Radial Undersampled MRI Reconstruction Using Deep Learning with Mutual Constraints between Real and Imaginary Components of K-Space.
IEEE Journal of Biomedical and Health Informatics(2024)
摘要
The deep learning method is an efficient solution for improving the quality of undersampled magnetic resonance (MR) image reconstruction while reducing lengthy data acquisition. Most deep learning methods neglect the mutual constraints between the real and imaginary components of complex-valued k-space data. In this paper, a new complex-valued convolutional neural network (CNN), namely, Dense-U-Dense Net (DUD-Net), is proposed to interpolate the undersampled k-space data and reconstruct MR images. The proposed network comprises dense layers, U-Net, and other dense layers in sequence. The dense layers are used to simulate the mutual constraints between real and imaginary components, and U-Net performs feature sparsity and interpolation estimation for the k-space data. Two MRI datasets were used to evaluate the proposed method: brain magnitude-only MR images and knee complex-valued k-space data. Several operations were conducted to simulate the true undersampled k-space. First, the complex-valued MR images were synthesized by phase modulation on magnitude-only images. Second, a particular radial trajectory based on the golden ratio was used for k-space undersampling, whereby a reversible normalization method was proposed to balance the distribution of positive and negative values in k-space data. The optimal performance of DUD-Net was demonstrated based on a quantitative evaluation of inter-method comparisons of widely used CNNs and intra-method comparisons using an ablation study. When compared with other methods, significant improvements were achieved, PSNRs were increased by 10.78 and 5.74dB, whereas RMSEs were decreased by 71.53% and 30.31% for magnitude and phase image at least, respectively. It is concluded that DUD-Net significantly improves the performance of complex-valued k-space interpolation and MR image reconstruction.
更多查看译文
关键词
Undersampled MRI Reconstruction,K-space interpolation,Radial trajectory,Deep learning
AI 理解论文
溯源树
样例
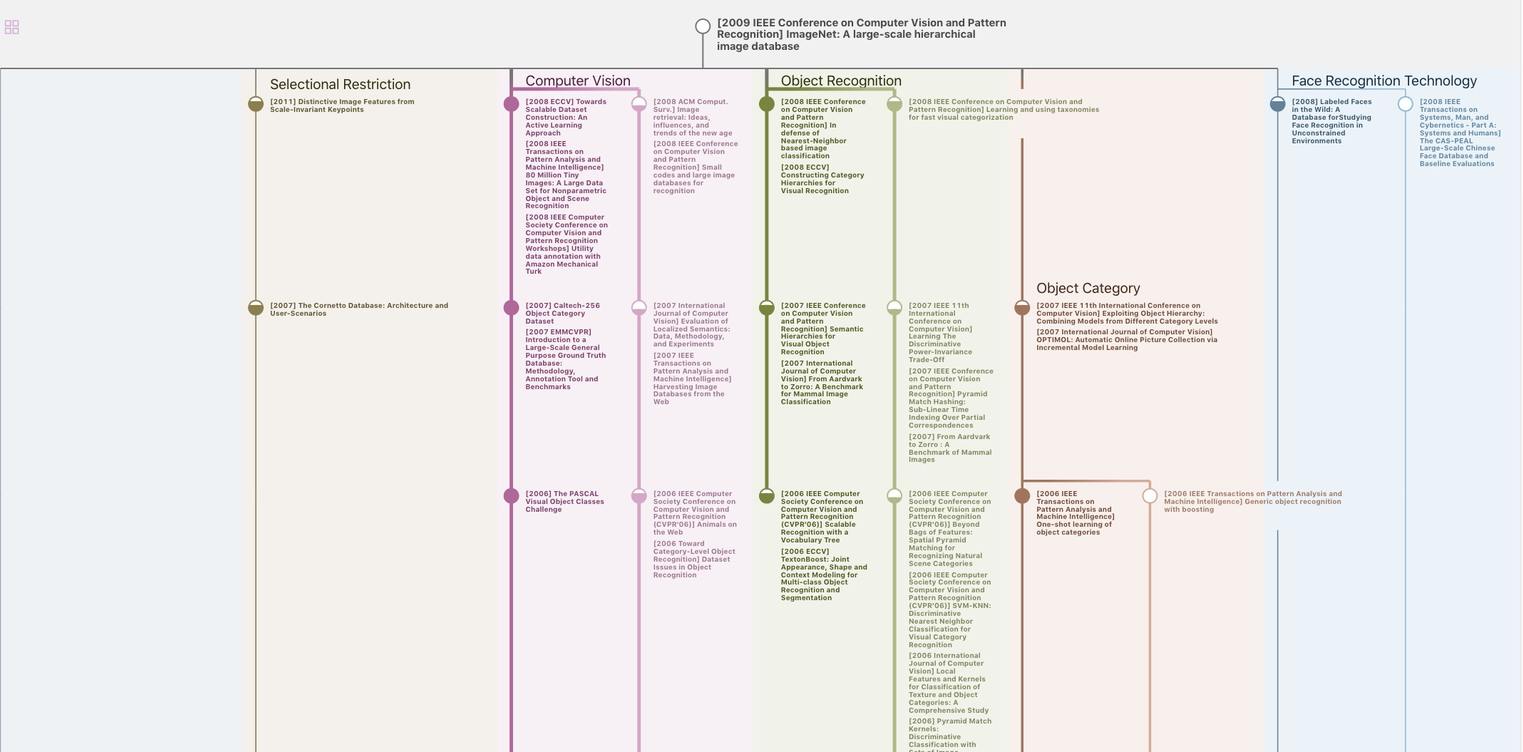
生成溯源树,研究论文发展脉络
Chat Paper
正在生成论文摘要