P2DT: Mitigating Forgetting in task-incremental Learning with progressive prompt Decision Transformer
CoRR(2024)
摘要
Catastrophic forgetting poses a substantial challenge for managing
intelligent agents controlled by a large model, causing performance degradation
when these agents face new tasks. In our work, we propose a novel solution -
the Progressive Prompt Decision Transformer (P2DT). This method enhances a
transformer-based model by dynamically appending decision tokens during new
task training, thus fostering task-specific policies. Our approach mitigates
forgetting in continual and offline reinforcement learning scenarios. Moreover,
P2DT leverages trajectories collected via traditional reinforcement learning
from all tasks and generates new task-specific tokens during training, thereby
retaining knowledge from previous studies. Preliminary results demonstrate that
our model effectively alleviates catastrophic forgetting and scales well with
increasing task environments.
更多查看译文
关键词
Continual Learning,Offline Reinforcement Learning,Prompt Learning,AI Agent
AI 理解论文
溯源树
样例
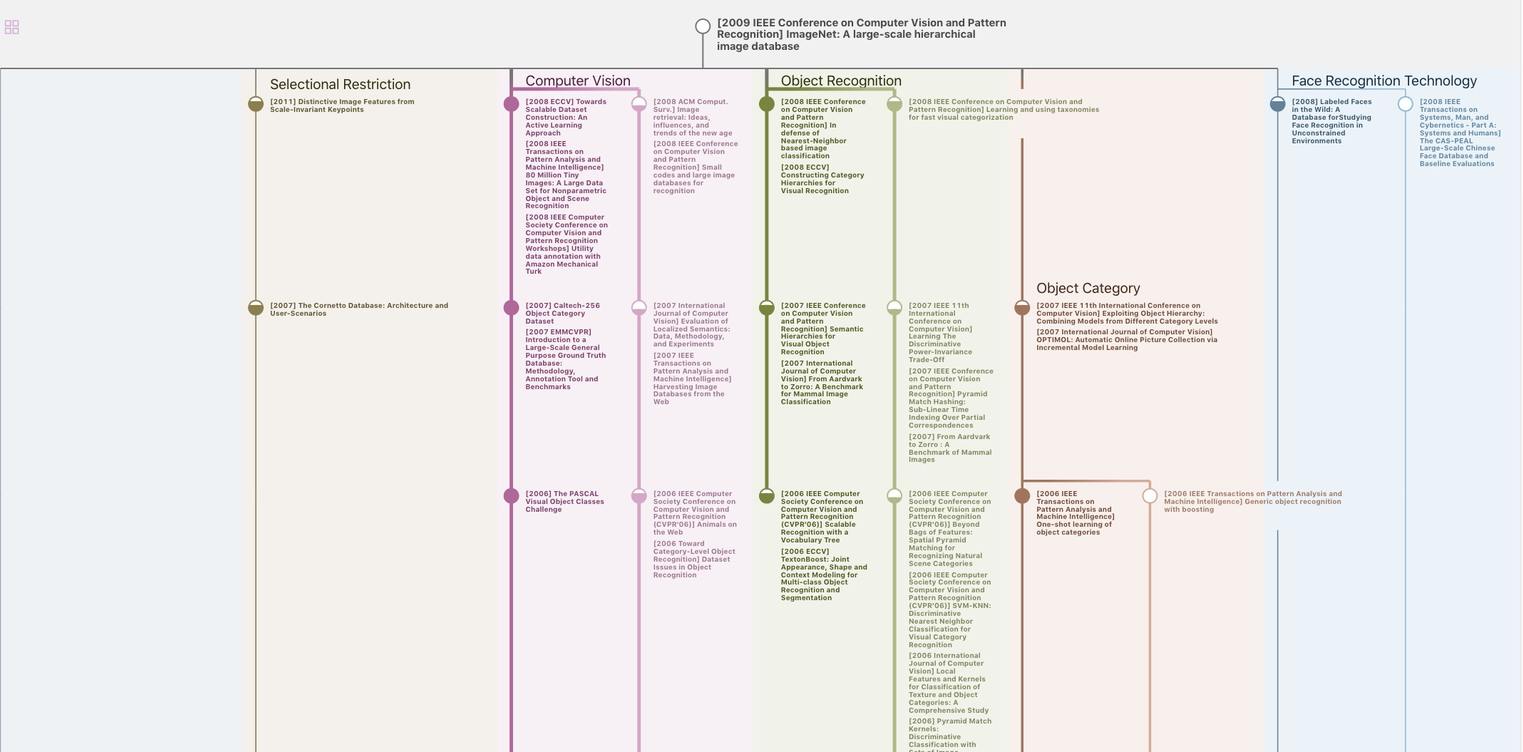
生成溯源树,研究论文发展脉络
Chat Paper
正在生成论文摘要