Time-Delayed Koopman Network-Based Model Predictive Control for the FRIB RFQ
arxiv(2024)
摘要
The radio-frequency quadrupole (RFQ) at the Facility for Rare Isotope Beams
(FRIB) is a critical device to accelerate heavy ion beams from 12 keV/u to 0.5
MeV/u for state-of-the-art nuclear physics experiments. Efficient control of
the RFQ resonance frequency detuning still remains a challenge because the
temperature-sensitive frequency is solely control by a cooling water system,
exhibiting complicated transport delay and nonlinearity in the heat transfer
processes. In this work, we propose a long-short term memory (LSTM)-based
Koopman network model that can simultaneously learn the time-delayed and
non-delayed correlations hidden in the historical operating data. It is proven
that the model can effectively predict the behavior of the RFQ resonance
frequency using historical data as inputs. With this model, a model predictive
control (MPC) framework based on the Newton-Raphson method is proposed and
tested. We demonstrate that the MPC framework utilizing deep learning model is
able to provide precise and rapid control for the RFQ frequency detuning,
reducing the control time by half compared to the
proportional-integral-derivative (PID) controller.
更多查看译文
AI 理解论文
溯源树
样例
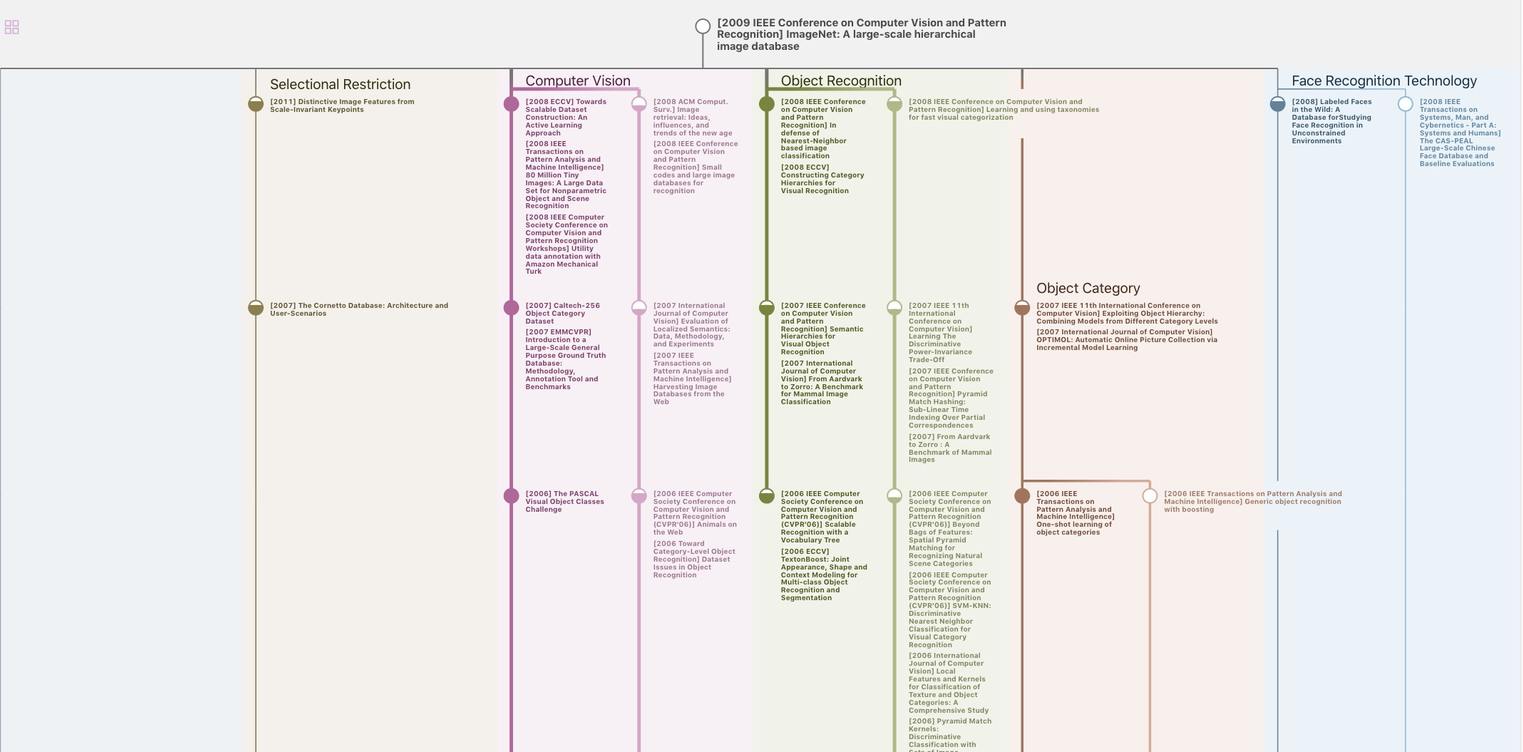
生成溯源树,研究论文发展脉络
Chat Paper
正在生成论文摘要