Deep Guided Attention Network for Joint Denoising and Demosaicing in Real Image
CHINESE JOURNAL OF ELECTRONICS(2024)
摘要
Denoising (DN) and demosaicing (DM) are the first crucial stages in the image signal processing pipeline. Recently, researches pay more attention to solve DN and DM in a joint manner, which is an extremely undetermined inverse problem. Existing deep learning methods learn the desired prior on synthetic dataset, which limits the generalization of learned network to the real world data. Moreover, existing methods mainly focus on the raw data property of high green information sampling rate for DM, but occasionally exploit the high intensity and signal-to-noise (SNR) of green channel. In this work, a deep guided attention network (DGAN) is presented for real image joint DN and DM (JDD), which considers both high SNR and high sampling rate of green information for DN and DM, respectively. To ease the training and fully exploit the data property of green channel, we first train DN and DM sub-networks sequentially and then learn them jointly, which can alleviate the error accumulation. Besides, in order to support the real image JDD, we collect paired raw clean RGB and noisy mosaic images to conduct a realistic dataset. The experimental results on real JDD dataset show the presented approach performs better than the state-of-the-art methods, in terms of both quantitative metrics and qualitative visualization.
更多查看译文
关键词
Training,Visualization,Inverse problems,Green products,Noise reduction,Pipelines,Signal processing,Image denoising,Image demosaicing,Joint processing,Guided attention,Paired real dataset
AI 理解论文
溯源树
样例
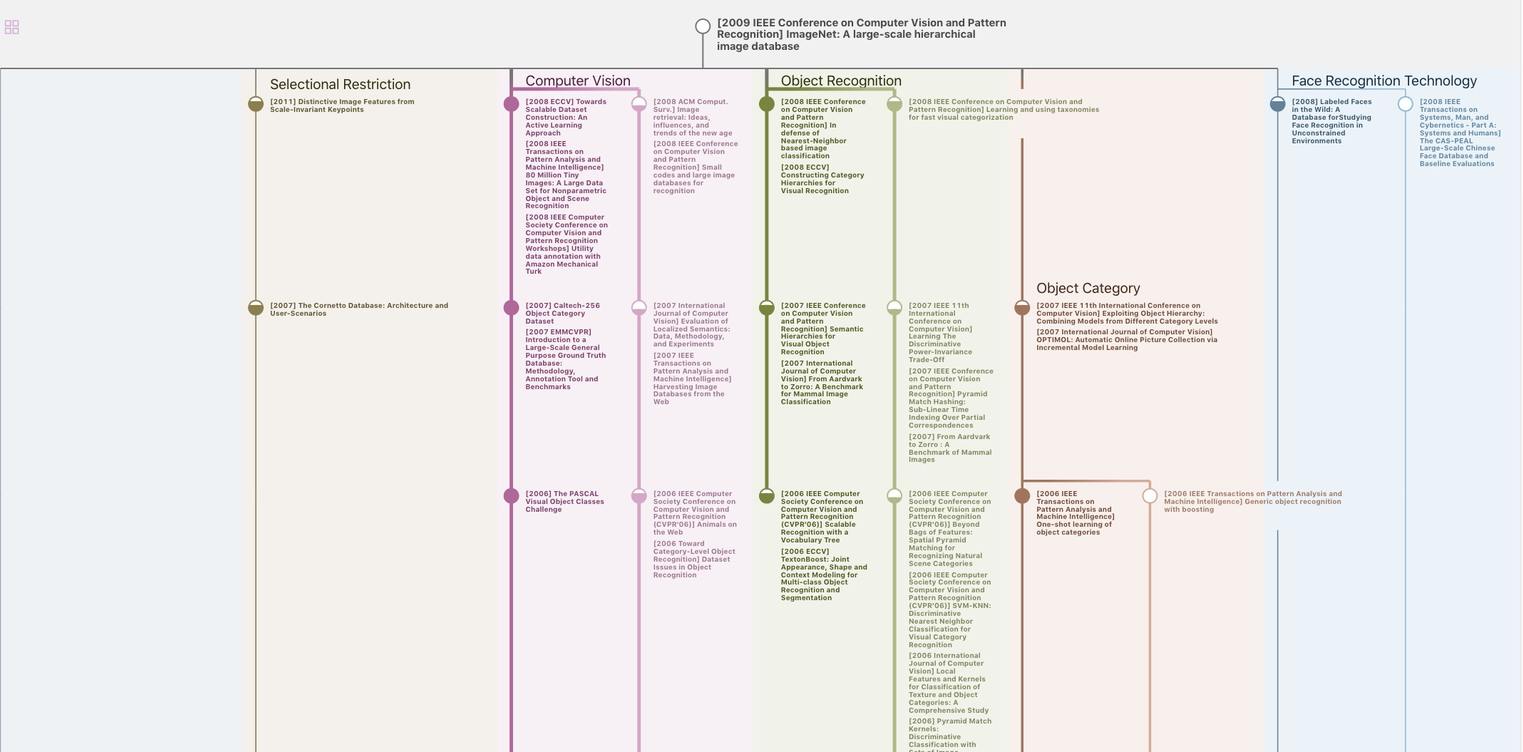
生成溯源树,研究论文发展脉络
Chat Paper
正在生成论文摘要