What Are We Optimizing For? A Human-centric Evaluation Of Deep Learning-based Recommender Systems
CoRR(2024)
摘要
Deep learning-based (DL) models in recommender systems (RecSys) have gained
significant recognition for their remarkable accuracy in predicting user
preferences. However, their performance often lacks a comprehensive evaluation
from a human-centric perspective, which encompasses various dimensions beyond
simple interest matching. In this work, we have developed a robust
human-centric evaluation framework that incorporates seven diverse metrics to
assess the quality of recommendations generated by five recent open-sourced DL
models. Our evaluation datasets consist of both offline benchmark data and
personalized online recommendation feedback collected from 445 real users. We
find that (1) different DL models have different pros and cons in the
multi-dimensional metrics that we test with; (2) users generally want a
combination of accuracy with at least one another human values in the
recommendation; (3) the degree of combination of different values needs to be
carefully experimented to user preferred level.
更多查看译文
AI 理解论文
溯源树
样例
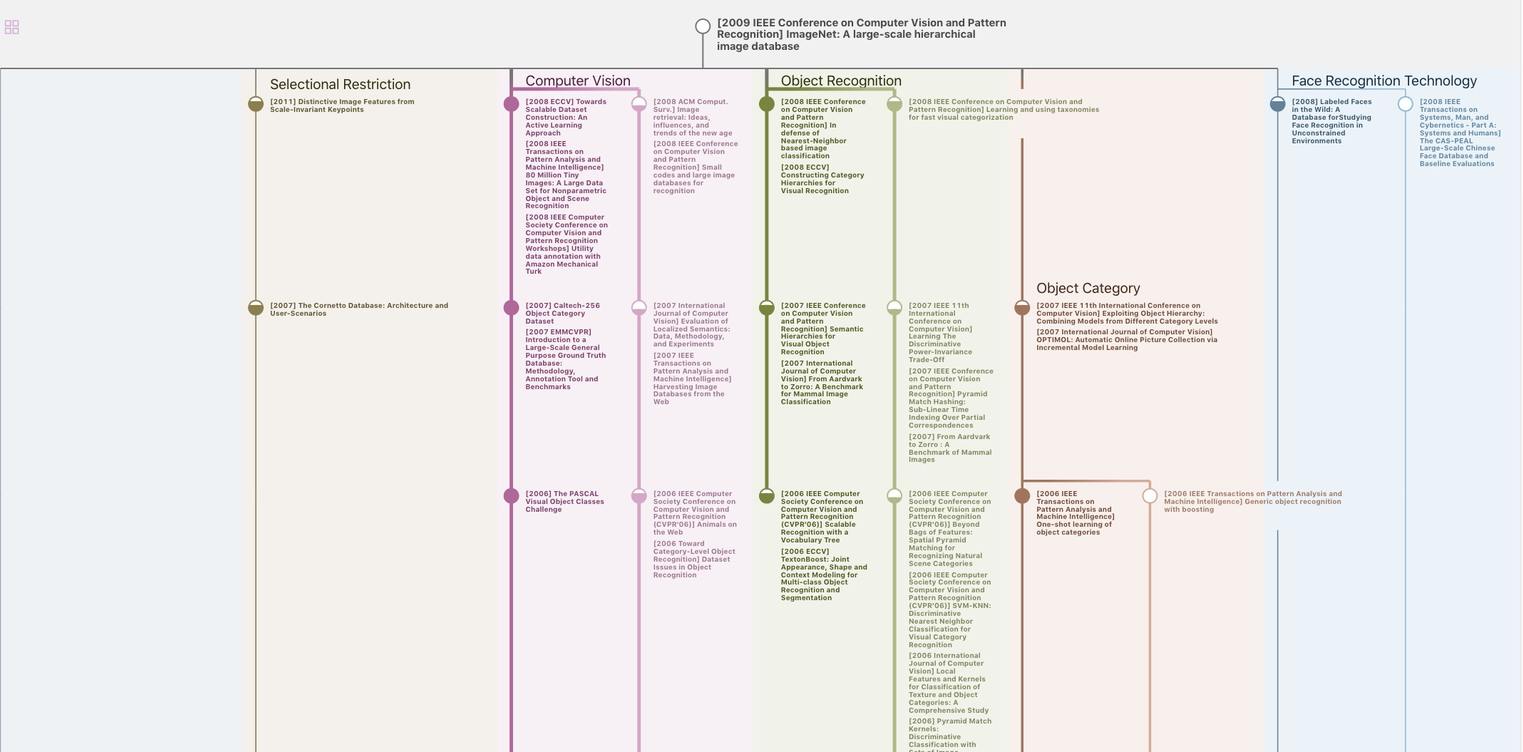
生成溯源树,研究论文发展脉络
Chat Paper
正在生成论文摘要