Raman and photoluminescence signal separation in Raman hyperspectral imagery including noise reduction
JOURNAL OF RAMAN SPECTROSCOPY(2024)
摘要
Raman hyperspectral imaging (RHSI) is a valuable tool for gaining crucial information about the chemical composition of materials. However, obtaining clear Raman signals is not always a trivial task. Raw Raman signals can be susceptible to photoluminescence interference and noise. Hence, the preprocessing of RHSI is a required step for an effective and reliable chemical analysis. The main challenge is splitting the measured RHSI into separate Raman photoluminescence signals. Since no golden-standard exists, it is non-trivial to validate the correctness of the separated signals. While current state-of-the-art preprocessing methods are effective, they require expert knowledge and involve unintuitive hyperparameters. Current approaches also lack generalizability, requiring extensive hyperparameter tuning on a case-by-case basis, while even then results are not always as expected. To this end, this work proposes a novel iterative RHSI preprocessing pipeline for splitting raw Raman signals and noise removal based on linear spline and radial basis function regression (IlsaRBF). The proposed method involves hyperparameters based on the physical properties of Raman spectroscopy, making them intuitive to use. This leads to more robust and stable hyperparameters, reducing the necessity for extensive hyperparameter tuning. A thorough evaluation shows that the proposed method outperforms the current state-of-the-art. Additionally, a cosmic ray identification and removal algorithm (CRIR) and dynamic PCA for noise reduction are introduced. A standalone tool containing our proposed methods is provided, making RHSI preprocessing available to a broader audience, aiding further research and advancements in the field of Raman spectroscopy. Raman hyperspectral imaging is valuable for understanding material chemical composition. However, challenges arise due to photoluminescence interference and noise in raw signals. Current preprocessing methods are effective but demand expert knowledge and lack generalizability. This work introduces an iterative preprocessing pipeline utilizing linear spline and radial basis function regression. Hyperparameters aligned with Raman spectroscopy's physical properties enhance intuitiveness, robustness, and stability. The evaluation demonstrates superiority over existing methods. Additional innovations include a cosmic ray identification and removal algorithm and dynamic PCA for noise reduction. image
更多查看译文
关键词
automated background removal,hyperspectral imaging,Raman spectroscopy,spectral signal processing,unsupervised machine learning
AI 理解论文
溯源树
样例
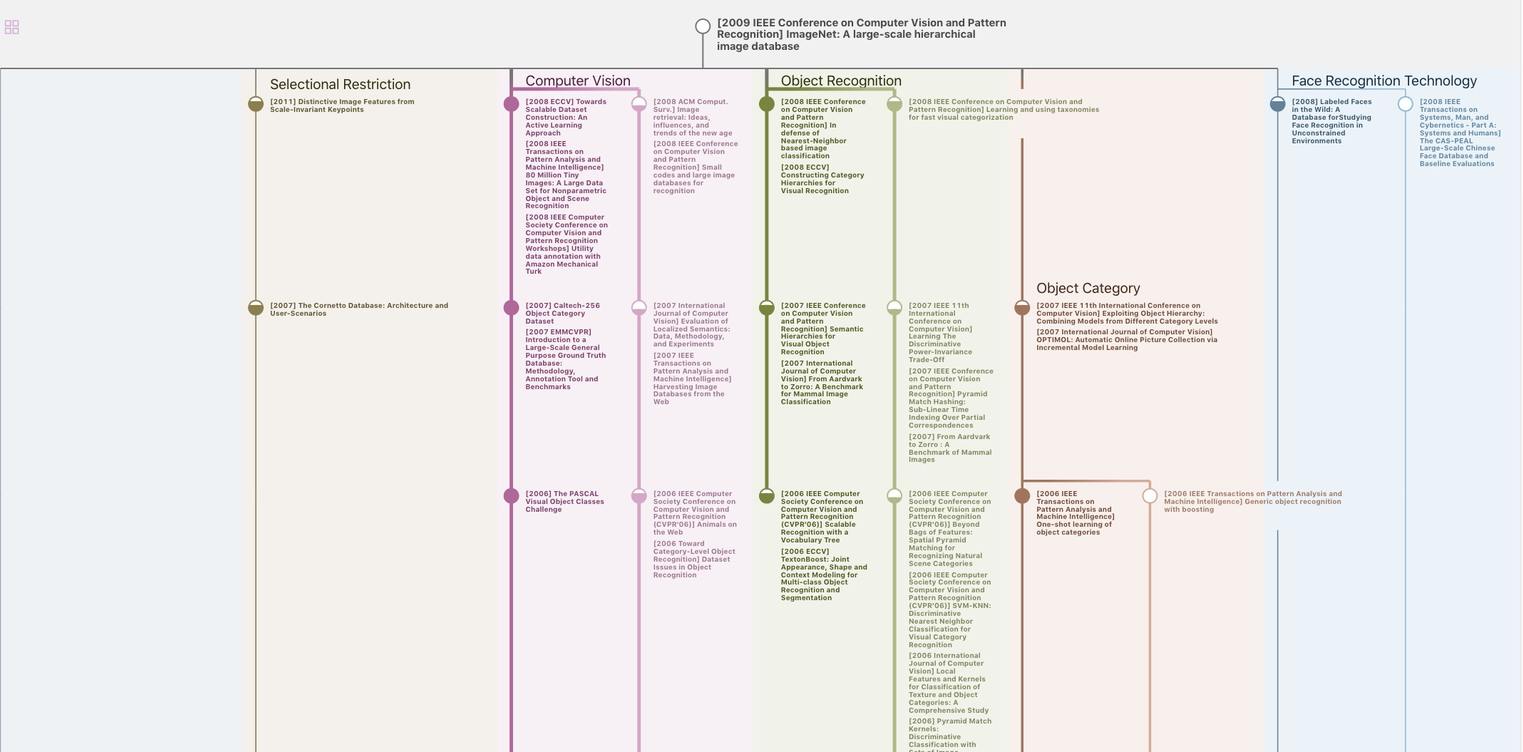
生成溯源树,研究论文发展脉络
Chat Paper
正在生成论文摘要