Segmentation of 71 Anatomical Structures Necessary for the Evaluation of Guideline-Conforming Clinical Target Volumes in Head and Neck Cancers
CANCERS(2024)
摘要
Simple Summary In radiation therapy, accurately contouring the volume containing cancerous cells is crucial for effective tumor control. Experts defined this volume by its borders with respect to anatomical structures. This study assesses the feasibility and precision of a deep-learning method in extracting 71 necessary anatomical structures from provided computed tomography scans. For most of these structures, automatically generated outlines are presented for the first time. For other structures, our model improves upon previously reported results. We analyzed the constraints posed by errors in the automatically generated outlines and found none that are relevant to our goal of identifying the entire volume containing cancerous cells. Our research contributes additional and enhanced outlines of anatomical structures, advancing the scientific objective of facilitating the contouring of a human's complete anatomy. Moreover, confirming the effectiveness of automatic contouring techniques signifies a step closer to achieving precise automated contouring of the cancerous volume.Abstract The delineation of the clinical target volumes (CTVs) for radiation therapy is time-consuming, requires intensive training and shows high inter-observer variability. Supervised deep-learning methods depend heavily on consistent training data; thus, State-of-the-Art research focuses on making CTV labels more homogeneous and strictly bounding them to current standards. International consensus expert guidelines standardize CTV delineation by conditioning the extension of the clinical target volume on the surrounding anatomical structures. Training strategies that directly follow the construction rules given in the expert guidelines or the possibility of quantifying the conformance of manually drawn contours to the guidelines are still missing. Seventy-one anatomical structures that are relevant to CTV delineation in head- and neck-cancer patients, according to the expert guidelines, were segmented on 104 computed tomography scans, to assess the possibility of automating their segmentation by State-of-the-Art deep learning methods. All 71 anatomical structures were subdivided into three subsets of non-overlapping structures, and a 3D nnU-Net model with five-fold cross-validation was trained for each subset, to automatically segment the structures on planning computed tomography scans. We report the DICE, Hausdorff distance and surface DICE for 71 + 5 anatomical structures, for most of which no previous segmentation accuracies have been reported. For those structures for which prediction values have been reported, our segmentation accuracy matched or exceeded the reported values. The predictions from our models were always better than those predicted by the TotalSegmentator. The sDICE with 2 mm margin was larger than 80% for almost all the structures. Individual structures with decreased segmentation accuracy are analyzed and discussed with respect to their impact on the CTV delineation following the expert guidelines. No deviation is expected to affect the rule-based automation of the CTV delineation.
更多查看译文
关键词
automatic segmentation,anatomical structures,multi-label segmentation,clinical target volume delineation,lymph-node-level segmentation,expert guidelines,head and neck cancer
AI 理解论文
溯源树
样例
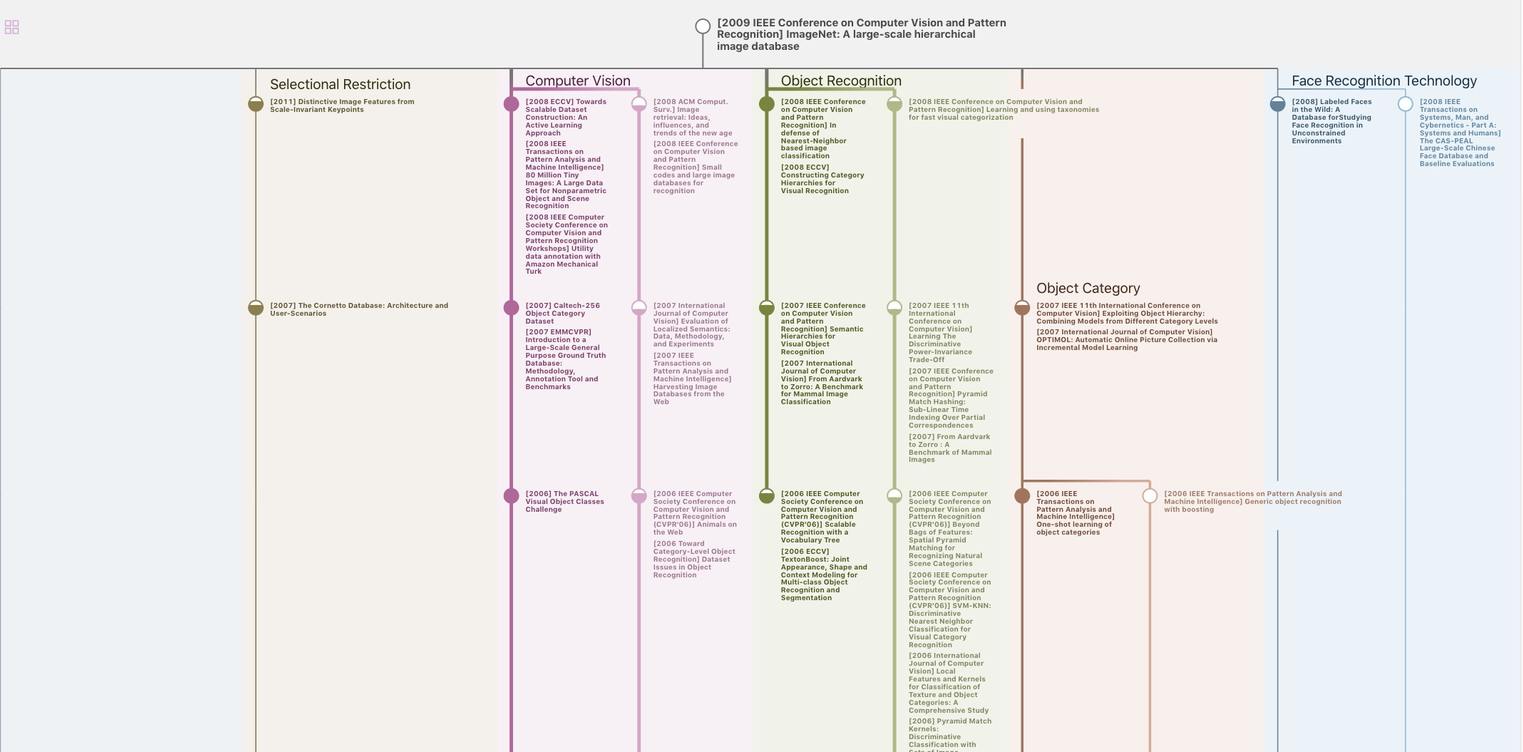
生成溯源树,研究论文发展脉络
Chat Paper
正在生成论文摘要