LKFormer: large kernel transformer for infrared image super-resolution
Multimedia Tools and Applications(2024)
摘要
Given the broad application of infrared technology across diverse fields, there is an increasing emphasis on investigating super-resolution techniques for infrared images within the realm of deep learning. Despite the impressive results of current Transformer-based methods in image super-resolution tasks, their reliance on the self-attention mechanism intrinsic to the Transformer architecture results in images being treated as one-dimensional sequences, thereby neglecting their inherent two-dimensional structure. Moreover, infrared images exhibit a uniform pixel distribution and a limited gradient range, posing challenges for the model to capture effective feature information. Consequently, we suggest a potent Transformer model, termed Large Kernel Transformer (LKFormer), to address this issue. Specifically, we have designed a Large Kernel Residual Depth-wise Convolutional Attention (LKRDA) module with linear complexity. This mainly employs depth-wise convolution with large kernels to execute non-local feature modeling, thereby substituting the standard self-attention layer. Additionally, we have devised a novel feed-forward network structure called Gated-Pixel Feed-Forward Network (GPFN) to augment the LKFormer’s capacity to manage the information flow within the network. Comprehensive experimental results reveal that our method surpasses the most advanced techniques available, using fewer parameters and yielding considerably superior performance.
更多查看译文
关键词
Infrared image,Super-resolution,Deep learning,Large kernel convolution
AI 理解论文
溯源树
样例
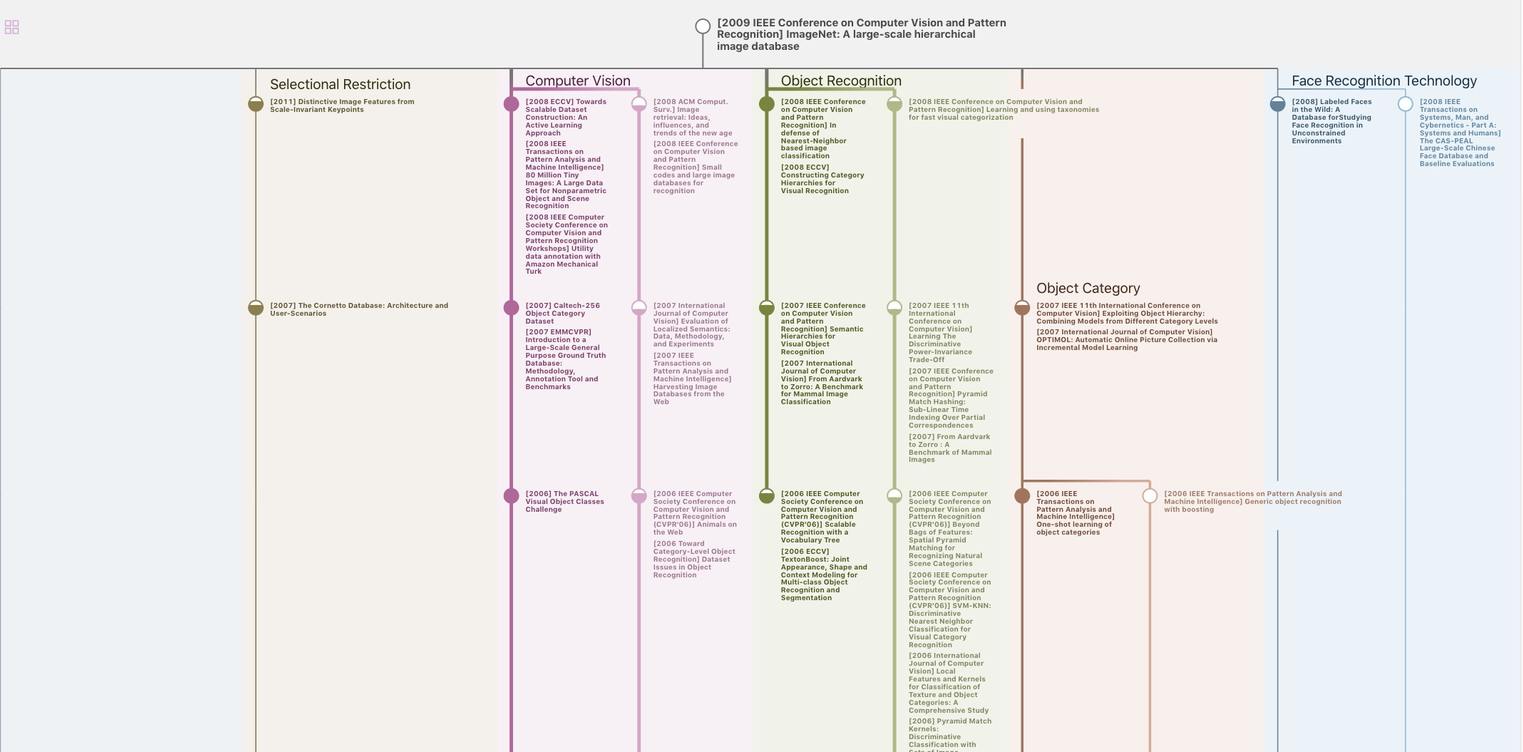
生成溯源树,研究论文发展脉络
Chat Paper
正在生成论文摘要