Simulating Nighttime Visible Satellite Imagery of Tropical Cyclones Using Conditional Generative Adversarial Networks
CoRR(2024)
摘要
Visible (VIS) imagery of satellites has various important applications in
meteorology, including monitoring Tropical Cyclones (TCs). However, it is
unavailable at night because of the lack of sunlight. This study presents a
Conditional Generative Adversarial Networks (CGAN) model that generates highly
accurate nighttime visible reflectance using infrared (IR) bands and sunlight
direction parameters as input. The model was trained and validated using target
area observations of the Advanced Himawari Imager (AHI) in the daytime. This
study also presents the first nighttime model validation using the Day/Night
Band (DNB) of the Visible/Infrared Imager Radiometer Suite (VIIRS). The daytime
statistical results of the Structural Similarity Index Measure (SSIM), Peak
Signal-to-Noise Ratio (PSNR), Root Mean Square Error (RMSE), Correlation
Coefficient (CC), and Bias are 0.885, 28.3, 0.0428, 0.984, and -0.0016
respectively, completely surpassing the model performance of previous studies.
The nighttime statistical results of SSIM, PSNR, RMSE, and CC are 0.821, 24.4,
0.0643, and 0.969 respectively, which are slightly negatively impacted by the
parallax between satellites. We performed full-disk model validation which
proves our model could also be readily applied in the tropical ocean without
TCs in the northern hemisphere. This model contributes to the nighttime
monitoring of meteorological phenomena by providing accurate AI-generated
visible imagery with adjustable virtual sunlight directions.
更多查看译文
AI 理解论文
溯源树
样例
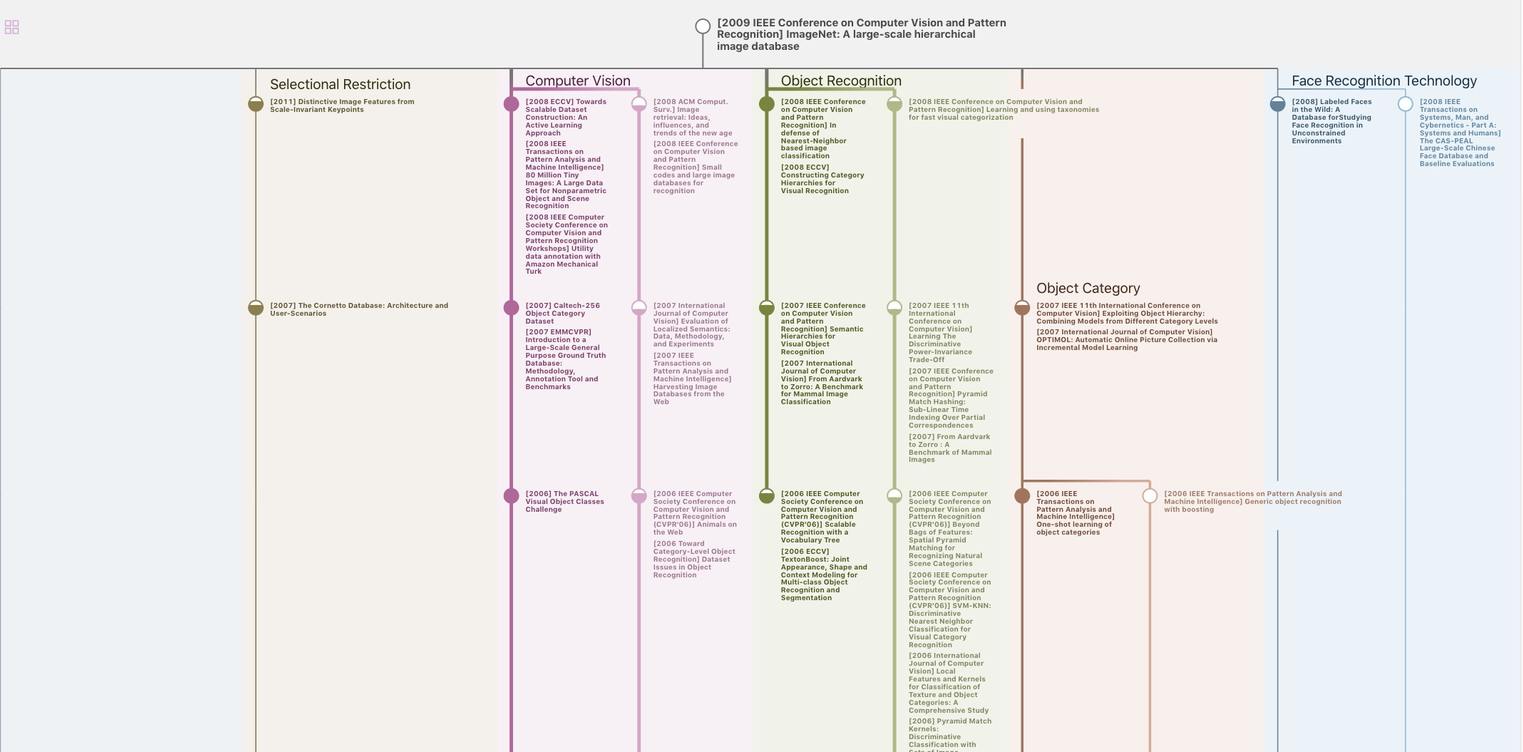
生成溯源树,研究论文发展脉络
Chat Paper
正在生成论文摘要