An FCN-based transfer-learning method for spatial infrared moving-target recognition
INFRARED PHYSICS & TECHNOLOGY(2024)
摘要
Spatial infrared (IR) moving -target sample data are difficult to collect; thus, they cannot be used for masstraining, and limit development and application of deep learning methods in spatial IR target recognition. This study proposes a transfer -learning method based on fully convolutional network (FCN) for spatial IR moving -target recognition. First, we built a set of semi -physical scale models of spatial IR moving targets and obtained IR radiation characteristics as target -domain data using actual shot data and image inversion. Simulation data of the spatial target were obtained using the IR radiation intensity model, and used as the sourcedomain data together with the public UCR dataset. We used the dynamic time warping distance to measure the similarity between datasets to select appropriate source -domain datasets. We used an FCN framework as the main classification framework with a large number of source -domain samples for training. Knowledge transfer and model fine-tuning facilitate target -domain learning using only a few samples to improve classification accuracy. The experimental results show that the proposed model achieved an accuracy rate of 78%, which increases by the 20% improvement compared to direct training on the target domain. This study verifies the effectiveness of transfer learning between spatial targets and related domains and provides a reference for application of deep learning in spatial situational awareness.
更多查看译文
关键词
Spatial target recognition,Transfer learning,Infrared radiation intensity,Semi -physical scale model,Fully convolutional network
AI 理解论文
溯源树
样例
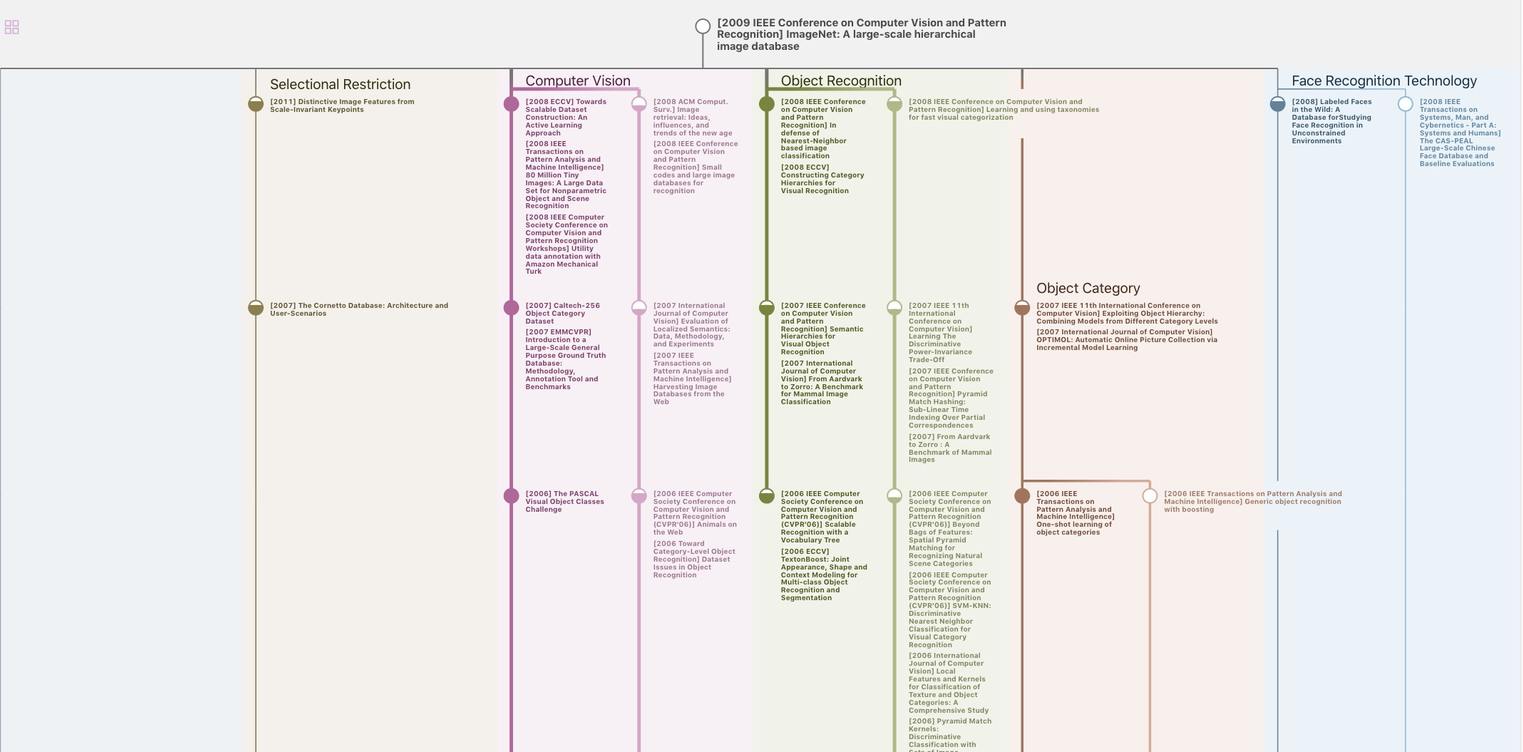
生成溯源树,研究论文发展脉络
Chat Paper
正在生成论文摘要