Stereo-Matching Knowledge Distilled Monocular Depth Estimation Filtered by Multiple Disparity Consistency
CoRR(2024)
摘要
In stereo-matching knowledge distillation methods of the self-supervised
monocular depth estimation, the stereo-matching network's knowledge is
distilled into a monocular depth network through pseudo-depth maps. In these
methods, the learning-based stereo-confidence network is generally utilized to
identify errors in the pseudo-depth maps to prevent transferring the errors.
However, the learning-based stereo-confidence networks should be trained with
ground truth (GT), which is not feasible in a self-supervised setting. In this
paper, we propose a method to identify and filter errors in the pseudo-depth
map using multiple disparity maps by checking their consistency without the
need for GT and a training process. Experimental results show that the proposed
method outperforms the previous methods and works well on various
configurations by filtering out erroneous areas where the stereo-matching is
vulnerable, especially such as textureless regions, occlusion boundaries, and
reflective surfaces.
更多查看译文
关键词
monocular depth estimation,deep learning,self-supervision,stereo-matching
AI 理解论文
溯源树
样例
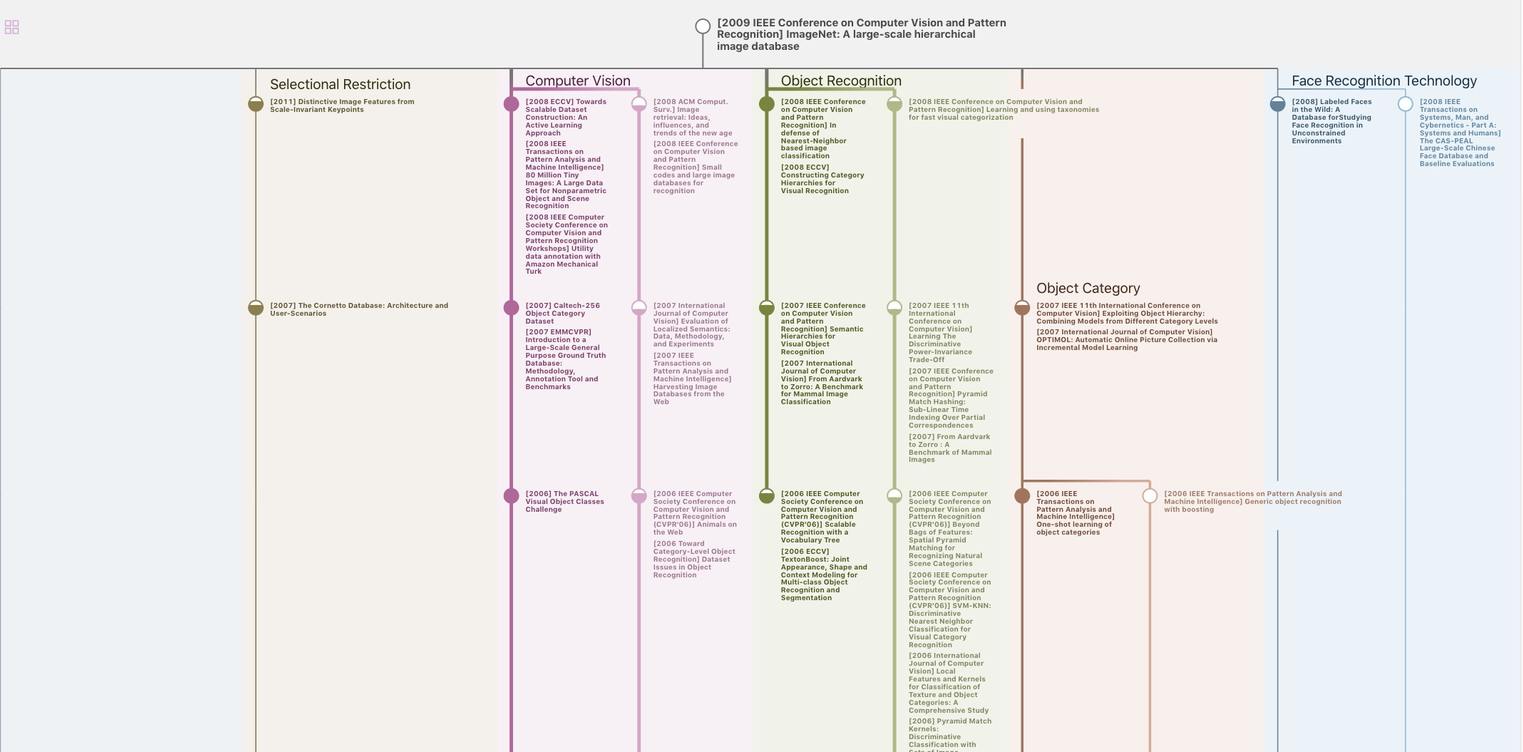
生成溯源树,研究论文发展脉络
Chat Paper
正在生成论文摘要