Feature Selection with Partial Autoencoding for Zero-Sample Fault Diagnosis
IEEE TRANSACTIONS ON INDUSTRIAL INFORMATICS(2024)
摘要
Fault diagnosis is still a challenging task especially for unseen faults, which could happen in the systems. Zero-sample fault diagnosis alleviates this issue by utilizing information from seen faults and attributes defined by domain knowledge. However, existing methods suffer from irrelevant features in the original space while existing supervised feature selection methods yield unsatisfactory performance due to discrepancy caused by domain difference. In this paper, we propose a novel feature selection method for zero-sample fault diagnosis, called concrete partial autoencoder. The concrete partial autoencoder selects features beneficial for both seen and unseen faults through striking a balance between classification accuracy and reconstruction errors of selected features. The concrete partial autoencoder utilizes categorical reparameterization to efficiently solve the feature selection problem. The evaluation results on the Tennessee Eastman Process show that the proposed method improves classification accuracy and robustness against irrelevant features at zero-sample fault diagnosis.
更多查看译文
关键词
Fault diagnosis,feature selection,machine learning,zero-shot learning
AI 理解论文
溯源树
样例
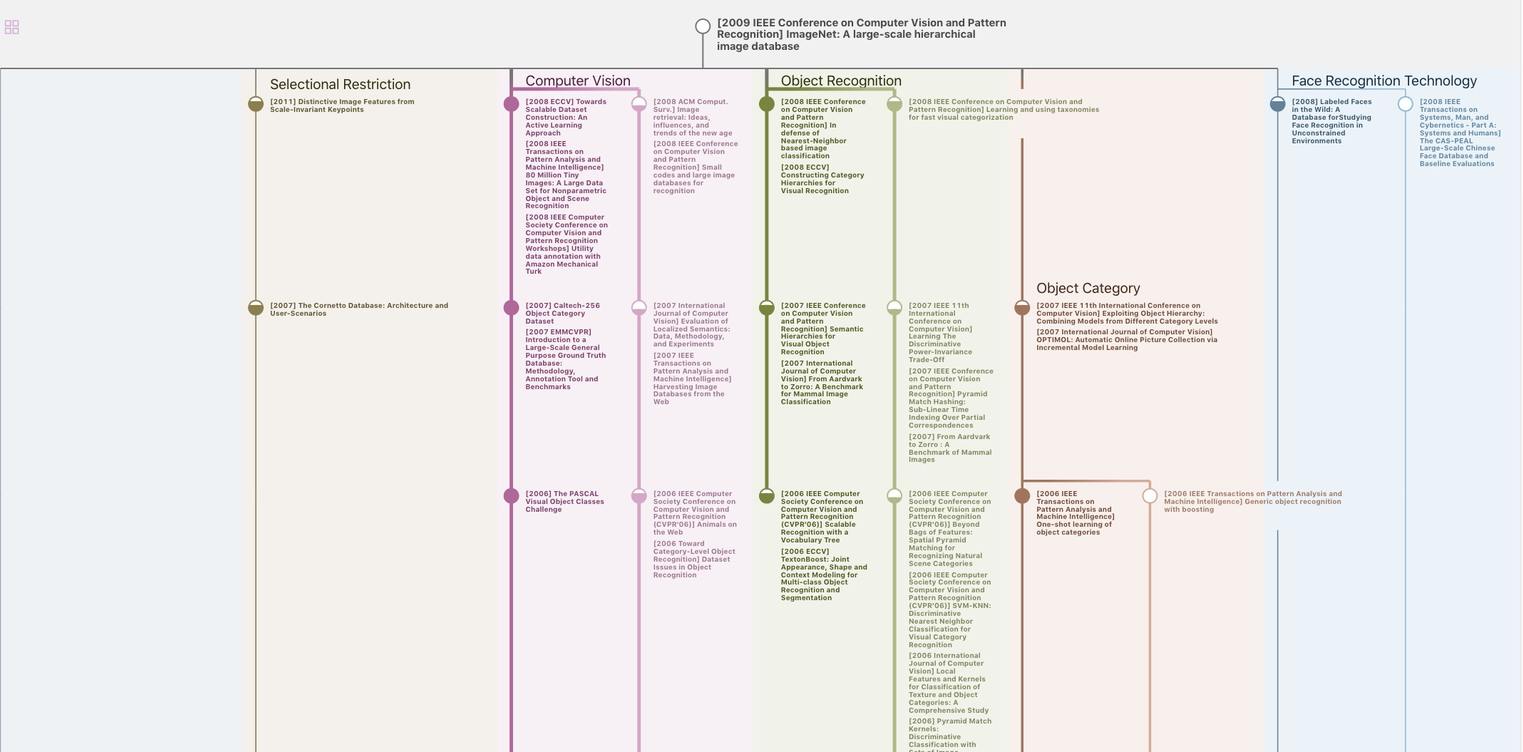
生成溯源树,研究论文发展脉络
Chat Paper
正在生成论文摘要