DDViT: Advancing lithology identification on FMI image logs through a dual modal transformer model with less information drop
GEOENERGY SCIENCE AND ENGINEERING(2024)
摘要
Lithology is an essential topic in oil and gas reservoir studies. Lithological observation lays the foundation for assessing oil and gas prospects and guides future exploration and development. Currently, the prevailing approach in lithological observation heavily depends on the manual analysis of core samples. However, such an approach is highly subjective and time-consuming. With the development of deep learning, some automated deep learning-based methods have been proposed for lithology interpretation from logging curves. However, the Fullbore Formation MicroImager (FMI) image logging, while widely used in the oil field exploration and development process, is occasionally deployed and utilized for lithology identification. In this work, we proposed a Dual-modal Drop-less-information Vision Transformer (DDViT), an FMI image lithology identification model based on transformer architecture. DDViT uses a dual-modal architecture to identify lithology using two different image modalities, namely dynamic FMI images (FMI_DYN) and static FMI images (FMI_STAT). These modalities reflect the local and overall characteristics, respectively. Furthermore, DDViT uses a less-information dropping module to drop the blank band information inherent in the FMI images to make our model more rational and stable. DDViT achieved a 90.81% lithology identification accuracy on Fengxi Well A of the western Qaidam Basin, providing a new approach to lithology identification and demonstrating the great potential of deep learning in geological images.
更多查看译文
关键词
Lithology identification,FMI images,Dual modal,Drop less information
AI 理解论文
溯源树
样例
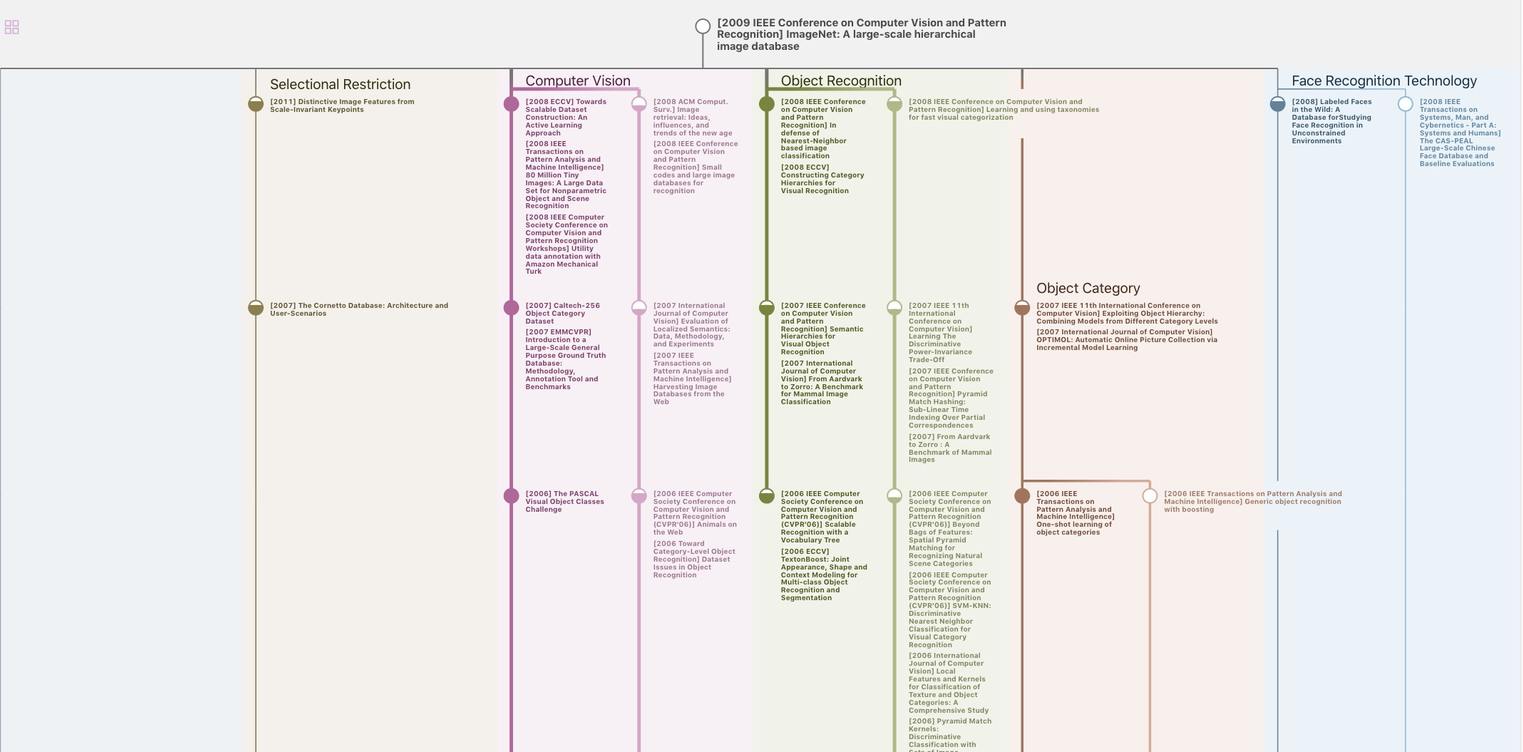
生成溯源树,研究论文发展脉络
Chat Paper
正在生成论文摘要