Speed Planning Based on Terrain-Aware Constraint Reinforcement Learning in Rugged Environments
IEEE ROBOTICS AND AUTOMATION LETTERS(2024)
摘要
Speed planning in rugged terrain poses challenges due to various constraints, such as traverse efficiency, dynamics, safety, and smoothness. This letter introduces a framework based on Constrained Reinforcement Learning (CRL) that considers all these constraints. In addition, extracting the terrain information as a constraint to be added to the CRL is also a barrier. In this letter, a terrain constraint extraction module is designed to quantify the semantic and geometric attributes of the terrain by estimating maximum safe speed. All networks are trained on simulators or datasets and eventually deployed on a real mobile robot. To continuously improve the planning performance and mitigate the error caused by the simulator-reality gap, we propose a feedback structure for detecting and preserving critical experiences during the testing process. The experiments in the simulator and the real robot demonstrate that our method can reduce the frequency of dangerous status by 45% and improve up to 71% smoothness.
更多查看译文
关键词
Planning,Robots,Semantics,Data mining,Neural networks,Reinforcement learning,Mobile robots,Speed planning,mobile robot,rugged environments,reinforcement learning
AI 理解论文
溯源树
样例
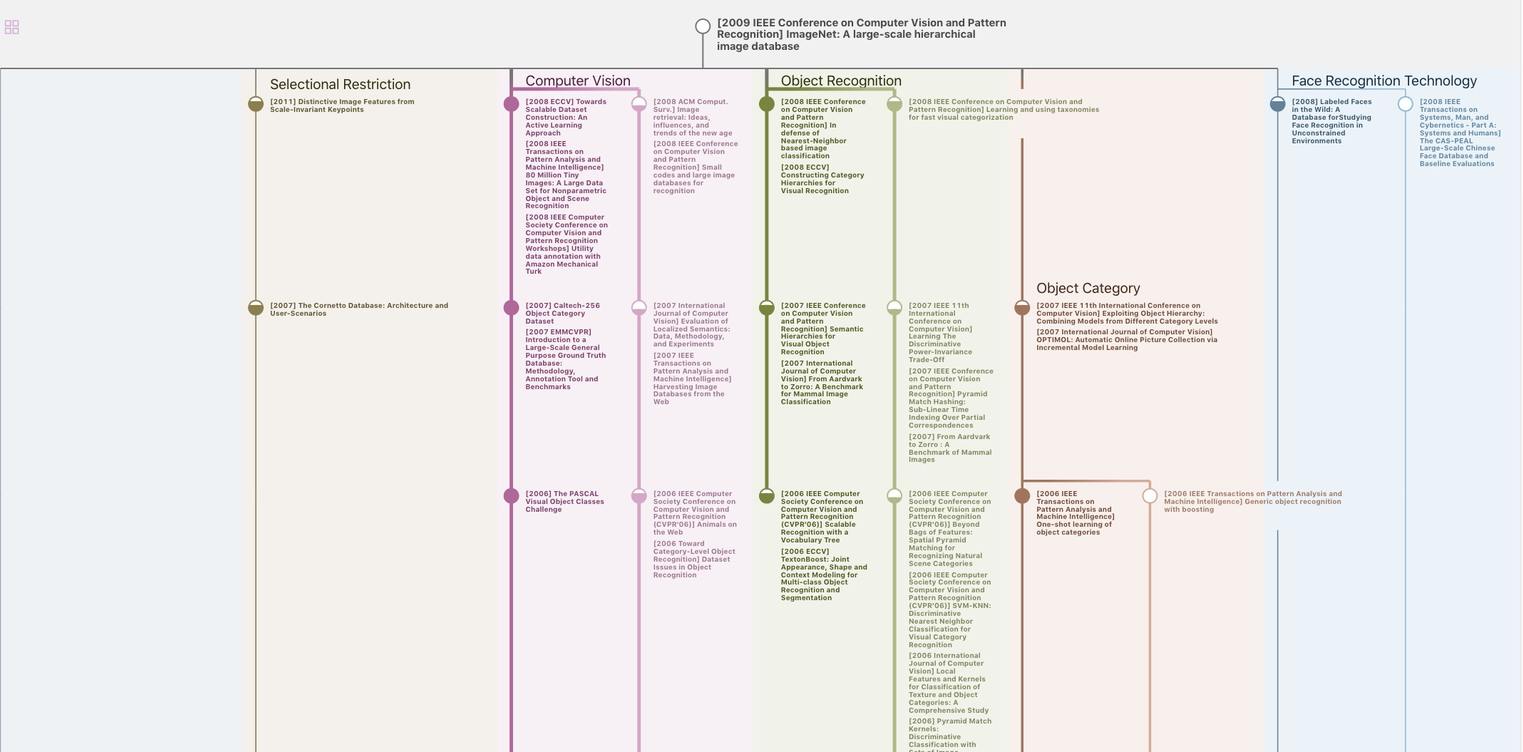
生成溯源树,研究论文发展脉络
Chat Paper
正在生成论文摘要