PointDifformer: Robust Point Cloud Registration With Neural Diffusion and Transformer
IEEE TRANSACTIONS ON GEOSCIENCE AND REMOTE SENSING(2024)
摘要
Point cloud registration is a fundamental technique in 3-D computer vision with applications in graphics, autonomous driving, and robotics. However, registration tasks under challenging conditions, under which noise or perturbations are prevalent, can be difficult. We propose a robust point cloud registration approach that leverages graph neural partial differential equations (PDEs) and heat kernel signatures. Our method first uses graph neural PDE modules to extract high-dimensional features from point clouds by aggregating information from the 3-D point neighborhood, thereby enhancing the robustness of the feature representations. Then, we incorporate heat kernel signatures into an attention mechanism to efficiently obtain corresponding keypoints. Finally, a singular value decomposition (SVD) module with learnable weights is used to predict the transformation between two point clouds. Empirical experiments on a 3-D point cloud dataset demonstrate that our approach not only achieves state-of-the-art performance for point cloud registration but also exhibits better robustness to additive noise or 3-D shape perturbations.
更多查看译文
关键词
Point cloud compression,Three-dimensional displays,Feature extraction,Kernel,Heating systems,Iterative methods,Neural networks,Graph neural network (GNN),heat kernel signature,neural diffusion,point cloud registration
AI 理解论文
溯源树
样例
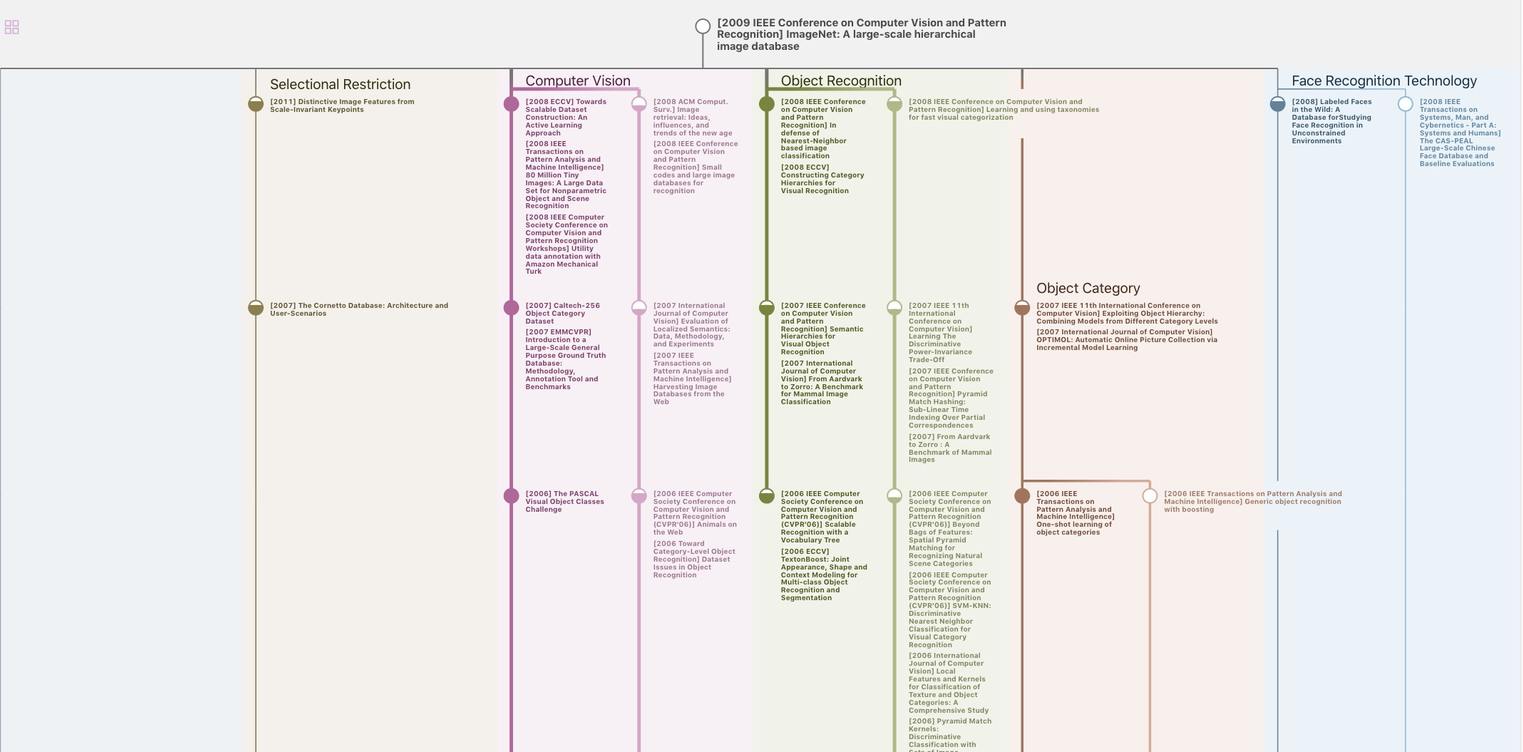
生成溯源树,研究论文发展脉络
Chat Paper
正在生成论文摘要