A GTI & Ada-Act LMCNN Method for Intelligent Fault Diagnosis of Motor Rotor-Bearing Unit Under Variable Conditions
IEEE TRANSACTIONS ON INSTRUMENTATION AND MEASUREMENT(2024)
摘要
The motor is the power source of a mechanical system, which often operates under the variable conditions make it prone to the failure, and the fault diagnosis is difficult. The classical deep learning network needs to design a deep and wide structure to enhance the feature extraction ability, but it will increase the computational cost. To realize the intelligent fault diagnosis of a motor under the variable conditions and take into account the efficiency and accuracy of the diagnosis technology, a new method based on the image data and the deep learning is proposed. Based on the ordinary gray image (GI), a feature-enhanced gray texture image (GTI) is obtained by the local binary pattern (LBP). Based on the standard convolutional neural network (CNN), a new adaptively activated lightweight multiscale CNN (Ada-act LMCNN) intelligent fault diagnosis model is developed through the improvement of the adaptive activation function, multiscale feature extraction, and model lightweight. The experimental scheme with different speeds, loads, and faults is designed for the rotor-bearing unit of a motor. The fault diagnosis effect of the proposed method is verified in the accelerated, accelerated on-load, and mixed case. The results show that the proposed method performs well among the compared methods. The model parameters are 0.2M, the floating point operations (FLOPs) are 91.8M, and the model size is 0.9 MB. The fault diagnosis accuracy under the mixed case reaches 98.5%. This work provides an intelligent, lightweight, accurate, and stable method for the motor fault diagnosis.
更多查看译文
关键词
Convolutional neural network (CNN),fault diagnosis,gray texture image (GTI),motor,rotor-bearing unit,variable conditions
AI 理解论文
溯源树
样例
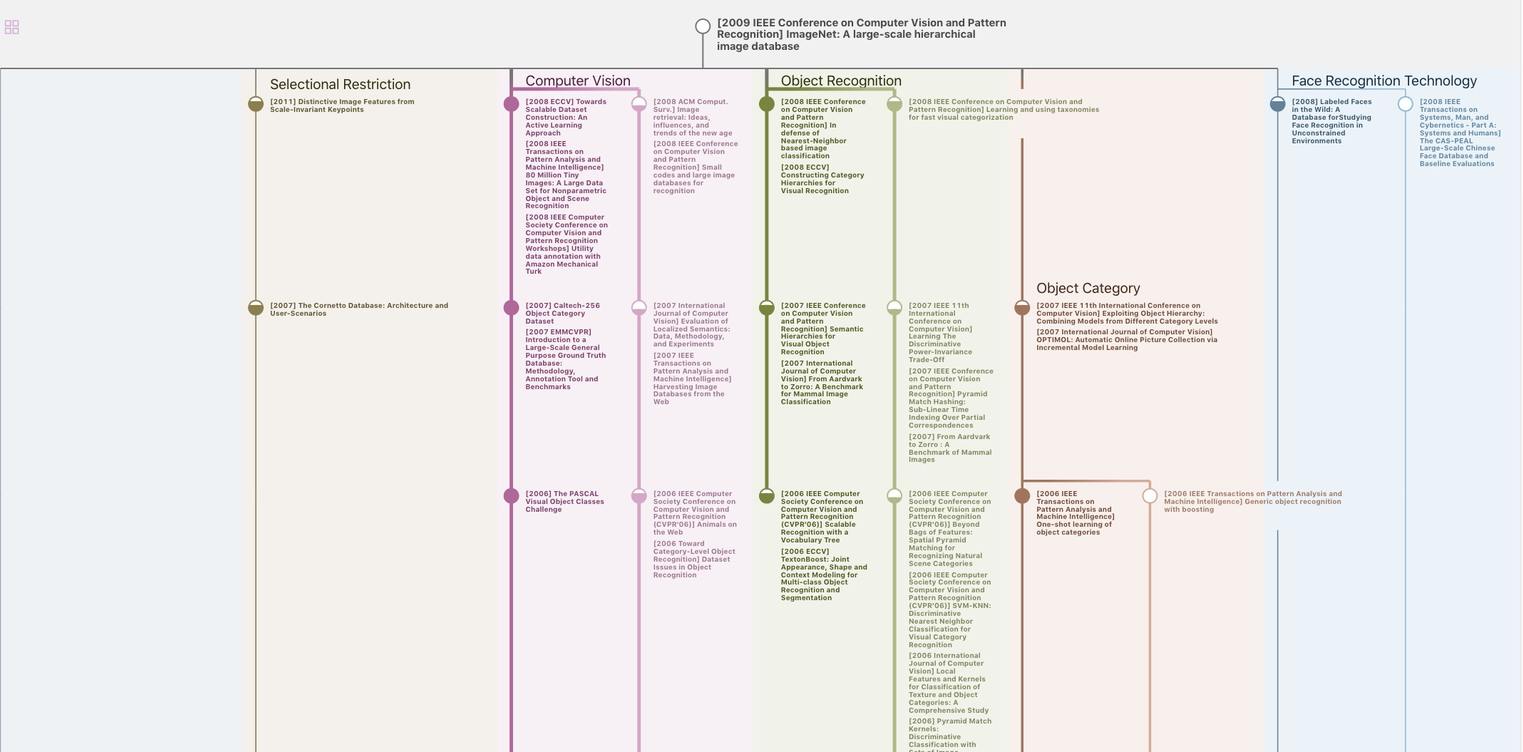
生成溯源树,研究论文发展脉络
Chat Paper
正在生成论文摘要