Prediction of Body Weight by Using PCA-Supported Gradient Boosting and Random Forest Algorithms in Water Buffaloes (Bubalus bubalis) Reared in South-Eastern Mexico
ANIMALS(2024)
摘要
Accurately estimating body weight is crucial for managing water buffalo health and optimizing feeding strategies. This study explored the effectiveness of machine learning models in predicting body weight based on body measurements. Principal component analysis was employed to reduce the dimensionality of the data and identify the most relevant features. Subsequently, Gradient Boosting and Random Forest algorithms were utilized to predict body weight using the reduced data set. The Gradient Boosting algorithm demonstrated superior performance compared to the Random Forest algorithm. These findings suggest that the combination of principal component analysis and Gradient Boosting offers a reliable and effective method for estimating body weight in water buffaloes. This approach holds promise for improving animal production and health management practices. Future research could focus on enhancing the applicability and generalizability of these models to diverse water buffalo populations across various geographical regions. This study aims to use advanced machine learning techniques supported by Principal Component Analysis (PCA) to estimate body weight (BW) in buffalos raised in southeastern Mexico and compare their performance. The first stage of the current study consists of body measurements and the process of determining the most informative variables using PCA, a dimension reduction method. This process reduces the data size by eliminating the complex structure of the model and provides a faster and more effective learning process. As a second stage, two separate prediction models were developed with Gradient Boosting and Random Forest algorithms, using the principal components obtained from the data set reduced by PCA. The performances of both models were compared using R-2, RMSE and MAE metrics, and showed that the Gradient Boosting model achieved a better prediction performance with a higher R-2 value and lower error rates than the Random Forest model. In conclusion, PCA-supported modeling applications can provide more reliable results, and the Gradient Boosting algorithm is superior to Random Forest in this context. The current study demonstrates the potential use of machine learning approaches in estimating body weight in water buffalos, and will support sustainable animal husbandry by contributing to decision making processes in the field of animal science.
更多查看译文
关键词
principal component analysis,gradient boosting,random forest,buffalo,body weight
AI 理解论文
溯源树
样例
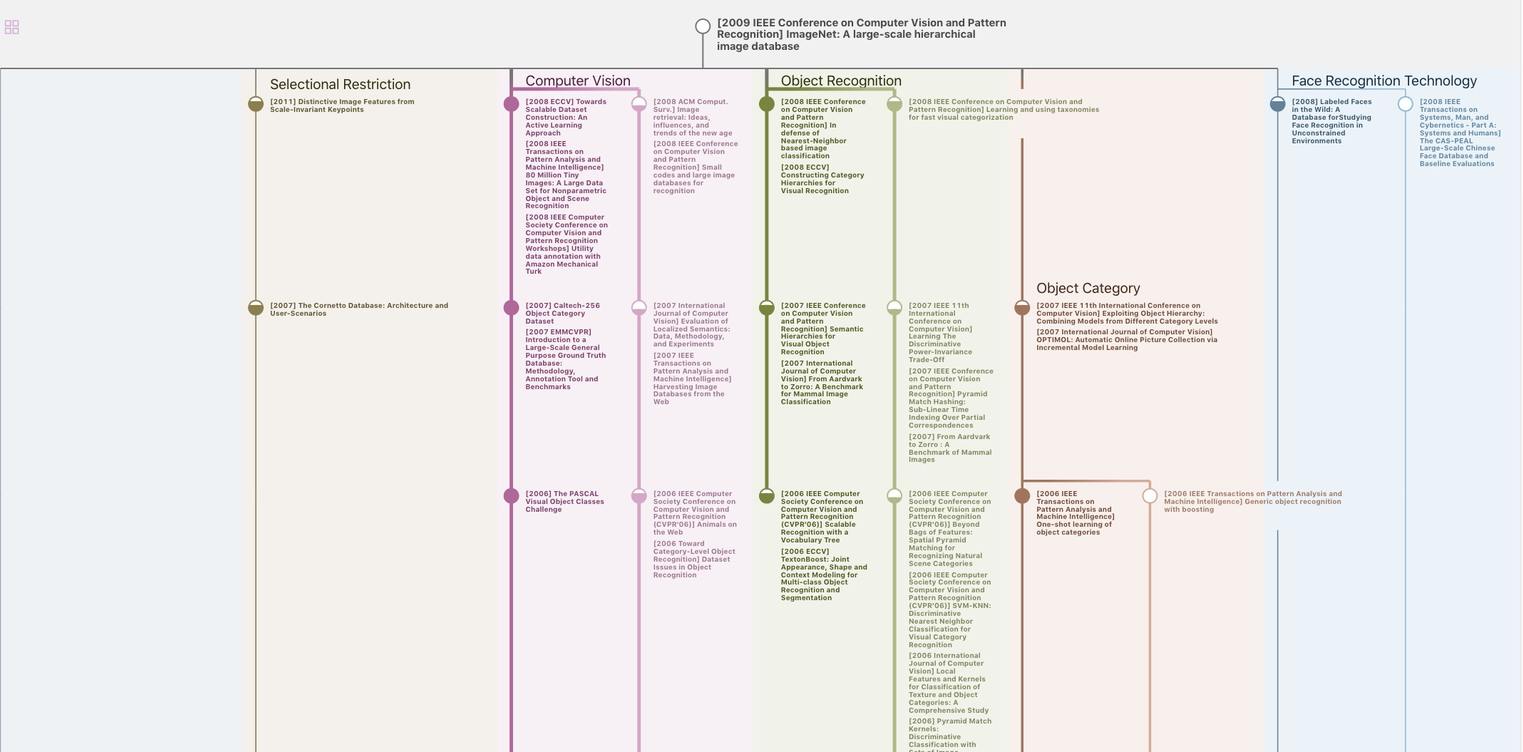
生成溯源树,研究论文发展脉络
Chat Paper
正在生成论文摘要