Enhancing Short-term Electric Load Forecasting with EMD-GA Optimized LSTM
2023 6th International Conference on Power and Energy Applications (ICPEA)(2023)
摘要
To enhance the accuracy of load forecasting, this paper considers both the inherent patterns within historical load data and external influencing factors. A short-term load forecasting approach is proposed that combines Empirical Mode Decomposition (EMD), Genetic Algorithm (GA), and Long Short-Term Memory (LSTM). Inputs include weather conditions, temperature, hourly weights, and date weights, while load data is the output. Historical data from a building in an industrial park in the Wuhan region is utilized as the dataset. Various prediction models are compared, including Back Propagation Neural Network (BP), Support Vector Regression (SVR), Random Forest (RF), and Long Short-Term Memory (LSTM). The evaluation metrics (MAE, RMSE, R
2
) indicate that LSTM outperforms the others. To further enhance the LSTM model, Empirical Mode Decomposition (EMD) is introduced for data preprocessing, and Genetic Algorithm (GA) is employed to optimize LSTM’s hyperparameters. The results show that the EMD-GA-LSTM model achieves the best performance in load forecasting with MAE, RMSE, and R
2
values of 6.18, 7.31, and 0.994, respectively, which are better than the other compared methods.
更多查看译文
关键词
Short-term load forecasting,Genetic algorithm,Long Short-Term Memory network,Empirical Mode Decomposition,Combinatorial optimization
AI 理解论文
溯源树
样例
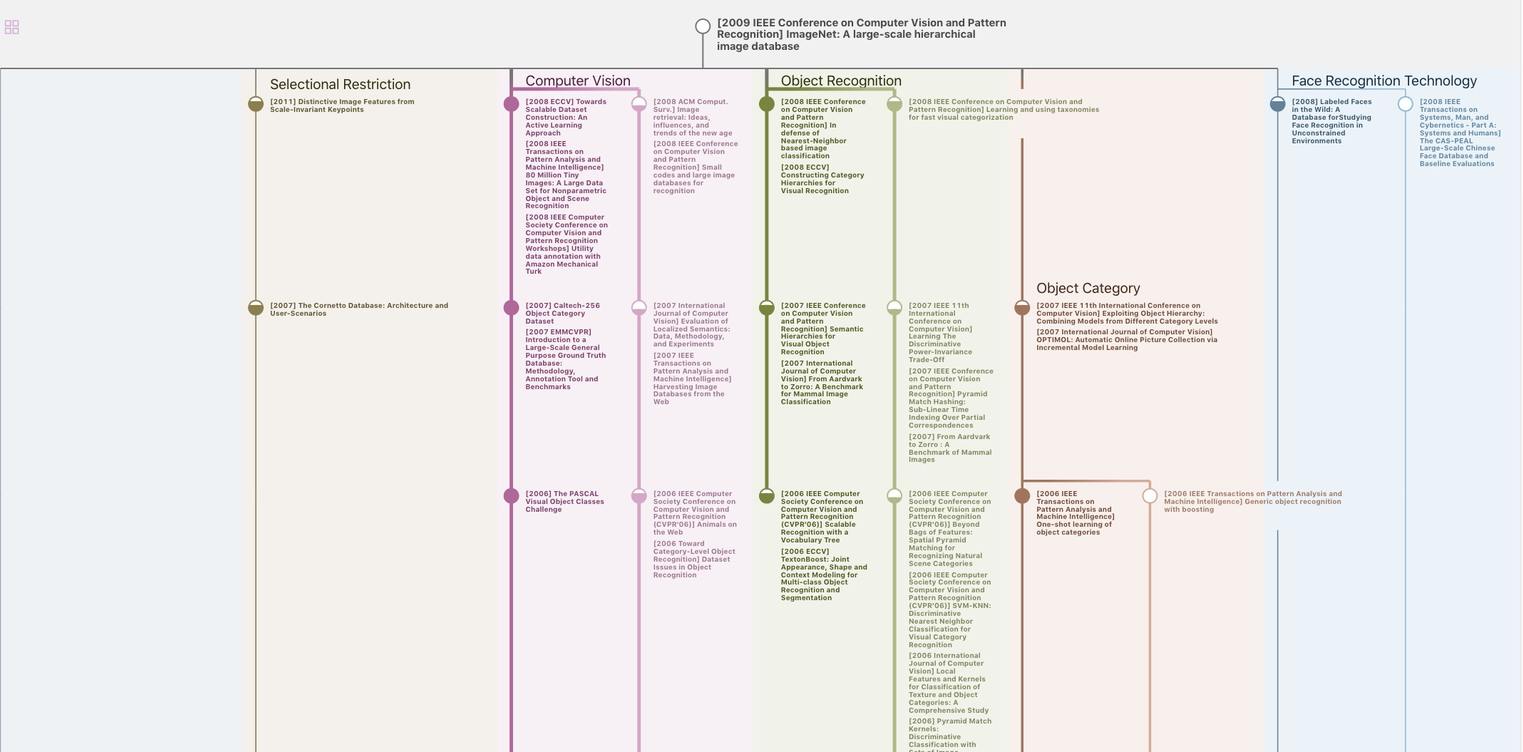
生成溯源树,研究论文发展脉络
Chat Paper
正在生成论文摘要