PVConvNet: Pixel-Voxel Sparse Convolution for multimodal 3D object detection
PATTERN RECOGNITION(2024)
摘要
Current LiDAR-only 3D detection methods inevitably suffer from the sparsity of point clouds and insufficient semantic information. To alleviate this difficulty, recent proposals densify LiDAR points by depth completion and then perform feature fusion with image pixels at the data -level or result -level. However, these methods often suffer from poor fusion effects and insufficient use of image information for voxel feature -level fusion. Meanwhile, noises brought by inaccurate depth completion significantly degrade detection accuracy. In this paper, we propose PVConvNet, a unified framework for multi -modal feature fusion that cleverly combines LiDAR points, virtual points and image pixels. Firstly, we develop an efficient Pixel-Voxel Sparse Convolution (PVConv) to perform voxel-wise feature -level fusion of point clouds and images. Secondly, we design a Noise -Resistant Dilated Sparse Convolution (NRDConv) to encode the voxel features of virtual points, which effectively reduces the impact of noise. Finally, we propose a unified RoI pooling strategy, namely Multimodal Voxel-RoI Pooling, for improving proposal refinement accuracy. We evaluate PVConvNet on the widely used KITTI dataset and the more challenging nuScenes dataset. Experimental results show that our method outperforms state-of-the-art multi -modal based methods, achieving a moderate 3D AP of 86.92% on the KITTI test set.
更多查看译文
关键词
3D object detection,LiDAR points,Virtual points,Image pixels,Multi-modal fusion
AI 理解论文
溯源树
样例
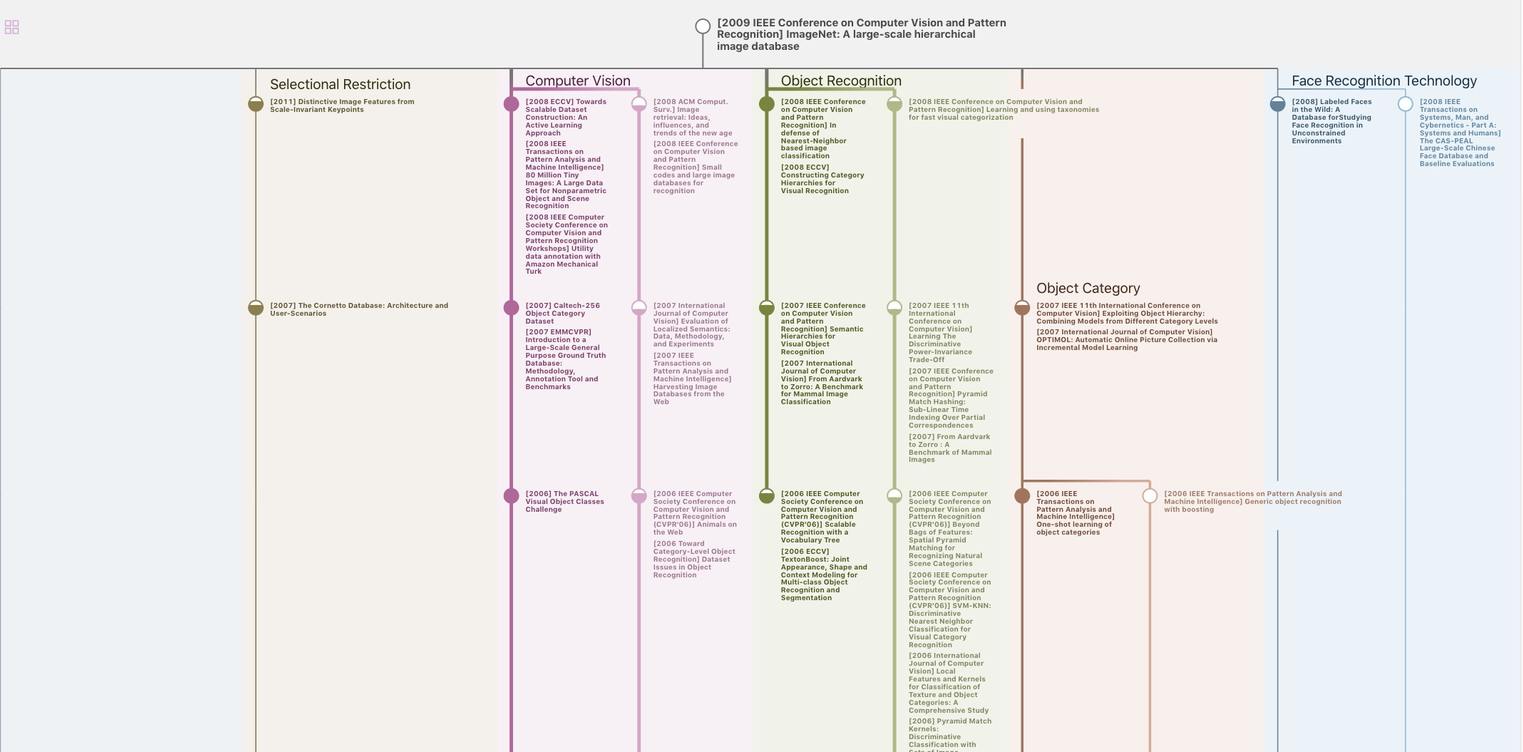
生成溯源树,研究论文发展脉络
Chat Paper
正在生成论文摘要