HARDSEA: Hybrid Analog-ReRAM Clustering and Digital-SRAM In-Memory Computing Accelerator for Dynamic Sparse Self-Attention in Transformer
IEEE TRANSACTIONS ON VERY LARGE SCALE INTEGRATION (VLSI) SYSTEMS(2024)
摘要
Self-attention-based transformers have outperformed recurrent and convolutional neural networks (RNN/ CNNs) in many applications. Despite the effectiveness, calculating self-attention is prohibitively costly due to quadratic computation and memory requirements. To solve this challenge, this article proposes a hybrid analog-ReRAM and digital-SRAM in-memory computing accelerator (HARDSEA), a computing-in-memory (CIM) accelerator supporting self-attention in transformer applications. To trade off between energy efficiency and algorithm accuracy, HARDSEA features an algorithm-architecture-circuit codesign. A product-quantization-based scheme dynamically facilitates self-attention sparsity by predicting lightweight token relevance. A hybrid in-memory computing architecture employs both high-efficiency analog ReRAM-CIM and high-precision digital SRAM-CIM to implement the proposed new scheme. The ReRAM-CIM, whose precision is sensitive to circuit nonidealities, takes charge of token relevance prediction where only computing monotonicity is demanded. The SRAM-CIM, utilized for exact sparse attention computing, is reorganized as an on-memory-boundary computing scheme, thus adapting to irregular sparsity patterns. In addition, we propose a time-domain winner-take-all (WTA) circuit to replace the expensive ADCs in ReRAM-CIM macros. Experimental results show that HARDSEA prunes BERT and GPT-2 models to 12%-33% sparsity without accuracy loss, achieving 13.5x13.5x- 28.5x28.5x speedup and 291.6x291.6x- 1894.3x1894.3x energy efficiency over GPU. Compared to state-of-the-art transformer accelerators, HARDSEA has 1.2x1.2x- 14.9x14.9x better energy efficiency at the same level of throughput.
更多查看译文
关键词
Algorithm-architecture-circuit co-design,in-memory computing,self-attention,transformer
AI 理解论文
溯源树
样例
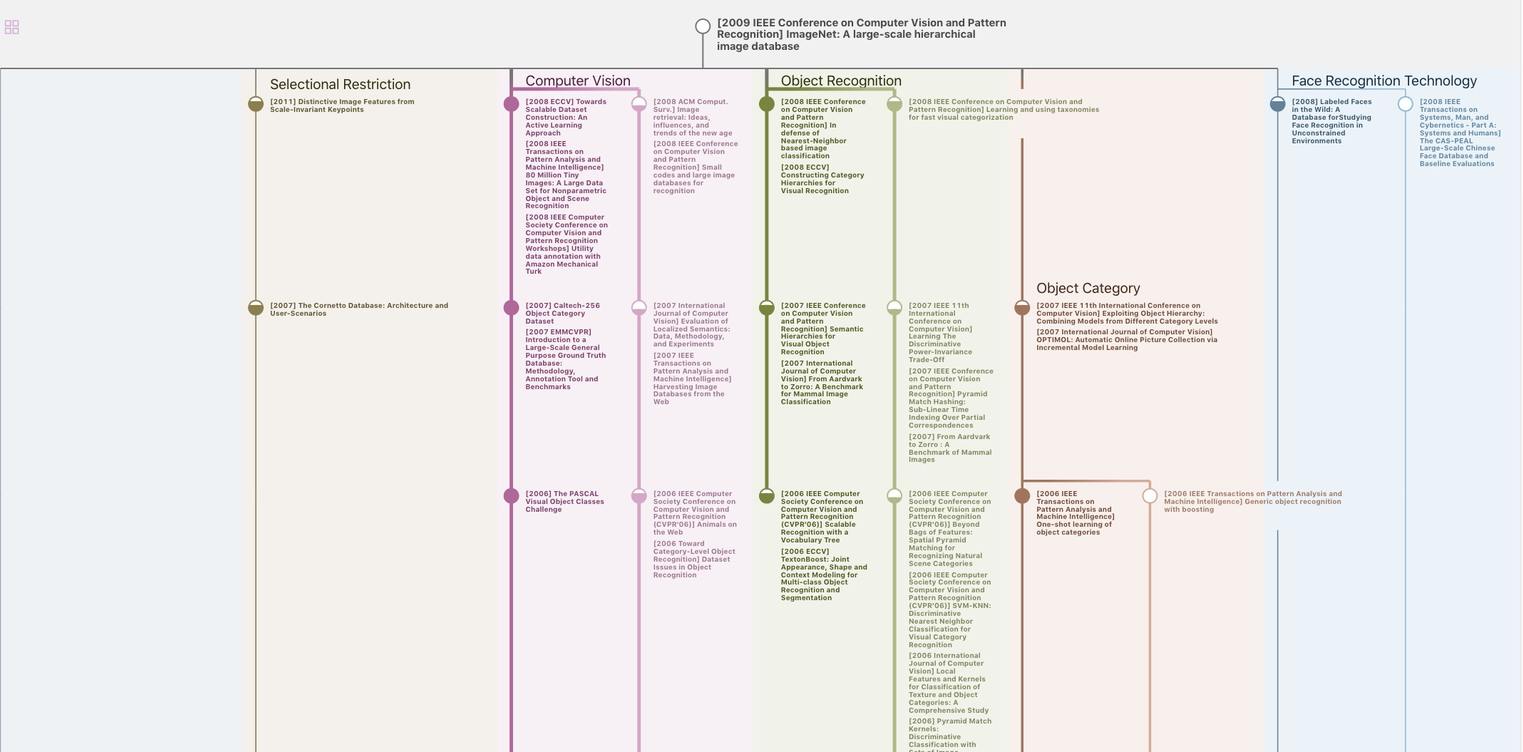
生成溯源树,研究论文发展脉络
Chat Paper
正在生成论文摘要