Assessing the spatial distribution of positional error associated to dense point cloud measurements using regional Gaussian random fields
MEASUREMENT(2024)
摘要
Being able to assess the amount of uncertainty locally associated to dense point clouds generated by measurement can help investigate the relations between the metrological performance of a chosen measuring technology, and the local geometric and surface properties of the measurand geometry. In previous research it was demonstrated that spatial statistics based on Gaussian Random Fields and measurement repeats could be used to obtain spatial maps capturing both local dispersion and local bias associated to the position of points within measured clouds. However, the previous method had scalability limitations when handling very dense point clouds, due to it requiring the resolution of a global, increasingly larger, covariance matrix in order to solve the random field fitting problem. This work presents a variant to the previous method, where the covariance matrix is solved only locally, making the method better scalable to handle denser point clouds. Despite the new method not being able to return an equally rich information content in relation to spatial covariance, it still allows to obtain almost equally accurate information on local bias and variance, with significant gains in terms of processing speed and, importantly, making it now possible to handle very dense clouds which would be unviable to process with the original method.
更多查看译文
关键词
Point positional error,Gaussian random fields,Local covariance,Optical coordinate metrology
AI 理解论文
溯源树
样例
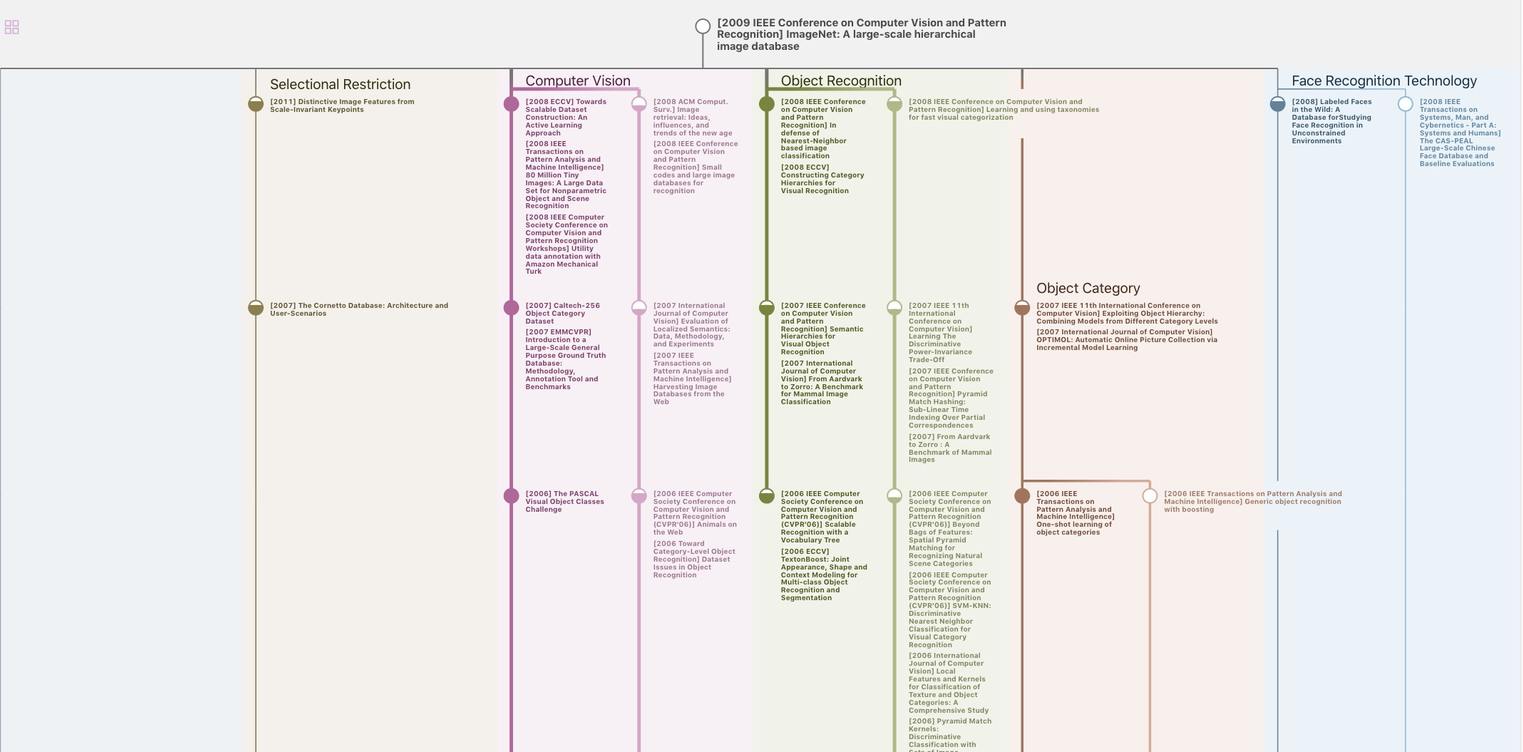
生成溯源树,研究论文发展脉络
Chat Paper
正在生成论文摘要