Truncated Cauchy random perturbations for smoothed functional-based stochastic optimization
AUTOMATICA(2024)
摘要
In this paper, we present a stochastic gradient algorithm for minimizing a smooth objective function that is an expectation over noisy cost samples and only the latter are observed for any given parameter. Our algorithm employs a gradient estimation scheme with random perturbations obtained from the truncated Cauchy distribution. We analyze the bias and variance of the proposed gradient estimator. Our algorithm is found to be particularly useful in the case when the objective function is non -convex and the parameter dimension is high. From an asymptotic convergence analysis, we establish that our algorithm converges almost surely to the set of stationary points of the objective function. Further, the asymptotic convergence rate of our algorithm is better than Gaussian smoothed functional (GSF) and simultaneous perturbation stochastic approximation (SPSA), which are two popular algorithms that employ random perturbations for gradient estimation. We also show that our algorithm avoids unstable equilibria and thereby converges to local minima. In addition, we establish a non -asymptotic bound for our algorithm toward finding a stationary point of the non -convex objective function. (c) 2024 Elsevier Ltd. All rights reserved.
更多查看译文
关键词
Stochastic non-convex optimization,Smoothed functional (SF) algorithms,Truncated Cauchy perturbations,Zeroth-order gradient estimation
AI 理解论文
溯源树
样例
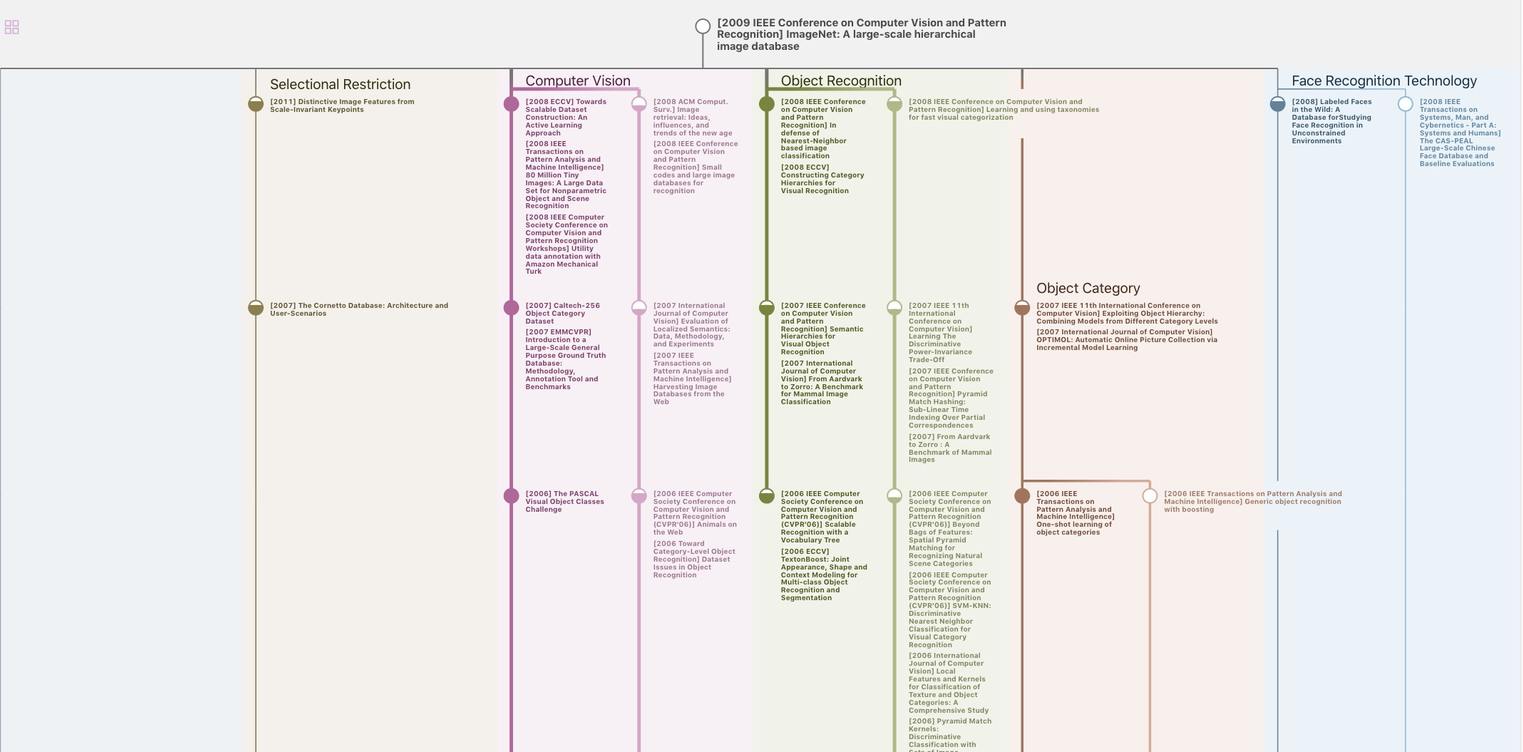
生成溯源树,研究论文发展脉络
Chat Paper
正在生成论文摘要