A lightweight model combining convolutional neural network and Transformer for driver distraction recognition
ENGINEERING APPLICATIONS OF ARTIFICIAL INTELLIGENCE(2024)
摘要
Driver distraction recognition has been studied by many researchers. However, most studies have failed to balance the efficiency and accuracy of models. In this study, a lightweight network called CaTNet is proposed. The CaTNet is a simplified framework based on the existing model ConvNeXt, which prunes redundant feature layers. And a whole new module CaT containing self -attention is introduced in tandem, both of which are combined to enhance the feature characterization. It captures long-range dependencies and retains the local inductive bias provided by the convolutional neural network (CNN). The proposed method is verified on the dataset of American University in Cairo (AUC) and the State Farm Distracted Driver Detection (SFD3) Dataset. The CaTNet achieves 94.82% and 99.91% Top -1 accuracy while the number of model parameters is only 2.832M, with 12.44 frames per second (FPS) running on Jetson Nano. These results are superior to other existing models.
更多查看译文
关键词
Driver distraction recognition,Lightweight network,Convolutional neural network,Transformer
AI 理解论文
溯源树
样例
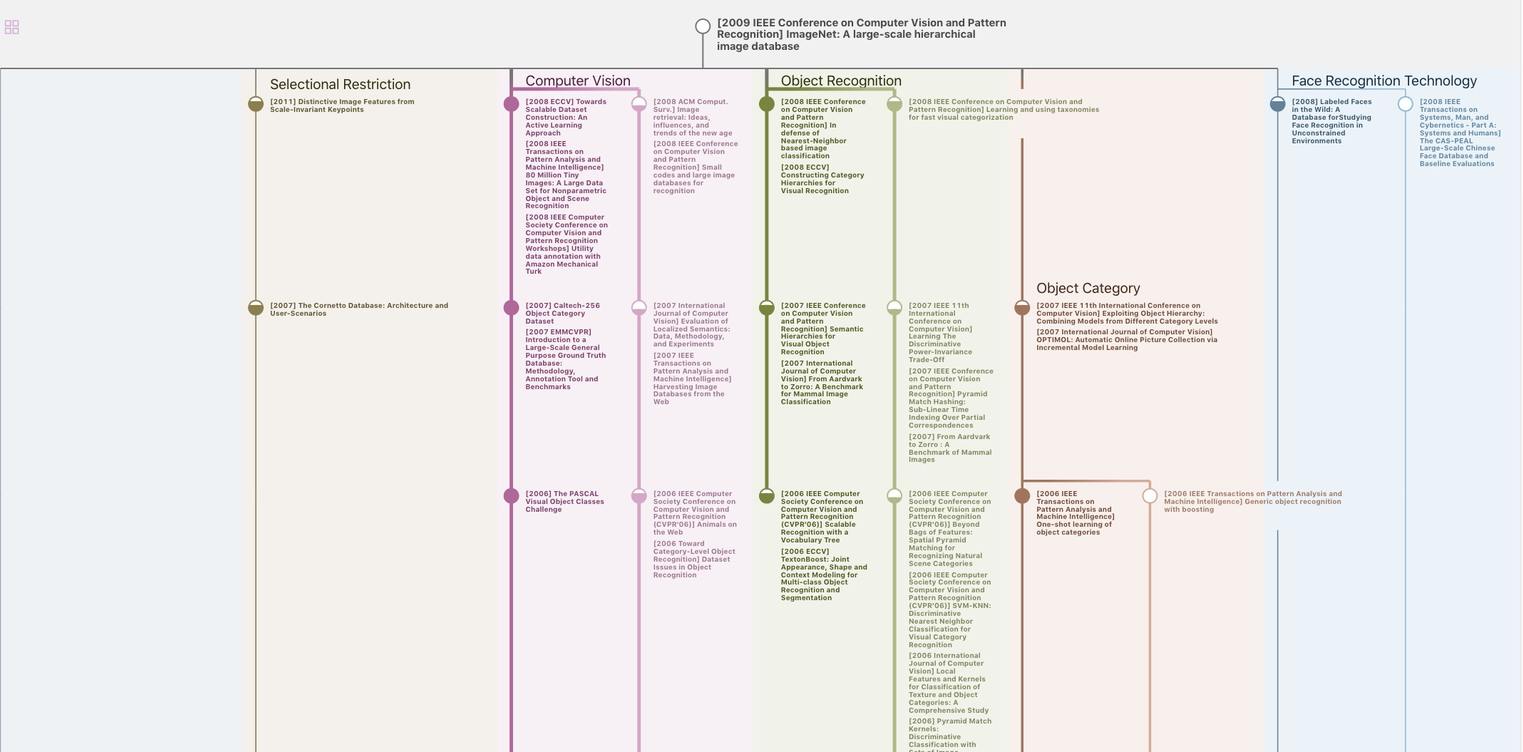
生成溯源树,研究论文发展脉络
Chat Paper
正在生成论文摘要