Monthly NDVI Prediction Using Spatial Autocorrelation and Nonlocal Attention Networks
IEEE JOURNAL OF SELECTED TOPICS IN APPLIED EARTH OBSERVATIONS AND REMOTE SENSING(2024)
摘要
Accurate prediction of vegetation indices is useful for helping maintain vegetation stability, sustaining food production, and reducing socioeconomic losses. The traditional convolutional long short-term memory (ConvLSTM) model for vegetation prediction ignores the spatial aggregation characteristics of the normalized difference vegetation index (NDVI) itself and the global dependence information in space. In this study, we propose a new NDVI prediction method, namely, the ConvLSTM with spatial autocorrelation and nonlocal attention module (ConvLSTM-SAC-NL), by combining the nonlocal attention module to capture long-range dependence and the spatial autocorrelation modeling based on the local Moran index to learn spatial dependence. The experimental results indicate that the ConvLSTM-SAC-NL model outperforms seven baseline forecasting models, with an R${}<^>{2}$ of 0.881 in monthly NDVI prediction in the Huangpi district of Wuhan city, relative to the R${}<^>{2}$ values of 0.758, 0.777, 0.741, 0.776, 0.804, 0.829, and 0.815 for random forest, support vector machine regression, long short-term memory, bidirectional long short-term memory, graph convolutional network, predictive recurrent neural network, and ConvLSTM models, respectively. Spatially, the prediction results of the ConvLSTM-SAC-NL model demonstrate improved accuracy over 91.49$\%$ of the study area when compared with ConvLTSM. Therefore, the proposed ConvLSTM-SAC-NL model could serve as an effective approach for short-term prediction of vegetation conditions at regional scales.
更多查看译文
关键词
Vegetation mapping,Predictive models,Biological system modeling,Forecasting,Autocorrelation,Spatial resolution,Data models,Convolutional long short-term memory (ConvLSTM),nonlocal attention module,normalized difference vegetation index (NDVI),spatial autocorrelation,spatiotemporal prediction
AI 理解论文
溯源树
样例
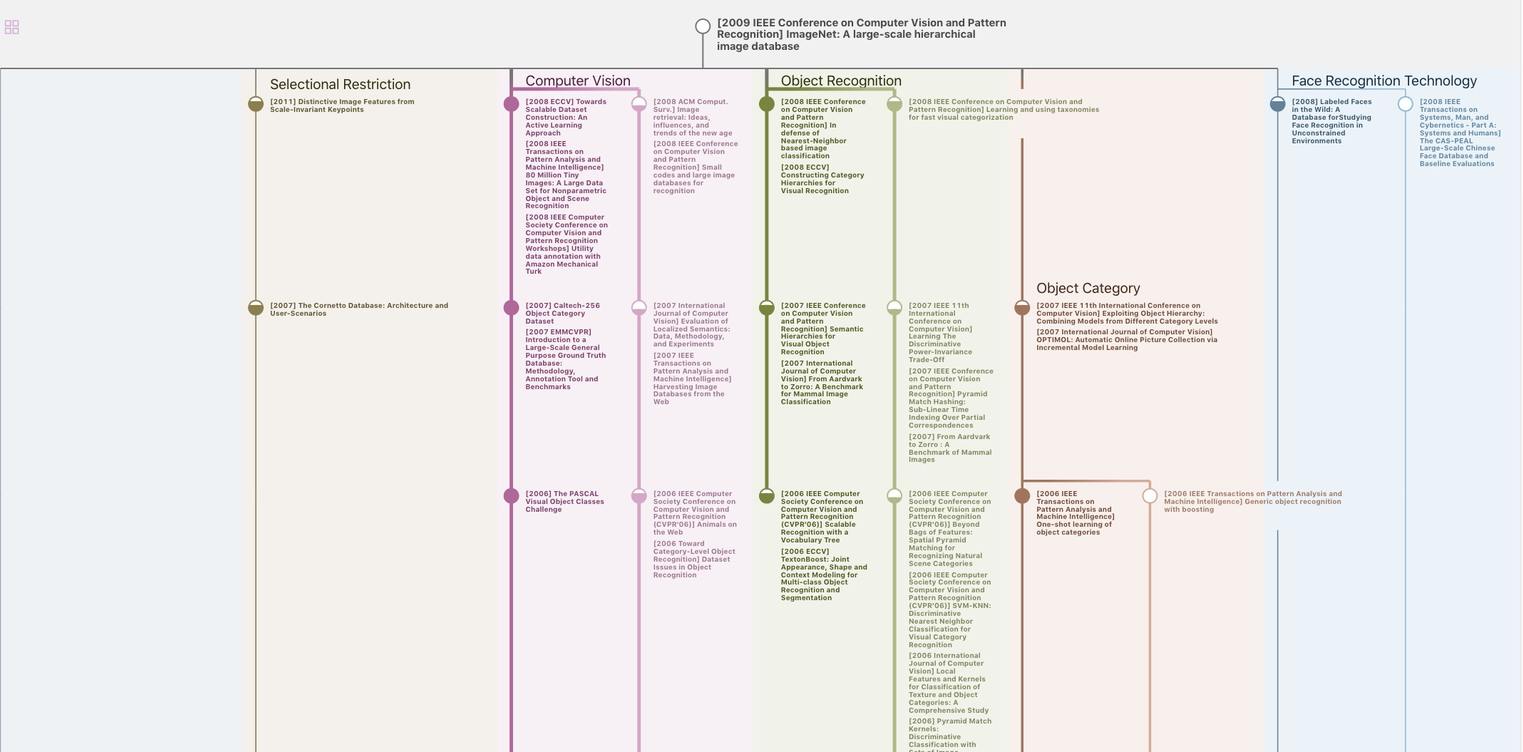
生成溯源树,研究论文发展脉络
Chat Paper
正在生成论文摘要