Subspace Interpolation and Indexing on Stiefel and Grassmann Manifolds as a Lightweight Inference Engine.
2023 IEEE International Conference on Big Data (BigData)(2023)
摘要
Subspace Indexing with Interpolation (SIM-I) on Stiefel and Grassmann manifolds is proposed in this work. Given a partition of some original high-dimensional data set, SIM-I is constructed via two steps: in the first step we build linear affinity-aware subspace models based on each partition; in the second step we interpolate between several adjacent linear subspace models constructed in the first step using the “center of mass” calculation on Stiefel and Grassmann manifolds. Through these two steps, SIM-I builds a globally nonlinear and smoothly regularized low-dimensional embedding model of the original data set. Furthermore, given sufficiently many training samples on the data manifold either labelled by some pre-trained learning model such as Deep Neural Networks (DNNs) or provided with original natural labels, we first apply SIM-I on this data set and then perform nearest-neighbor classification on the resulting low-dimensional embedding. This helps us to build a Lightweight Inference Engine (LIE) carrying similar level of feature extraction by the pre-trained learning model. For DNNs, such LIE can be interpreted as some (nonstandard) shallow neural network with a wide first hidden layer. From this perspective, SIM-I provides a way to exchange deep network for wide but shallow ones and may provide some new insights to interpret DNNs.
更多查看译文
AI 理解论文
溯源树
样例
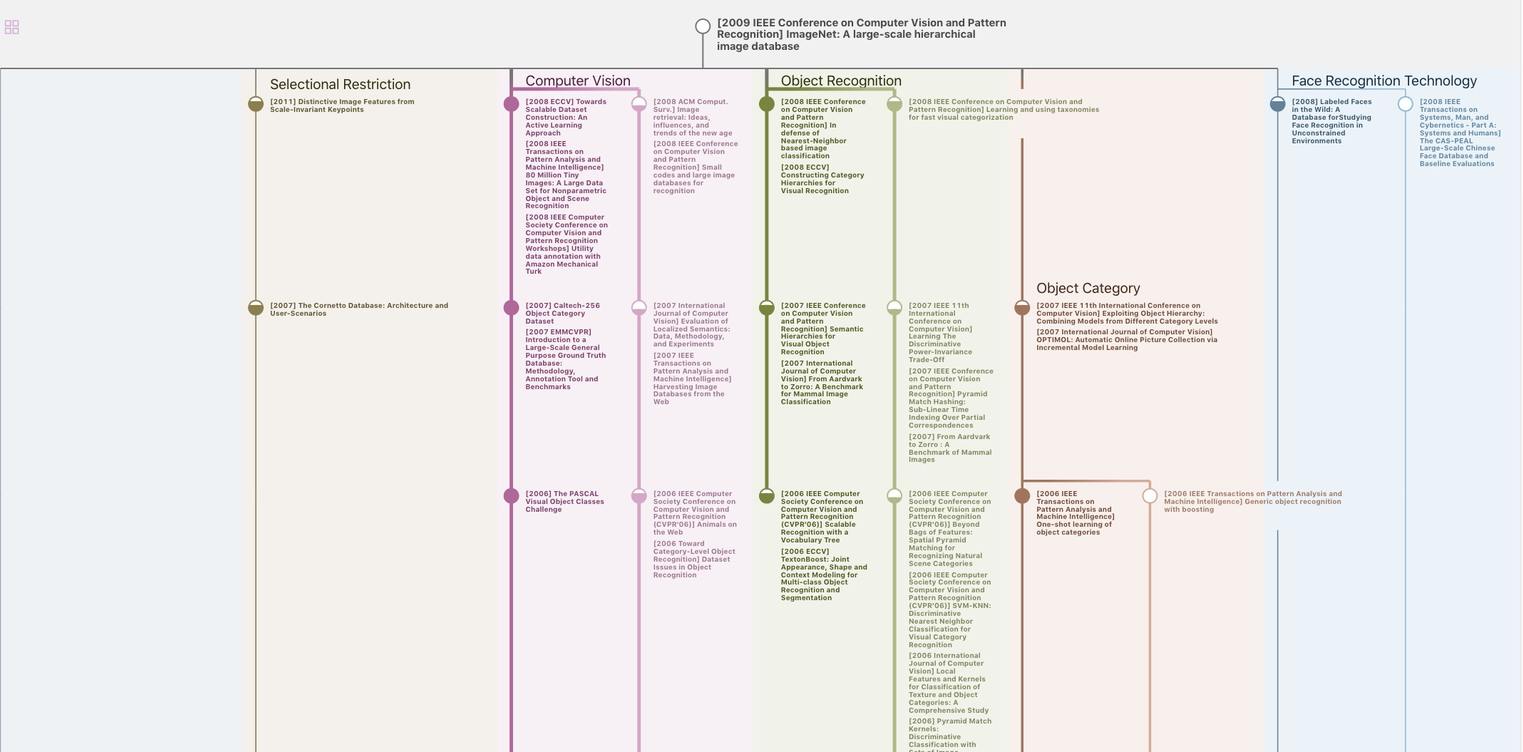
生成溯源树,研究论文发展脉络
Chat Paper
正在生成论文摘要