CrowdGraph: Weakly supervised Crowd Counting via Pure Graph Neural Network
ACM TRANSACTIONS ON MULTIMEDIA COMPUTING COMMUNICATIONS AND APPLICATIONS(2024)
摘要
Most existing weakly supervised crowd counting methods utilize Convolutional Neural Networks (CNN) or Transformer to estimate the total number of individuals in an image. However, both CNN-based (grid-to-count paradigm) and Transformer-based (sequence-to-count paradigm) methods take images as inputs in a regular form. This approach treats all pixels equally but cannot address the uneven distribution problem within human crowds. This challenge would lead to a decline in the counting performance of the model. Compared with grid and sequence, the graph structure could better explore the relationship among features. In this article, we propose a new graph-based crowd counting method named CrowdGraph, which reinterprets the weakly supervised crowd counting problem from a graph-to-count perspective. In the proposed CrowdGraph, each image is constructed as a graph, and a graph-based network is designed to extract features at the graph level. CrowdGraph comprises three main components: a dynamic graph convolutional backbone, a multi-scale dilated graph convolution module, and a regression head. To the best of our knowledge, CrowdGraph is the first method that is completely formulated based on the Graph Neural Network (GNN) for the crowd counting task. Extensive experiments demonstrate that the proposed CrowdGraph outperforms pure CNN-based and pure Transformer-based weakly supervised methods comprehensively and achieves highly competitive counting performance.
更多查看译文
关键词
Crowd counting,weakly supervised learning,graph neural network,uneven distribution of crowds
AI 理解论文
溯源树
样例
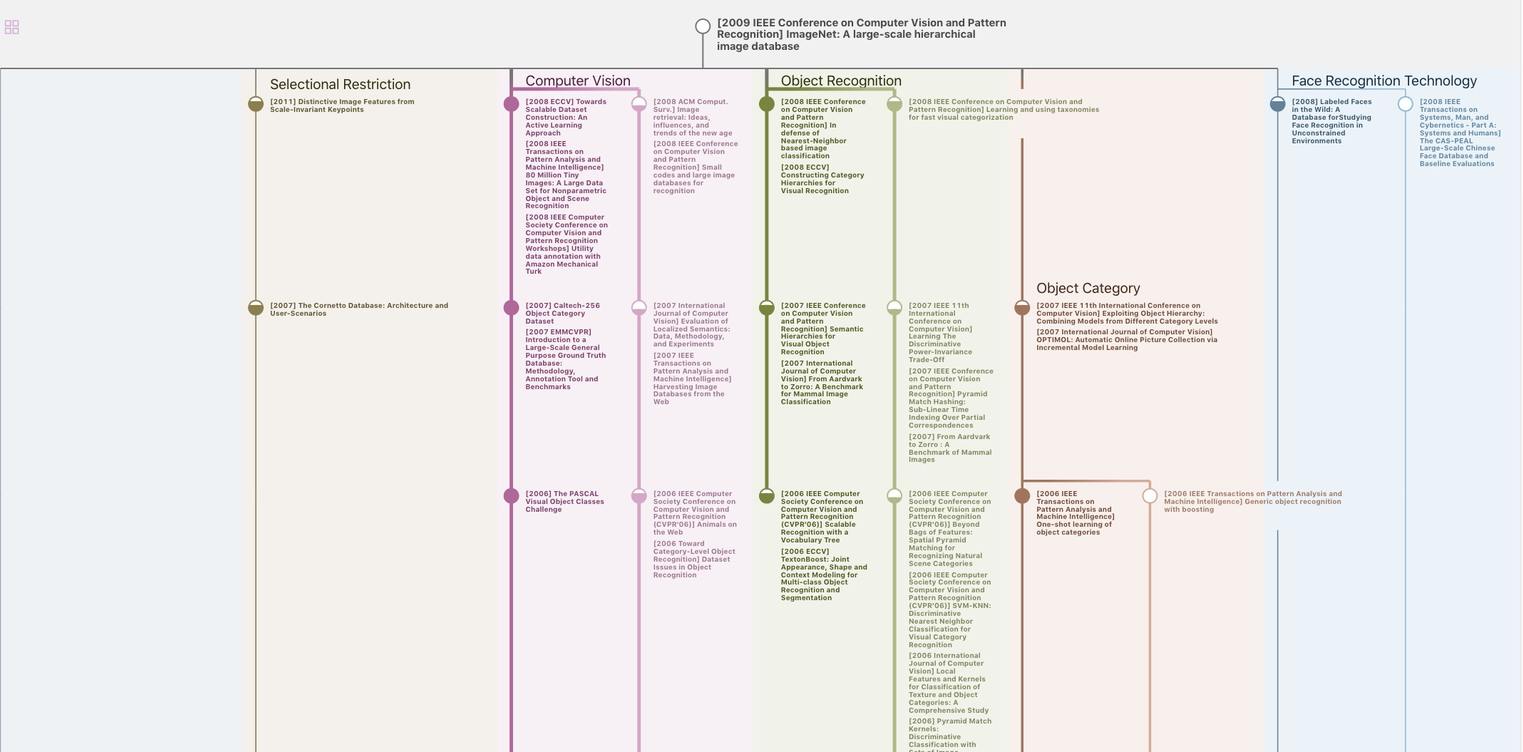
生成溯源树,研究论文发展脉络
Chat Paper
正在生成论文摘要