Learning with Variational Objectives on Measures.
2023 IEEE International Conference on Big Data (BigData)(2023)
摘要
The theory of machine learning has predominantly focused on variational objectives expressed on functions. Yet, the advent of big data has exposed limitations in these formulations: functions struggle to capture uncertainty arising from data veracity issues; and the assumption of data independence often conflicts with data variety. In this vision paper, we propose a shift towards measures as the basis for variational objectives, highlighting their advantages to quantify uncertainty, to generalize out of training distribution, and to deal with scarce annotations. We aim to inspire and stimulate further research in adapting conventional machine learning algorithms to optimize these new objectives, without compromising statistical efficiency.
更多查看译文
关键词
Statistical learning,distribution learning,measure spaces
AI 理解论文
溯源树
样例
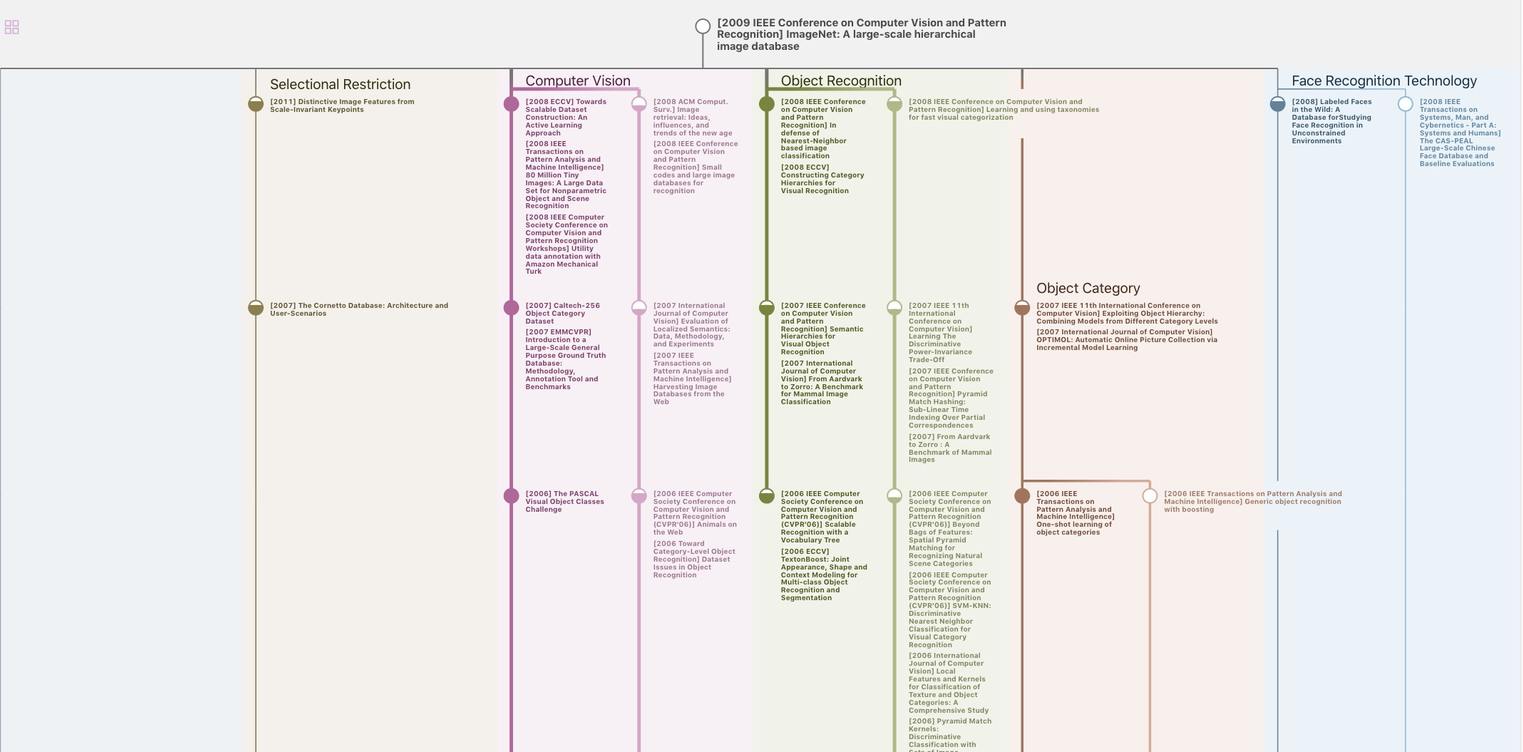
生成溯源树,研究论文发展脉络
Chat Paper
正在生成论文摘要