Efficient Structure Slimming for Spiking Neural Networks
IEEE Transactions on Artificial Intelligence(2024)
摘要
Spiking neural networks (SNNs) are deeply inspired by biological neural information systems. Compared to convolutional neural networks (CNNs), SNNs are low power consumption because of their spike based information processing mechanism. However, most of the current structures of SNNs are fully-connected or converted from deep CNNs which poses redundancy connections. While the structure and topology in human brain systems are sparse and efficient. This paper aims at taking full advantage of sparse structure and low power consumption which lie in human brain and proposed efficient structure slimming methods. Inspired by the development of biological neural network structures, this paper designed types of structure slimming methods including neuron pruning and channel pruning. In addition to pruning, this paper also considers the growth and development of the nervous system. Through iterative application of the proposed neural pruning and rewiring algorithms, experimental evaluations on CIFAR-10, CIFAR-100, and DVS-Gesture datasets demonstrate the effectiveness of the structure slimming methods. When the parameter count is reduced to only about 10% of the original, the performance decreases by less than 1%.
更多查看译文
关键词
Channel pruning,network slimming,spiking neural networks,structure learning,weight pruning
AI 理解论文
溯源树
样例
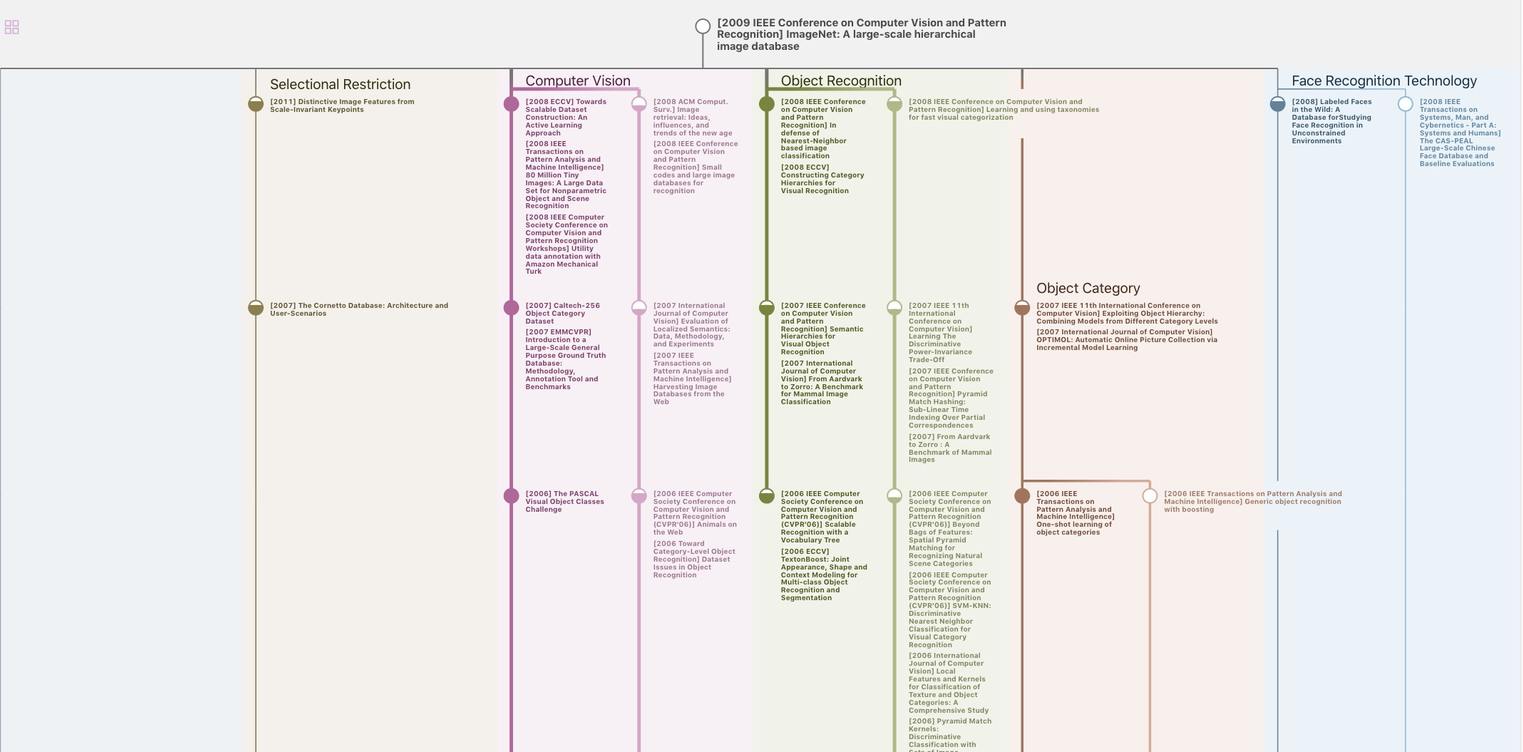
生成溯源树,研究论文发展脉络
Chat Paper
正在生成论文摘要