Source-free domain adaptation with unrestricted source hypothesis
PATTERN RECOGNITION(2024)
摘要
Domain adaptation aims to bridge the distribution discrepancy across different domains and improve the generalization ability of learning models on the target domain. The existing domain adaptation approaches align the distribution shift via adversarial training on the source and target data. In practice, however, the source data is usually unavailable due to the privacy factor. In this work, we mainly focus on the source -free domain adaptation setting, in which we are only accessible to the model trained on the source data and the unlabeled target data. To this end, we propose the Source -Free Adversarial Domain Adaptation (SFADA) approach to align the distribution of the target domain data in the absence of source domain data. In particular, we develop an effective metric to measure the domain discrepancy by introducing the proxy data of the source domain. To generate the proxy data, our approach retrieves target data which lie over the intersection of the supports of the source and target domains. We also derive the learning bound of the source -free domain adaptation theoretically and show that our proposed SFADA approach is capable of reducing the bound effectively. Additionally, instead of modifying the source model in previous source -free approaches, our SFADA does not require training the source model with specific restrictions (i.e., normalizing the classifier weight) for practice and privacy -related concerns. State-of-the-art results are achieved for different standard domain adaptation benchmarks. The code can be available from https://github.com/tiggers23/SFADA-main.
更多查看译文
关键词
Domain adaptation,Privacy protection,Transfer learning,Deep learning
AI 理解论文
溯源树
样例
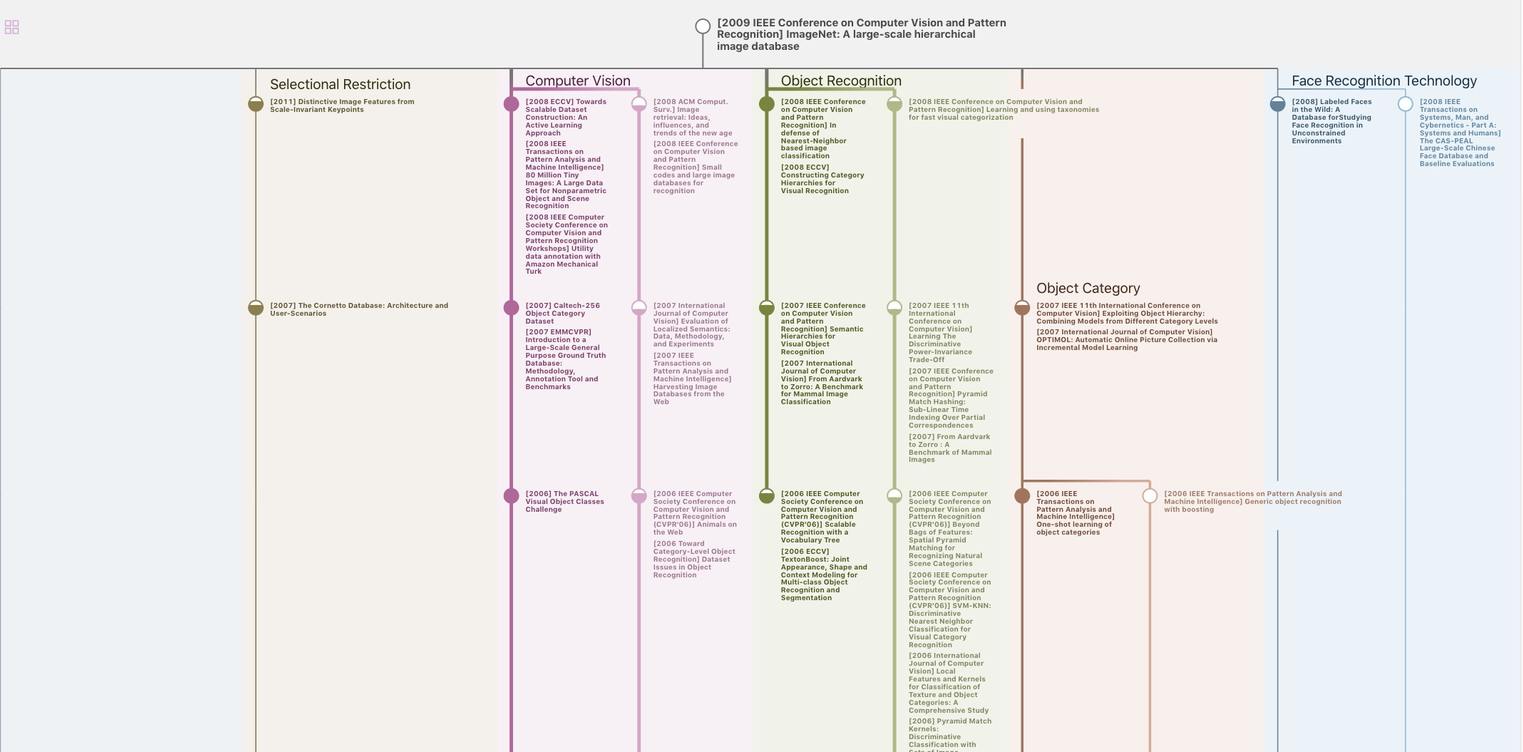
生成溯源树,研究论文发展脉络
Chat Paper
正在生成论文摘要