Achieving Privacy and Tracing Unauthorised Usage: Anonymisation-based Fingerprinting of Private Data.
2023 IEEE International Conference on Big Data (BigData)(2023)
摘要
Since many types of data nowadays contain personally identifiable information about individuals, it is important to apply privacy protection techniques to mitigate disclosure risks. One approach is k-anonymity, where hiding the identity within a group of k similar entities reduces the risk of re-identification. Another risk when distributing data is the loss of control over their further re-distribution and sharing. This risk is frequently addressed by fingerprinting, a method that allows to identify the recipient of a specific copy of the data, by embedding a generally invisible mark.In this paper, we specifically implement and adapt an intrinsic fingerprint scheme that makes use of k-anonymity and the fact that multiple, differently perturbed versions of a dataset can be found that all fulfil a certain k-anonymity, and share a rather similar level of data precision. Thus, these different datasets can be seen each as a fingerprinted version of the original.One research question we address in this paper is the evaluation of the most common generalisation algorithms according to their generalisation strategy and their influence on data utility and the number of resulting release candidates, i.e. fingerprints and execution time. In addition, we investigate the properties and robustness of these fingerprints against intentional (adversarial) manipulation through attack simulations. We further provide recommendations and guidelines on how fingerprinting can be best achieved based on the results of our evaluation.
更多查看译文
关键词
anonymisation,fingerprinting,robustness
AI 理解论文
溯源树
样例
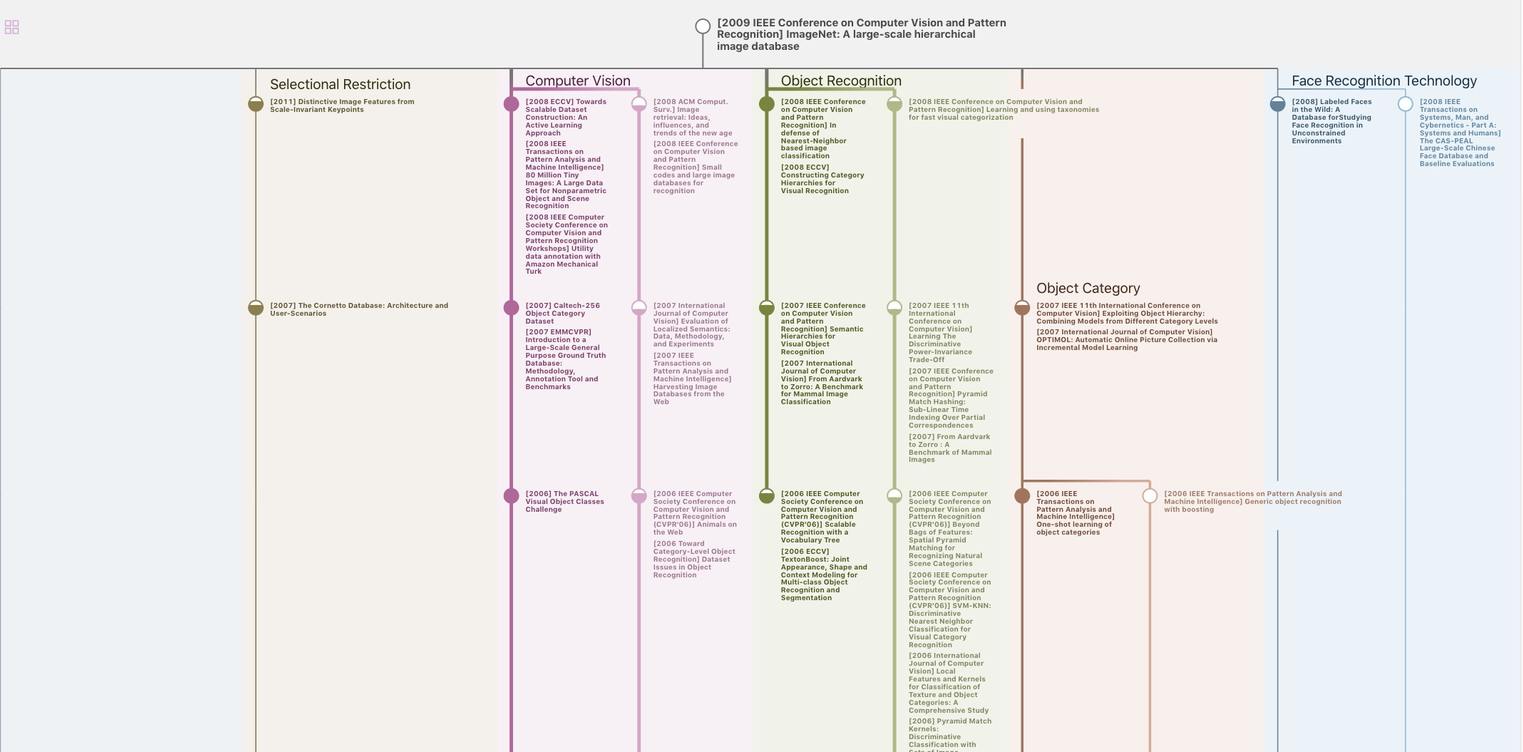
生成溯源树,研究论文发展脉络
Chat Paper
正在生成论文摘要