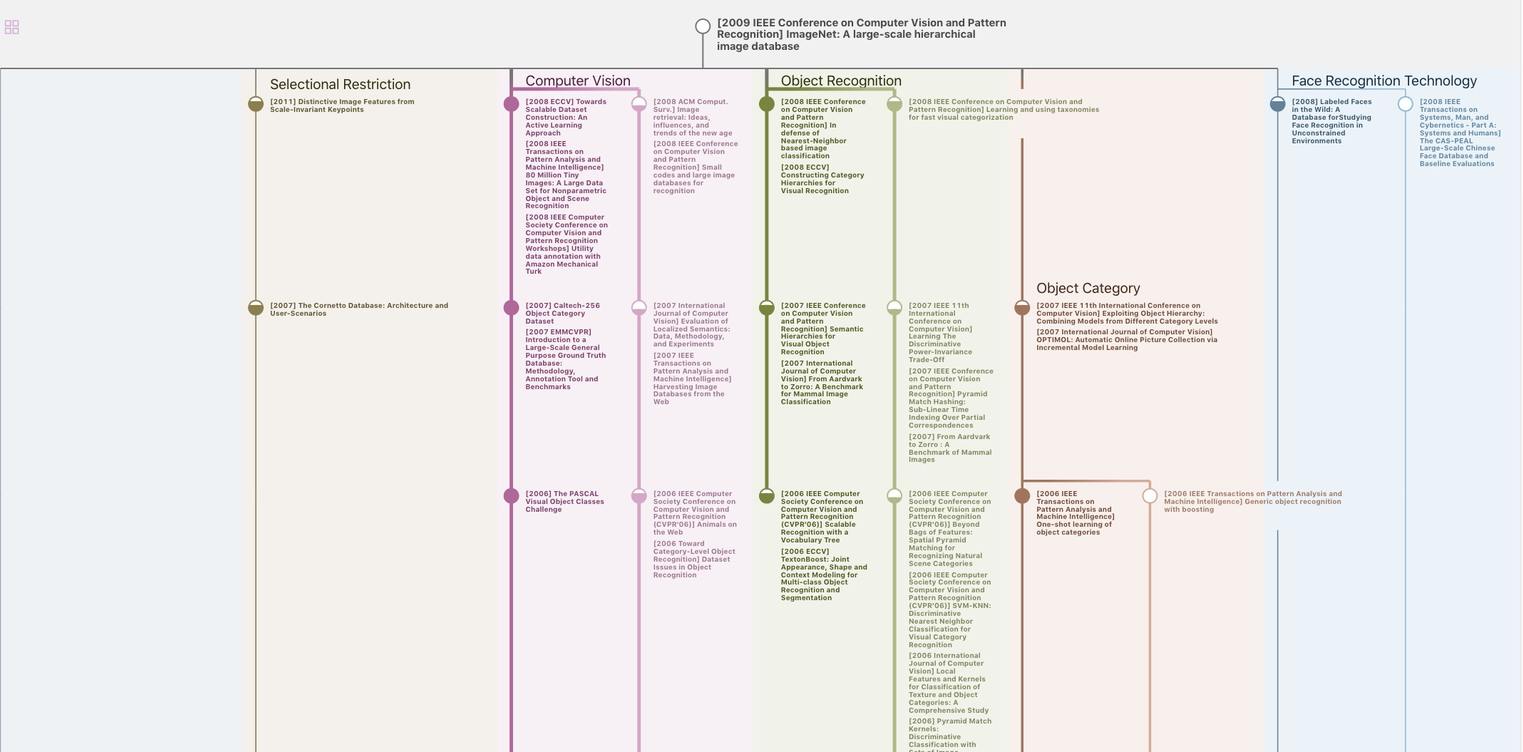
生成溯源树,研究论文发展脉络
Chat Paper
正在生成论文摘要