Universal Neurons in GPT2 Language Models
Trans Mach Learn Res(2024)
摘要
A basic question within the emerging field of mechanistic interpretability isthe degree to which neural networks learn the same underlying mechanisms. Inother words, are neural mechanisms universal across different models? In thiswork, we study the universality of individual neurons across GPT2 modelstrained from different initial random seeds, motivated by the hypothesis thatuniversal neurons are likely to be interpretable. In particular, we computepairwise correlations of neuron activations over 100 million tokens for everyneuron pair across five different seeds and find that 1-5% of neurons areuniversal, that is, pairs of neurons which consistently activate on the sameinputs. We then study these universal neurons in detail, finding that theyusually have clear interpretations and taxonomize them into a small number ofneuron families. We conclude by studying patterns in neuron weights toestablish several universal functional roles of neurons in simple circuits:deactivating attention heads, changing the entropy of the next tokendistribution, and predicting the next token to (not) be within a particularset.
更多查看译文
AI 理解论文
溯源树
样例
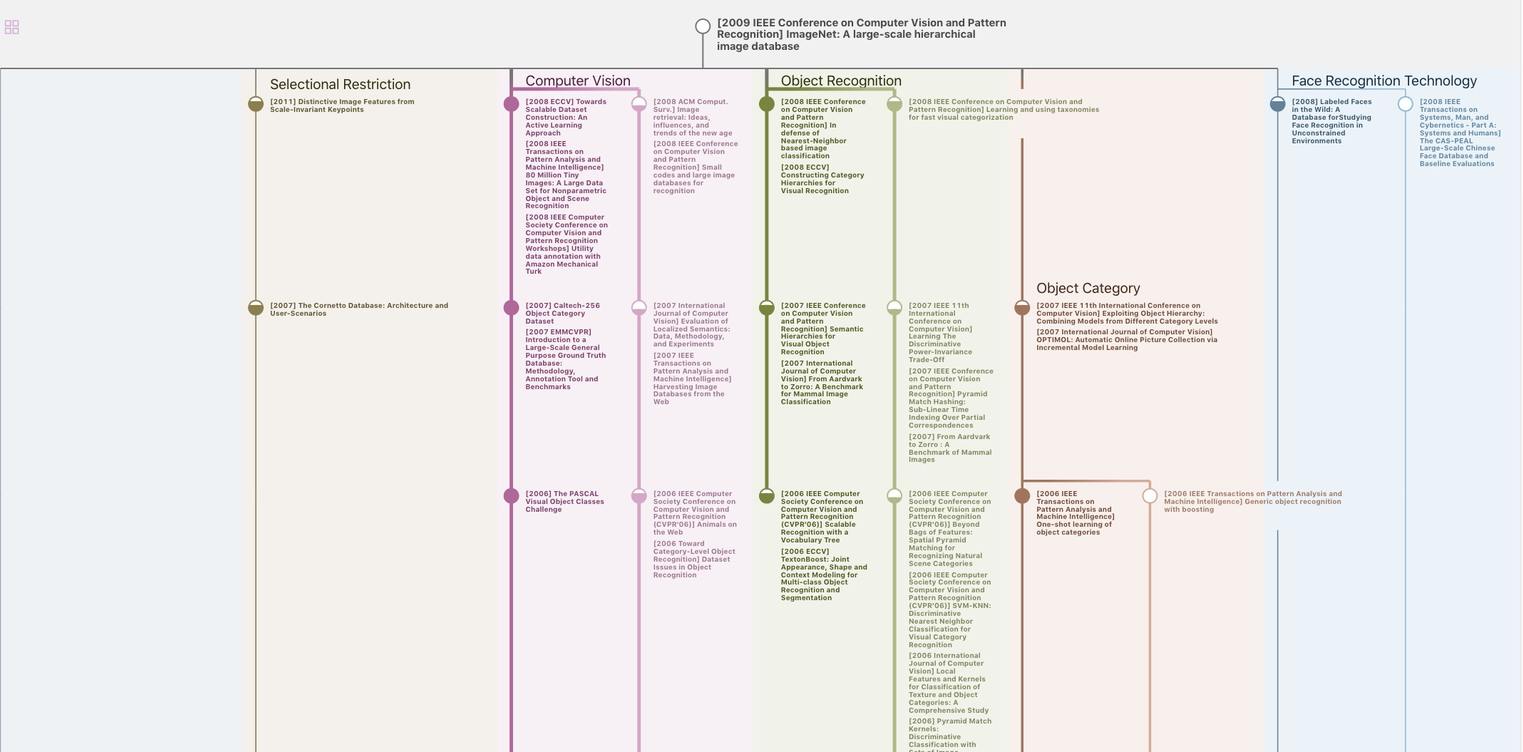
生成溯源树,研究论文发展脉络
Chat Paper
正在生成论文摘要