Exemplar-based Continual Learning via Contrastive Learning
IEEE Transactions on Artificial Intelligence(2024)
摘要
Despite the impressive performance of deep learning models, they suffer from catastrophic forgetting, which refers to a significant decline in overall performance when trained with new classes added incrementally. The primary reason for this phenomenon is the overlapping or confusion between the feature space representations of old and new classes. In this study, we examine this issue and propose a model that can mitigate the problem by learning more transferable features. We employ contrastive learning, a recent breakthrough in deep learning, which can learn visual representations better than the task-specific supervision method. Specifically, we introduce an exemplar-based continual learning method using contrastive learning to learn a task-agnostic and continuously improved feature expression. However, the class imbalance between old and new samples in continual learning can affect the final learned features. To address this issue, we propose two approaches. First, we use a novel exemplar-based method, called determinantal point processes experience replay, to improve buffer diversity during memory update. Second, we propose an old sample compensation weight to resist the corruption of the old model caused by new task learning during memory retrieval. Our experimental results on benchmark datasets demonstrate that our approach outperforms state-of-the-art methods in terms of comparable performance.
更多查看译文
关键词
Continual Learning,Incremental Learning,Self-supervised Learning,Contrastive Learning
AI 理解论文
溯源树
样例
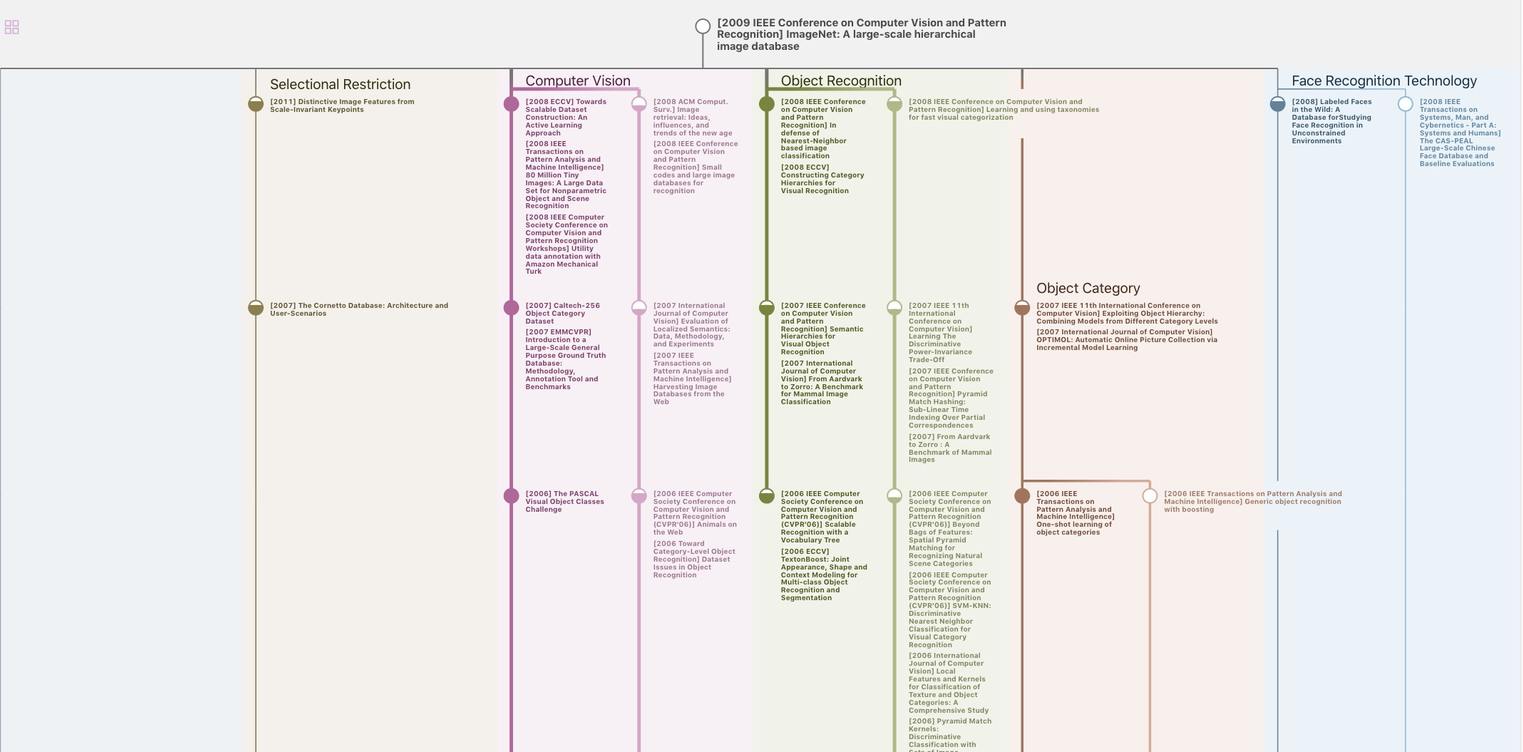
生成溯源树,研究论文发展脉络
Chat Paper
正在生成论文摘要