ViTFSL-Baseline: A Simple Baseline of Vision Transformer Network for Few-Shot Image Classification
IEEE ACCESS(2024)
摘要
Few-shot image classification, whose goal is to generalize to unseen tasks with scarce labeled data, has developed rapidly over the years. However, in traditional few-shot learning methods with CNNs, non-local features and long-rang dependencies of the image may be lost, and this leads to a poor generalization of the trained model. With the advantage of the self-attention mechanism of Transformer, researchers have tried to use vision transformer to improve few-shot learning recently. However, these methods are more complicated and take up a lot of computing resources, and there is no baseline to measure their effectiveness. We propose a new method called ViTFSL-baseline. We take advantage of vision transformer and train our model on all train set without episodic training. Meanwhile, we design a new nearest-neighbor classifier to used for few-shot image classification. Furthermore, in order to narrow the gap between difference of same class, we introduce centroid calibration in classifier after the feature extraction of backbone. We run the experiments on popular benchmarks to show that our method is a simple and effective for few-shot image classification. Our approach could be taken as the baseline upon vision transformer for few-shot learning.
更多查看译文
关键词
Deep learning,few-shot learning,feature processing,image classification,vision transformer
AI 理解论文
溯源树
样例
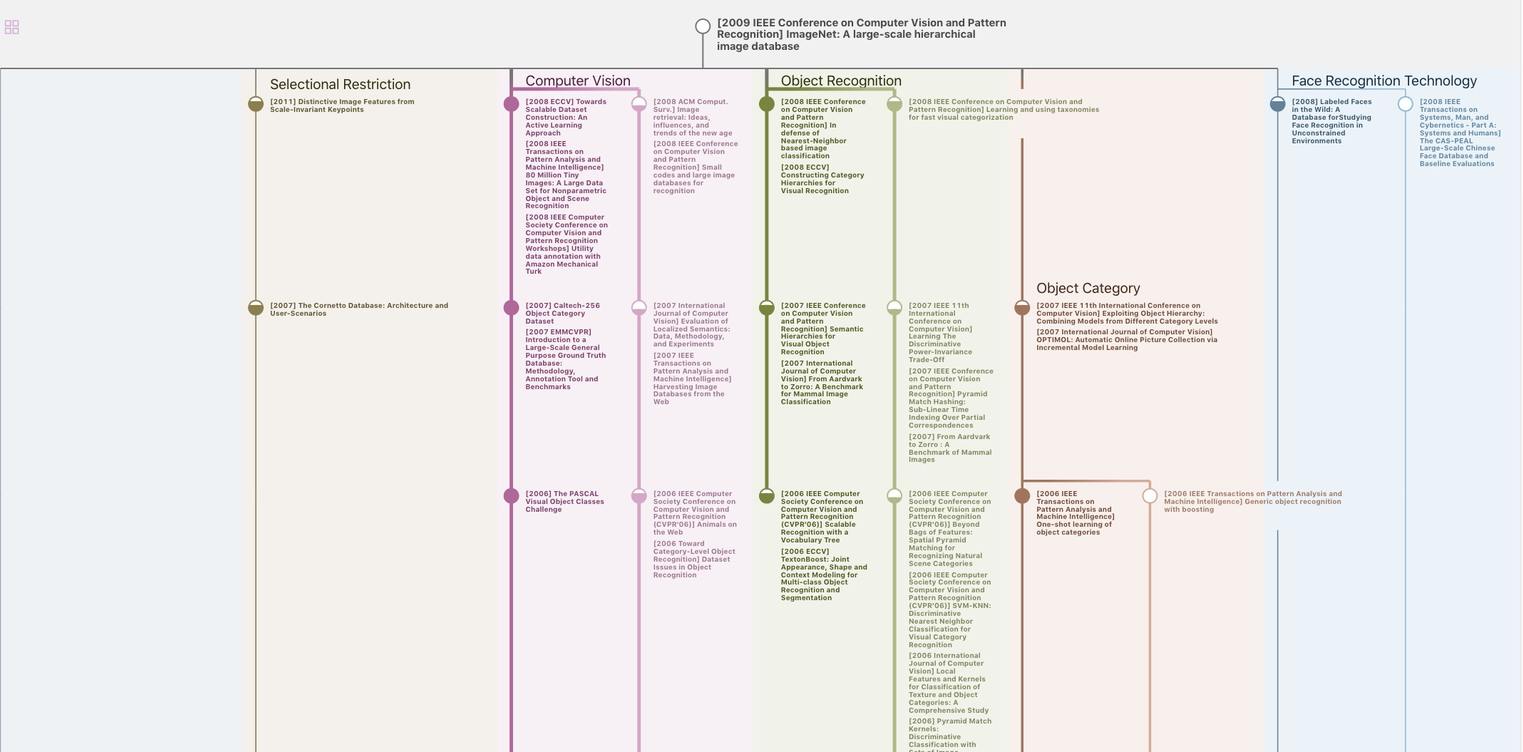
生成溯源树,研究论文发展脉络
Chat Paper
正在生成论文摘要