Rates of Convergence in a Class of Native Spaces for Reinforcement Learning and Control
IEEE CONTROL SYSTEMS LETTERS(2024)
摘要
This letter studies convergence rates for some value function approximations that arise in a collection of reproducing kernel Hilbert spaces (RKHS) H(Omega) . By casting an optimal control problem in a specific class of native spaces, strong rates of convergence are derived for the operator equation that enables offline approximations that appear in policy iteration. Explicit upper bounds on error in value function and control law approximations are derived in terms of power function P-H,P-N for the space of finite dimensional approximants H-N in the native space H(Omega) . These bounds exhibit a distinctive geometric nature, refine and build upon some well-known, now classical results concerning the convergence of approximations of value functions.
更多查看译文
关键词
Kernel,Convergence,Aerospace electronics,Optimal control,Approximation error,Reinforcement learning,Symmetric matrices,optimal control,reproducing kernel
AI 理解论文
溯源树
样例
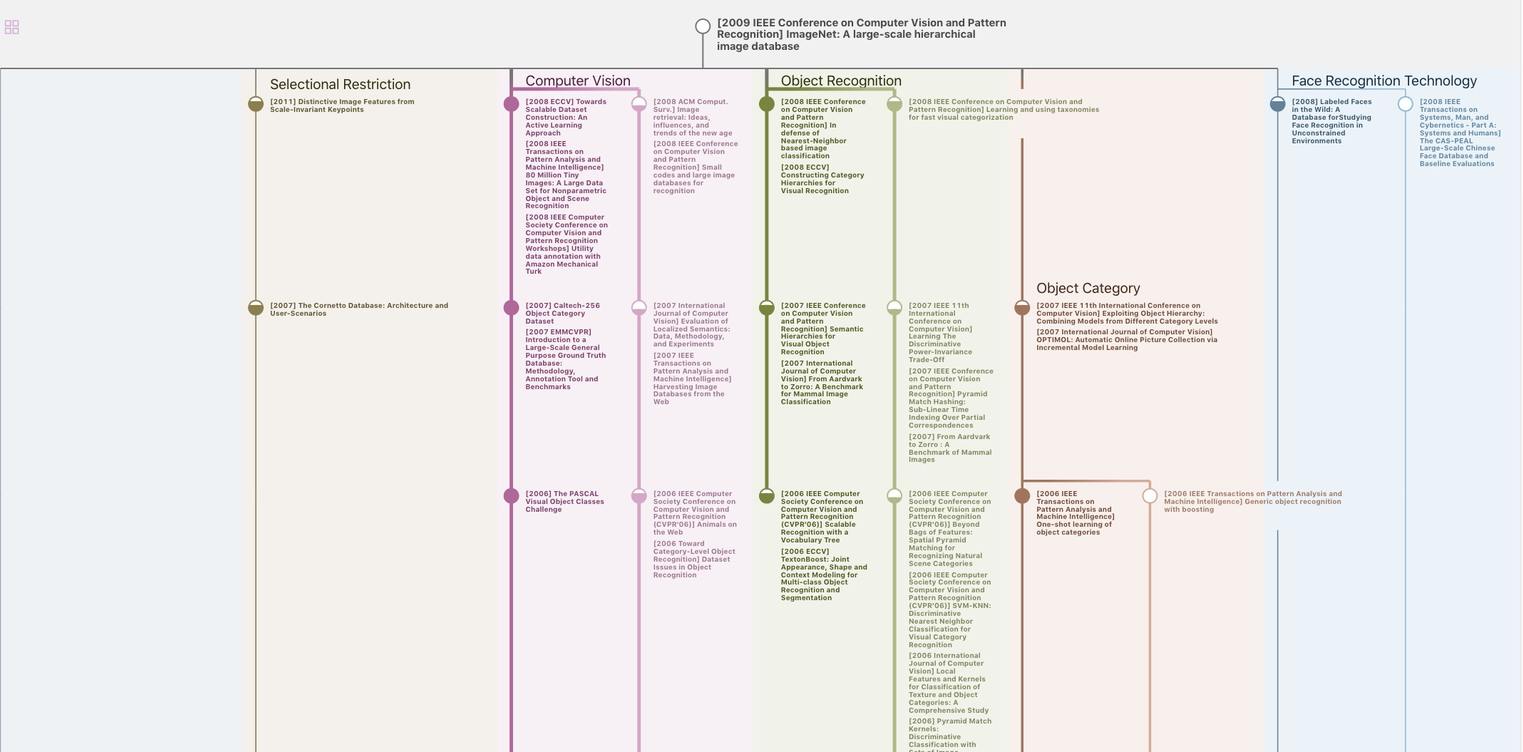
生成溯源树,研究论文发展脉络
Chat Paper
正在生成论文摘要